Quadratic Surface Preserving Parameterization of Unorganized Point Data
Computer Aided Geometric Design(2024)
摘要
Finding parameterizations of spatial point data is a fundamental step for surface reconstruction in Computer Aided Geometric Design. Especially the case of unstructured point clouds is challenging and not widely studied. In this work, we show how to parameterize a point cloud by using barycentric coordinates in the parameter domain, with the aim of reproducing the parameterizations provided by quadratic triangular Bézier surfaces. To this end, we train an artificial neural network that predicts suitable barycentric parameters for a fixed number of data points. In a subsequent step we improve the parameterization using non-linear optimization methods. We then use a number of local parameterizations to obtain a global parameterization using a new overdetermined barycentric parameterization approach. We study the behavior of our method numerically in the zero-residual case (i.e., data sampled from quadratic polynomial surfaces) and in the non-zero residual case and observe an improvement of the accuracy in comparison to standard methods. We also compare different approaches for non-linear surface fitting such as tangent distance minimization, squared distance minimization and the Levenberg Marquardt algorithm.
更多查看译文
关键词
surface approximation,neural networks,parameterization,point cloud parameterization,reverse engineering
AI 理解论文
溯源树
样例
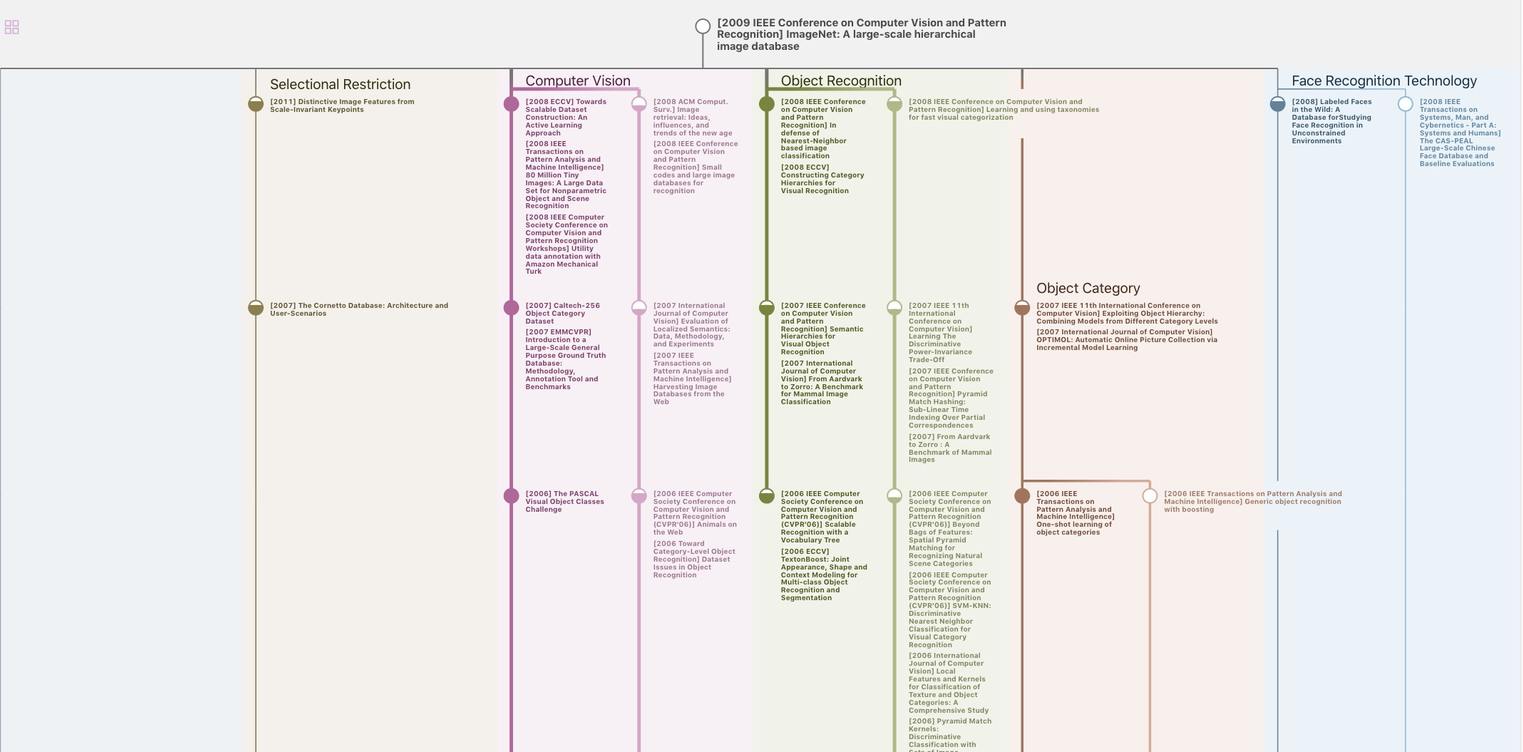
生成溯源树,研究论文发展脉络
Chat Paper
正在生成论文摘要