CA-Captioner: A novel concentrated attention for image captioning
Expert Systems with Applications(2024)
摘要
Image captioning is a task that involves understanding scenes by combining computer vision (CV) and natural language processing (NLP). While many advanced image captioning models only focus on extracting visual features for sentence generation, they neglect the importance of descriptions. To address this issue, we propose a novel concentrated attention within a fully Transformer-based image captioning model. Our approach first incorporates a positional encoding technique known as HAPE, which offers better spatial position information of objects compared with conventional positional encoding methods. Additionally, to enhance the correlation among feature pixels and direct the model’s attention toward important objects, we introduce a learnable sparse mechanism (LSM) that eliminates unnecessary noises from visual representation. Within LSM, a new RNorm function is utilized to improve the allocation of feature weights and extract emphasized object features. Furthermore, to address the limitation of self-attention in capturing local features, we employ local feature enhancement (LFE) which integrates a single layer of depth-separable convolution network to contribute to visual representation. Finally, the proposed model, named CA-Captioner, is validated on the MSCOCO, Fickr8k, and Flickr30k datasets, and the evaluation results demonstrate its robustness and effectiveness, with overall improved quantitative scores. Specifically, on the MSCOCO dataset, our model achieved a 1.4% increase in BLEU4 and a 4.0% increase in CIDEr metrics, demonstrating competitive performance compared to some advanced generators. Code is available at:https://github.com/y78h11b09/Ca-Captioner.
更多查看译文
关键词
Image captioning,Transformer,Concentrated attention,Sparse mechanism,Positional encoding
AI 理解论文
溯源树
样例
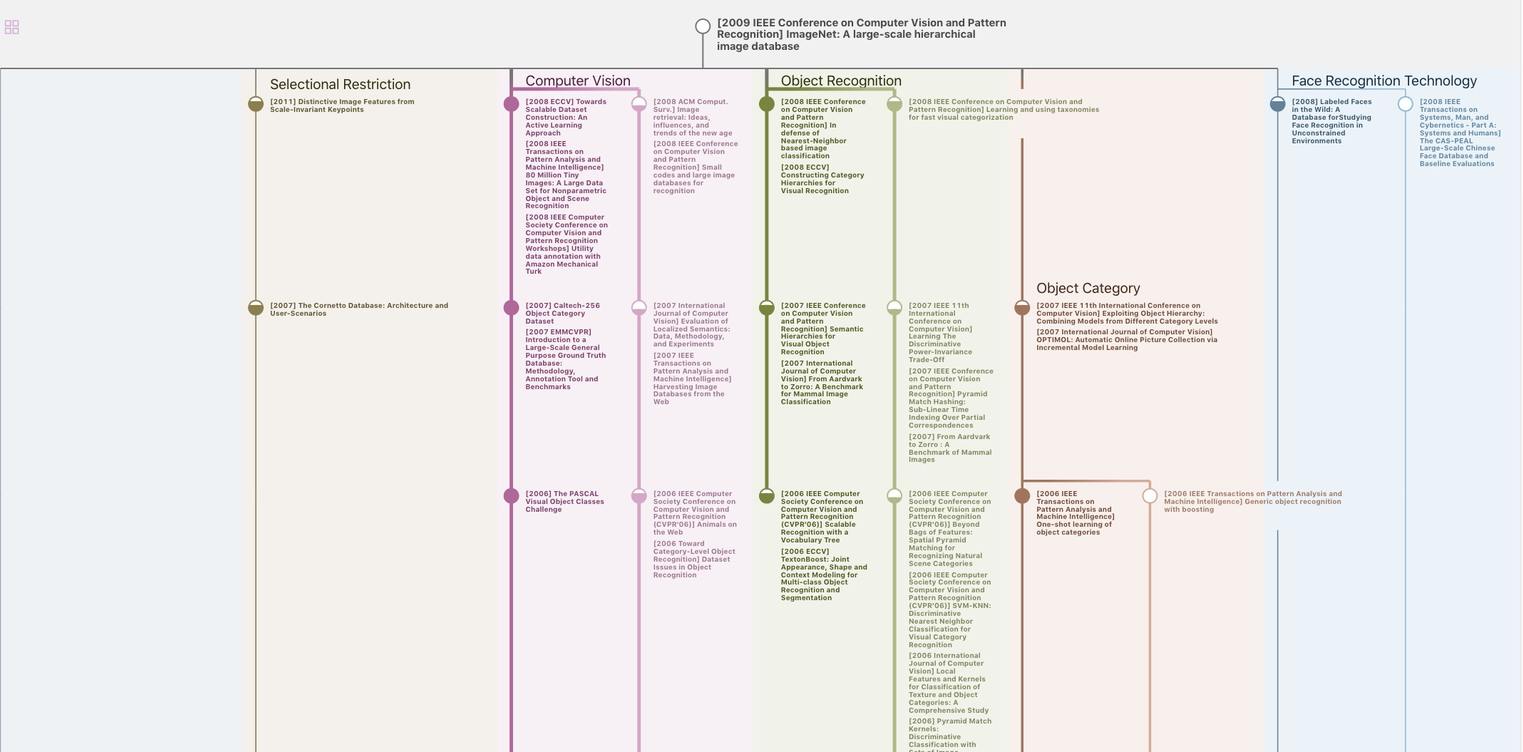
生成溯源树,研究论文发展脉络
Chat Paper
正在生成论文摘要