Ultrahigh-definition video quality assessment: A new dataset and benchmark
Neurocomputing(2024)
摘要
Video quality assessment (VQA) based on deep learning requires a massive amount of data for support. However, existing mainstream datasets do not consider the Ultrahigh-definition VQA (UHD-VQA) task, such as KoNViD1k and LIVE-Qualcomm, and can only be used for general resolution VQA tasks. These VQA datasets suffer from limitations, including low resolution and restricted scenarios. To address these issues, we present a novel UHD-VQA dataset, named UHD-VQ5k, which contains 5500 video clips, each 10 s in duration, with a resolution of 3840 × 2160 and a frame rate of 30 frames per second. Moreover, we provide strict expert ratings for each video in accordance with the ITU-R BT.500-13 standard. In addition, for the task of VQA, we propose a Hybrid Resformer Video Quality Assessment (HR-VQA) Network. The network consists of two branches, IQA and VQA, to take both video frames and video segments as inputs. In the IQA branch, features are extracted using a Resformer architecture, which including two parallel components: ResNet50 and ViT (Vision Transformer). These two components are connected through the Bidirectional Local Global Interaction module. And in the VQA branch, video segment quality is evaluated by extracting features with Swin-3D (Video Swin Transformer). The scores from both branches are individually regressed and combined through ensemble learning to obtain the ultimate video quality score. Furthermore, we introduce a “Usability Rate (UR)” metric that further enhances the accuracy of individual video predictions. Through experimental validation, our algorithm not only achieves state-of-the-art (SOTA) performance on the UHD-VQ5k dataset but also demonstrates promising results on the KONVID1k dataset and the preliminary dataset of the NAIC2023 Challenge.
更多查看译文
关键词
VQA,Dataset,Resformer,Video swin transformer,UHD
AI 理解论文
溯源树
样例
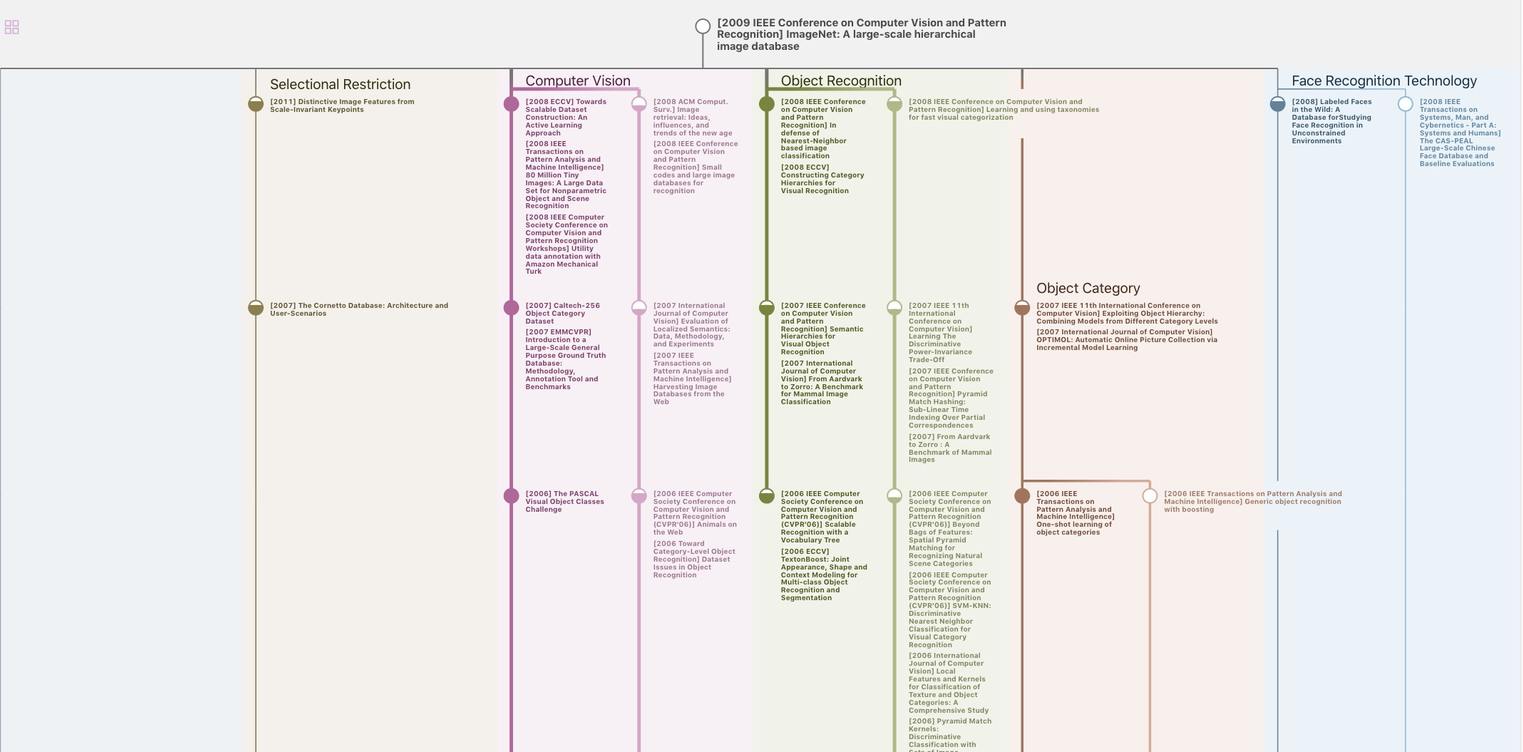
生成溯源树,研究论文发展脉络
Chat Paper
正在生成论文摘要