Enhancing user prompt confidentiality in Large Language Models through advanced differential encryption
Computers and Electrical Engineering(2024)
摘要
In the era of artificial intelligence (AI) advancements heralded by Large Language Models (LLMs) like GPT-3, the capacity to parse and generate human-like text brings to light substantial privacy concerns. These arise notably from LLMs’ reliance on vast datasets often laden with personal information, underscoring the potential for inadvertent memorization and disclosure of sensitive data. Addressing these pivotal privacy concerns, our research introduces a novel two-fold approach aimed at bolstering the confidentiality and security of user data in LLM applications. Firstly, we deploy advanced cryptographic techniques, incorporating bespoke encryption and hashing protocols, to preprocess user data. This strategy effectively anonymizes personal identifiers prior to their processing by LLMs, directly tackling the challenges of sensitive information exposure. Concurrently, our methodology encompasses a secure mutual authentication protocol utilizing lightweight cryptographic measures. This ensures that system interactions are strictly reserved for authenticated users, thereby enhancing overall data security. Collectively, our approach not only preserves the utility of data for AI tasks but also fortifies the privacy framework surrounding LLMs, significantly reducing the likelihood of privacy breaches and steering AI development towards a more secure and ethically grounded future.
更多查看译文
关键词
Cryptographic privacy,Large Language Models,Data anonymization,Secure AI framework,Personal data protection
AI 理解论文
溯源树
样例
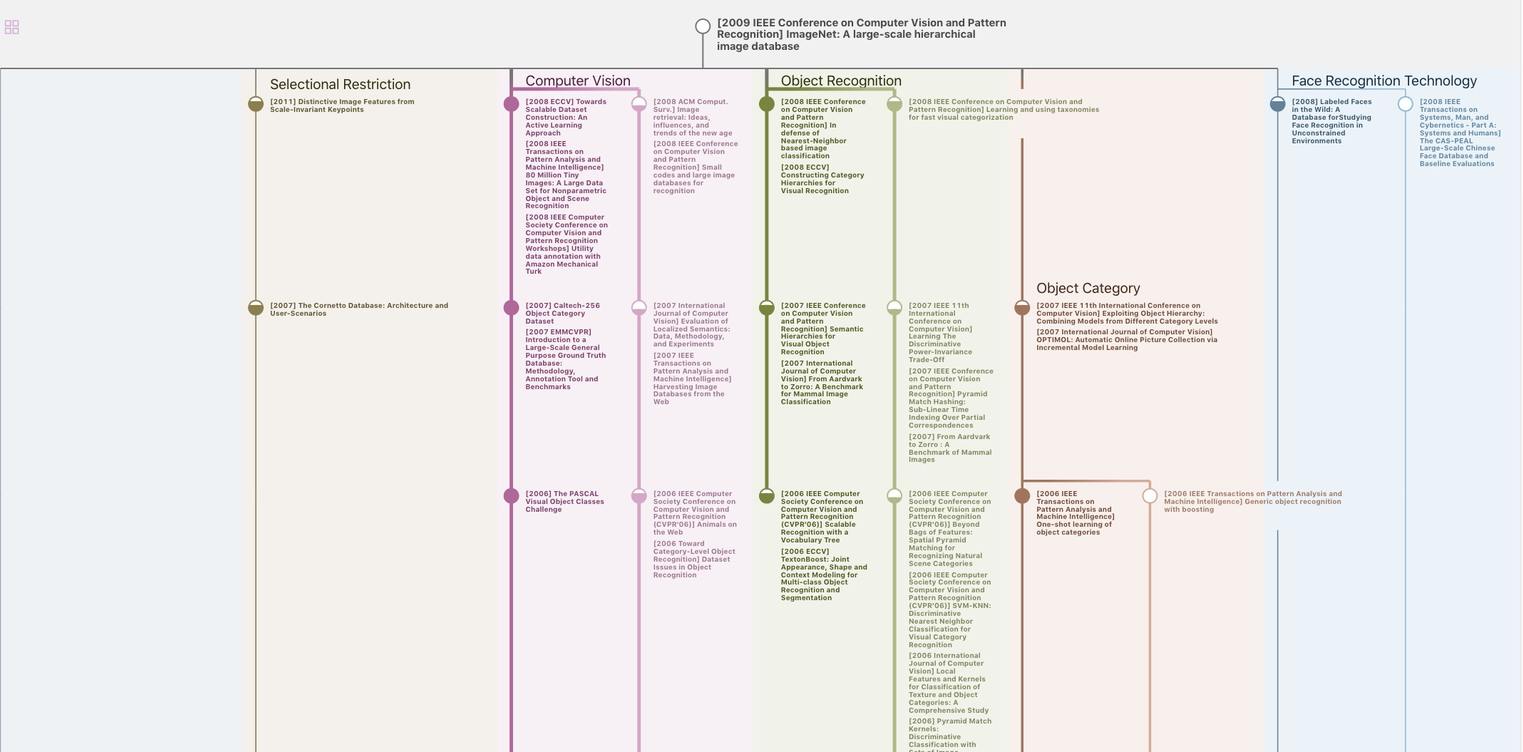
生成溯源树,研究论文发展脉络
Chat Paper
正在生成论文摘要