Few-shot Classification of Wafer Bin Maps Using Transfer Learning and Ensemble Learning
Journal of Manufacturing Science and Engineering(2024)
摘要
Abstract The high cost of collecting and annotating wafer bin maps (WBMs) necessitates few-shot WBM classification, i.e., classifying WBM defect patterns using a limited number of WBMs. Existing few-shot WBM classification algorithms mainly utilize meta learning methods that leverage knowledge learned in several episodes. However, meta-learning methods require a large amount of additional real WBMs, which can be unrealistic. To help train a network with a few real WBMs while avoiding this challenge, we propose the use of simulated WBMs to pre-train a classification model. Specifically, we employ transfer learning by pre-training a classification network with sufficient amounts of simulated WBMs and then fine-tuning it with a few real WBMs. We further employ ensemble learning to overcome the overfitting problem in transfer learning by fine-tuning multiple sets of classification layers of the network. A series of experiments on a real dataset demonstrate that our model outperforms the meta-learning methods that are widely used in few-shot WBM classification. Additionally, we empirically verify that transfer and ensemble learning, the two most important yet simple components of our model, reduce the prediction bias and variance in few-shot scenarios without a significant increase in training time.
更多查看译文
AI 理解论文
溯源树
样例
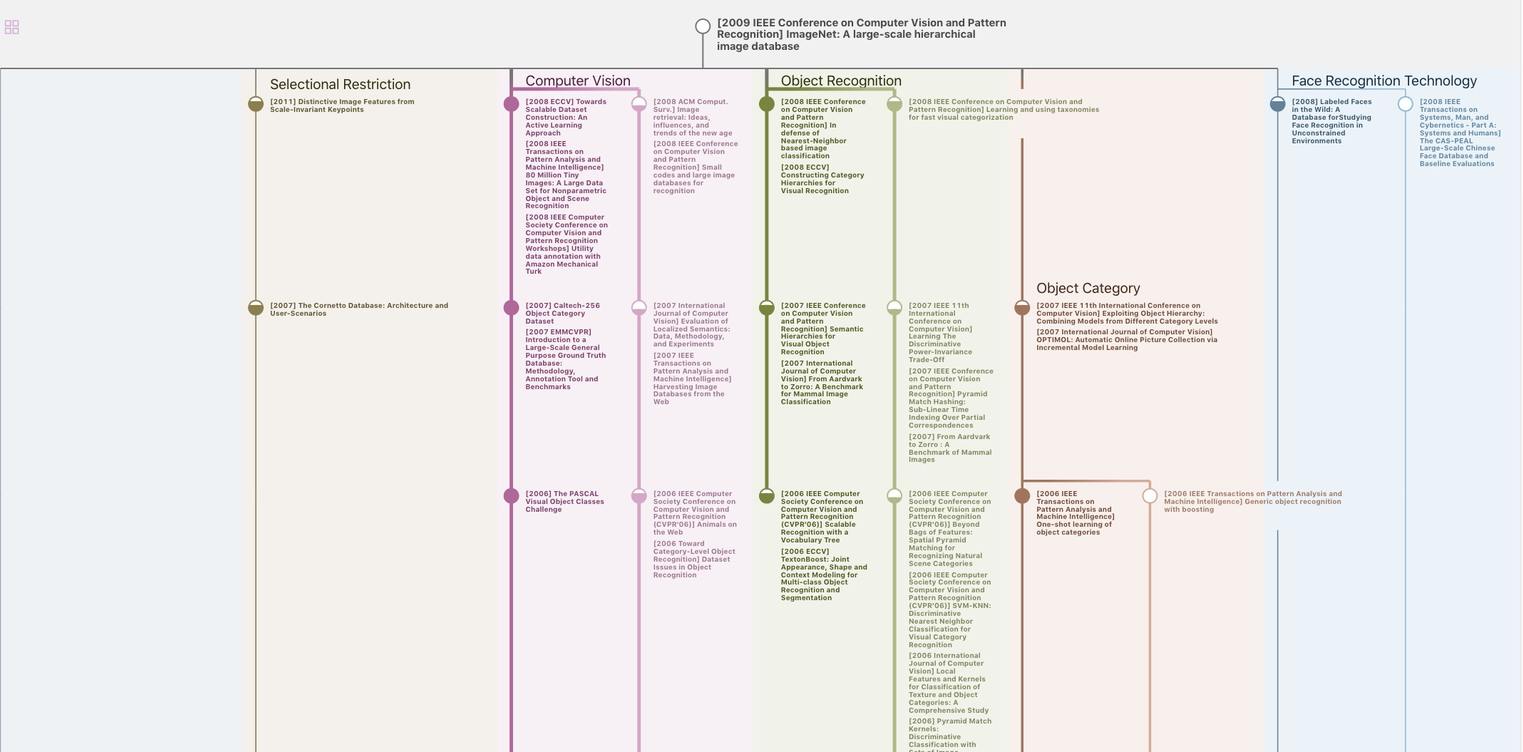
生成溯源树,研究论文发展脉络
Chat Paper
正在生成论文摘要