MosquitoNet Based Deep Learning Approach for Malaria Parasite Detection Using Cell Images
2024 International Conference on Automation and Computation (AUTOCOM)(2024)
摘要
Malaria is a major global health issue made worse by the COVID-19 pandemic. This paper explores using Convolutional Neural Networks (CNNs) to accurately detect malaria by analysing microscopic blood smear images. This work uses a diverse and balanced dataset of infected and uninfected malaria cell images, which is augmented to improve dataset diversity and generalisation. In this work, the MosquitoNet model, which includes convolutional and fully connected layers, is trained using the Adam optimiser with the binary cross entropy loss. The results show that the model has a high accuracy of 96.97% on the test set, effectively distinguishing between malaria-infected and uninfected cells. Recall, Precision, and F1-score metrics are used to evaluate MosquitoNet's efficiency. This work contributes to deep learning in healthcare and highlights the importance of advanced technologies in fighting life-threatening diseases like malaria. Continued progress and research in this area can potentially improve medical imaging techniques and healthcare outcomes.
更多查看译文
AI 理解论文
溯源树
样例
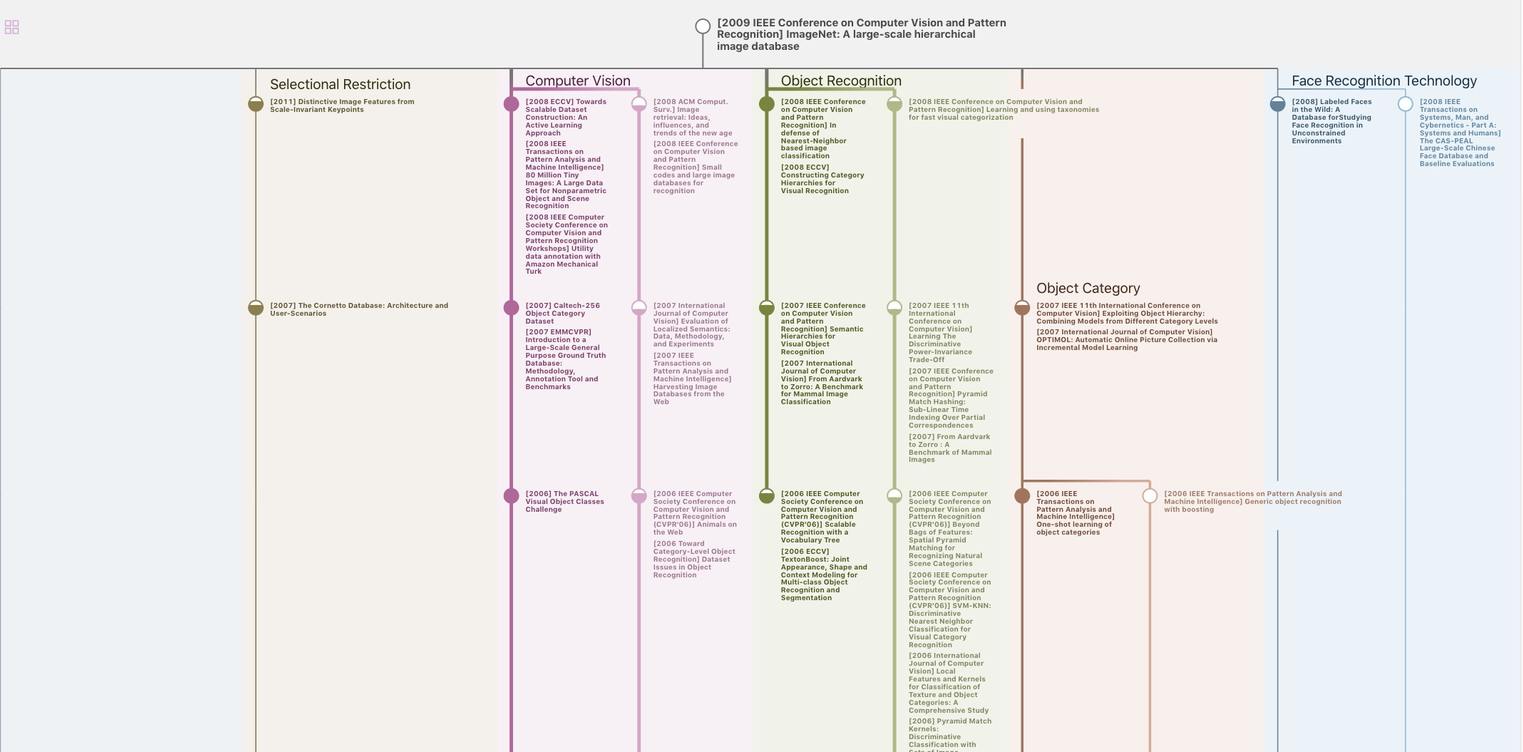
生成溯源树,研究论文发展脉络
Chat Paper
正在生成论文摘要