MEDITRON: Open Medical Foundation Models Adapted for Clinical Practice
crossref(2024)
摘要
Abstract Large language and multimodal models (LLMs and LMMs) will transform access to medical knowledge and clinical decision support. However, the current leading systems fall short of this promise, as they are either limited in scale, which restricts their capabilities, closed-source, which limits the extensions and scrutiny that can be applied to them, or not sufficiently adapted to clinical settings, which inhibits their practical use. In this work, we democratize large-scale medical AI systems by developing MEDITRON: a suite of open-source LLMs and LMMs with 7B and 70B parameters adapted to the medical domain. MEDITRON extends pretraining on a comprehensively curated medical corpus that includes biomedical literature and internationally recognized clinical practice guidelines. Evaluations using standard medical reasoning benchmarks show significant improvements over all current open-access models and several state-of-the-art commercial LLMs that are orders of magnitude larger, more expensive to host, and closed-source. Enhanced with visual processing capabilities, our MEDITRON-V model also outperforms all open-access models and much larger closed-source models on multimodal reasoning tasks for various biomedical imaging modalities. Beyond traditional benchmarks, we also create a novel and physician-driven adversarial question dataset grounded in real-world clinical settings, and a comprehensive 17-metric evaluation rubric to assess alignment and contextualization to real-world clinical practice. Applying this framework to MEDITRON-70B's responses, sixteen independent physicians found a high level of alignment across all metrics, including medical accuracy, safety, fairness, communication, and interpretation. The MEDITRON suite is a significant step forward in closing the technological gap between closed- and open-source medical foundation models. By releasing our methodologies, models, and real-world clinical practice benchmarks, we aim to drive the open-source development of more capable, representative, accessible, and transparent medical AI assistants.
更多查看译文
AI 理解论文
溯源树
样例
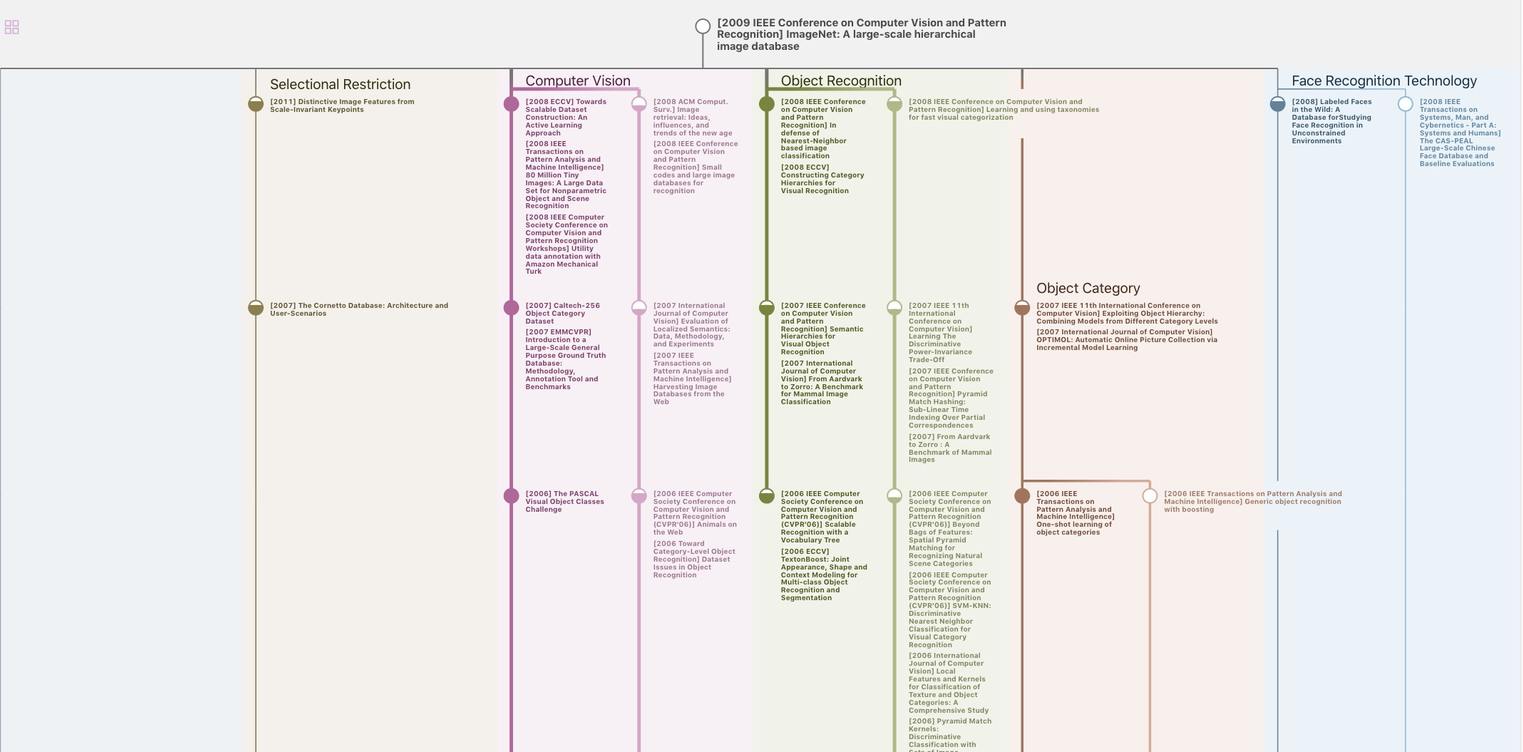
生成溯源树,研究论文发展脉络
Chat Paper
正在生成论文摘要