Comparison of Hybrid LSTAR-GARCH Model with Conventional Stochastic and Artificial-Intelligence Models to Estimate Monthly Streamflow
Water resources management(2024)
Abstract
Streamflow estimation plays an important role in water resources planning and management, and minimizes the impact of floods and droughts. Therefore, this study aims to estimate monthly streamflows in a semi-arid region of India by employing multiple data-driven models, which include standalone time-series models such as Thomas-Fiering (T-F), autoregressive moving average (ARMA), and the logistic smooth threshold autoregressive (LSTAR) models, artificial intelligence (AI) technique such as artificial neural network (ANN), and an advanced novel hybrid model such as LSTAR coupled with generalized autoregressive conditional heteroscedasticity (GARCH), i.e., LSTAR-GARCH model. Furthermore, capability of five models including standalone models (T-F, ARMA), a cascade feed-forward back propagation neural network (CFBPNN), LSTAR, and the hybrid LSTAR-GARCH model, was comparatively evaluated in estimating 40-year (June 1975 to May 2015) monthly streamflow of Jakham reservoir located in Rajasthan state of India. The models were calibrated using first 75% of the dataset, and their predictions were evaluated using four performance evaluation criteria: correlation coefficient (R), root mean square error (RMSE), modified Nash-Sutcliffe efficiency (MNSE), and modified index of agreement (MIA). The developed models were validated using remaining 25% dataset (120 months), and their performances were compared by plotting hydrographs and scatter plots between the observed and estimated values. The results suggested that the T-F model was satisfactory for generating synthetic monthly streamflow while the ARMA model accurately estimated the streamflows except at extremes. Among the stand-alone models, CFBPNN-4 model outperformed the other three ones with a higher MNSE value of 0.53, and a lower RMSE value of 30.78 million m3. The proposed hybrid LSTAR-GARCH model, which is based on a novel framework, outperformed the standalone ones in terms of R, MNSE, MIA, and RMSE (0.89, 0.78, 0.58, and 25.92 million m3, respectively).
MoreTranslated text
Key words
Stochastic models,Artificial intelligence,Thomas-Fiering model,LSTAR-GARCH model
AI Read Science
Must-Reading Tree
Example
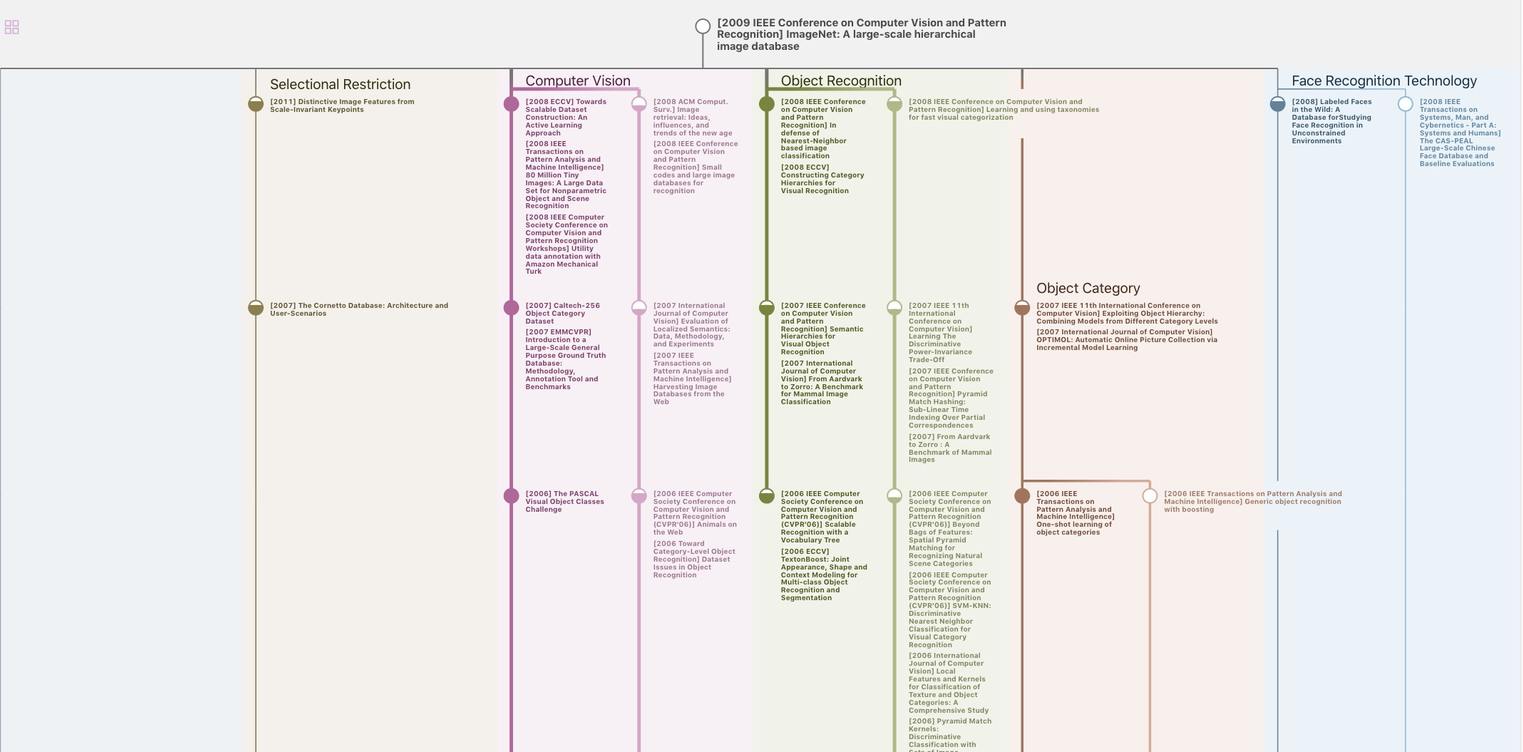
Generate MRT to find the research sequence of this paper
Chat Paper
Summary is being generated by the instructions you defined