Self-paced learning long short-term memory based on intelligent optimization for robust wind power prediction
IET CONTROL THEORY AND APPLICATIONS(2024)
摘要
Given the unpredictable and intermittent nature of wind energy, precise forecasting of wind power is crucial for ensuring the safe and stable operation of power systems. To reduce the influence of noise data on the robustness of wind power prediction, a wind power prediction method is proposed that leverages an enhanced multi-objective sand cat swarm algorithm (MO-SCSO) and a self-paced long short-term memory network (spLSTM). First, the actual wind power data is processed into time series as input and output. Then, the progressive advantage of self-paced learning is used to effectively solve the instability caused by noisy data during long short-term memory network (LSTM) training. Following this, the improved MO-SCSO is employed to iteratively optimize the hyperparameters of spLSTM. Ultimately, a combined MO-SCSO-spLSTM model is constructed for wind power prediction. This model is validated with the data of onshore wind farms in Austria and offshore wind farms in Denmark. The experimental results show that compared with the traditional LSTM prediction method, the proposed method has better prediction accuracy and robustness. Specifically, in the onshore and offshore wind power prediction experiments, the proposed method reduces the minimum MAE by 5.44% and 4.96%, respectively, and reduces the MAE range by 4.45% and 17.21%, respectively, which could be conducive to the safe and stable operation of power system. To reduce the influence of noise data on the robustness of wind power prediction, the authors propose a wind power prediction method that leverages an enhanced multi-objective sand cat swarm algorithm and a self-paced long short-term memory network. image
更多查看译文
关键词
wind power,wind turbines
AI 理解论文
溯源树
样例
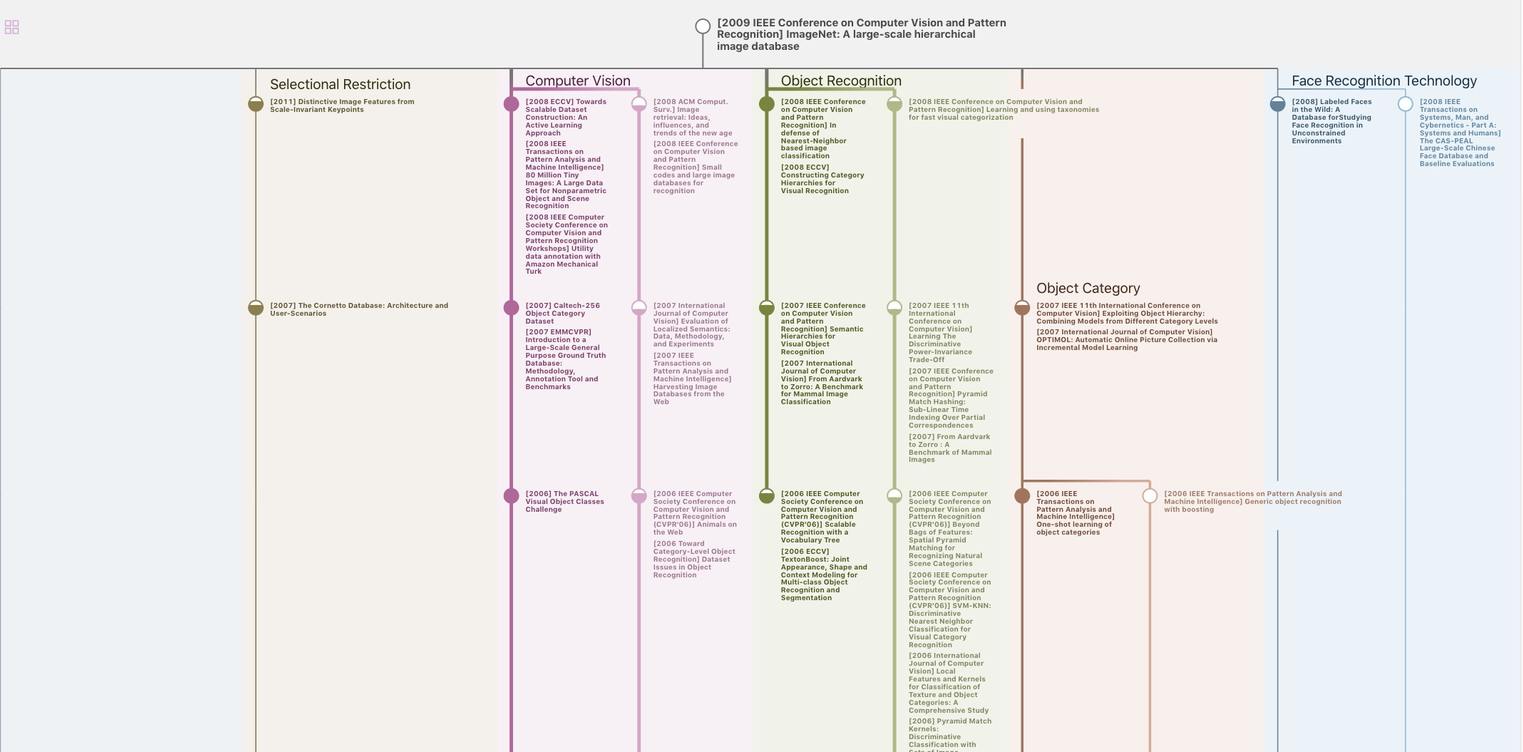
生成溯源树,研究论文发展脉络
Chat Paper
正在生成论文摘要