Spatiotemporal audio feature extraction with dynamic memristor-based time-surface neurons
SCIENCE ADVANCES(2024)
摘要
Neuromorphic speech recognition systems that use spiking neural networks (SNNs) and memristors are progressing in hardware development. The conventional manual preprocessing of audio signals is shifting toward event-based recognition with convolutional SNNs. Despite achieving high accuracy in classification, the efficient extraction of spatiotemporal features from audio events continues to be a substantial challenge. In this study, we introduce dynamic time-surface neurons (DTSNs) using volatile memristors featuring an adjustable temporal kernel decay, enabled by series-connected transistors with an Au/LiCoO2/Au configuration. DTSNs act as feature descriptors, enhancing the spatiotemporal feature extraction from event audio data. A two-layer SNN classifier, fully connected and incorporating a 1T1R nonvolatile memristor array, is trained to recognize the spatiotemporal features of the audio data. Our findings show classification accuracies of up to 95.91%, substantial improvements in computational efficiency, and increased noise resilience, confirming the promise of our memristor-based speech recognition system for practical applications.
更多查看译文
AI 理解论文
溯源树
样例
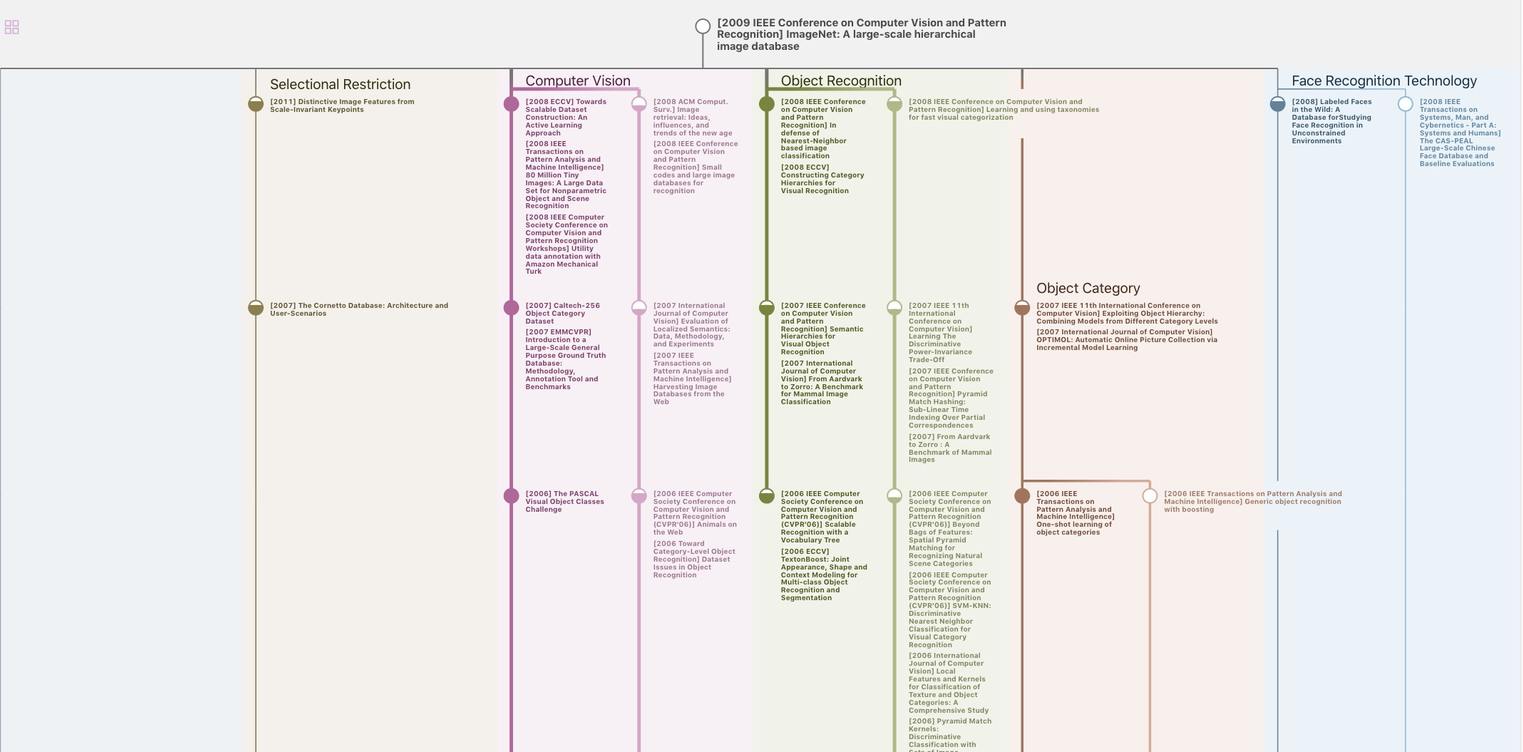
生成溯源树,研究论文发展脉络
Chat Paper
正在生成论文摘要