Intelligent prediction method for underbreak extent in underground tunnelling
International Journal of Rock Mechanics and Mining Sciences(2024)
摘要
Underground tunnel excavation using the drill-and-blast method often results in underbreak occurrences due to improper blasting parameters and complex geological environment. This underbreak phenomenon has a profound impact on tunnel safety, stability and construction costs. Traditional approaches for predicting underbreak extent (UE) through field measurements and theoretical models exhibit limitations in terms of accuracy and efficiency. Consequently, it is urgent to develop a novel approach for UE prediction in tunnelling operations. In this paper, extreme gradient boosting (XGBoost) is first applied as the foundational algorithm, and slime mould algorithm (SMA) is implemented for tuning hyper-parameters of XGBoost. Meanwhile, six chaotic maps are integrated with SMA to initialize the population and improve the optimization performance. Subsequently, a dataset of 250 samples is collected from three underground mines in China, involving ten influential factors (RMR, uniaxial compressive strength (UCS), vertical principal stress (σv), lateral pressure coefficient (λ), excavation area (EA), powder factor (PF), specific charge (SC), advance length (AL), periphery hole burden (HB)and periphery hole spacing (HS)). Additionally, four indices, i.e., coefficient of determination (R2), variance accounted for (VAF), mean absolute error (MAE) and root mean square error (RMSE), are used to evaluate the comprehensive performance of these proposed COSMA-XGBoost models and four common machine learning models. Finally, a parametric sensitivity analysis is performed, and the optimal intelligent model is applied in practical tunnelling projects. The results indicate that chaotic optimized SMA models have better convergence ability and higher accuracy compared with the original SMA model. Notably, the PSMA-XGBoost model outperforms other models as the R2, VAF, MAE and RMSE values for the testing set are 0.979, 95.79%, 4.176 and 4.884, respectively, whereas 0.968, 94.25%, 4.274 and 5.012, respectively for training set. EA, RMR and UCS exhibit significant effects on UE. In practical tunneling projects, the engineering application of the PSMA-XGBoost model demonstrates its feasibility and efficiency in predicting UE within underground tunnels.
更多查看译文
关键词
Underbreak extent,Blasting excavation,Underground tunnelling,COSMA,XGBoost
AI 理解论文
溯源树
样例
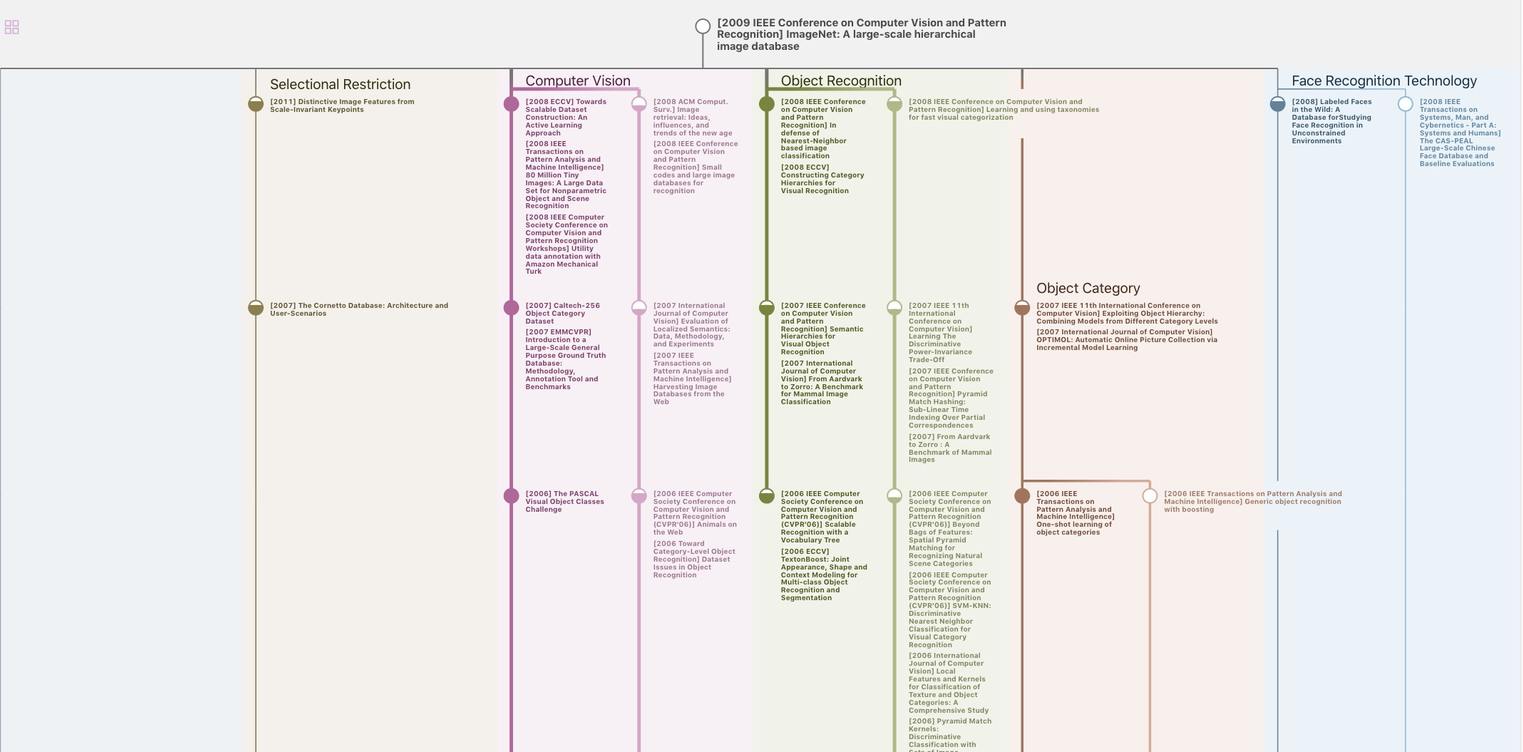
生成溯源树,研究论文发展脉络
Chat Paper
正在生成论文摘要