Data harvesting vs data farming: A study of the importance of variation vs sample size in deep learning-based auto-segmentation for breast cancer patients
arxiv(2024)
摘要
The aim of this study was to investigate the difference in output, when
training a model in three different scenarios: a large clinical delineated data
set (with 700/78 patients for training/testing, from the Danish Breast Cancer
Group (DBCG) RT Nation Study), a clinical but curated dataset (with 328/36
patients for training/testing, from the DBCG RT Nation Study) and a smaller,
but dedicated data set created by delineation experts (with 123/14 patients for
training/testing, consensus delineations created by delineation experts). The
model performance was estimated based on the performance metrics dice
similarity coefficient (DSC), Hausdorff 95th percentile (HD95) and mean surface
distance (MSD). Models were tested in test sets from their own cohort, and
afterwards also compared in the dedicated data test set. The difference between
model output was finally estimated by measuring the mean width and cranial
caudal length of the model output for the models. When testing the model output
between the clinical models and the dedicated models in their own test set, the
two clinical models had a poorer performance, than the dedicated models, but
not all metrics showed statistically significance. When testing the models in
the dedicated data, the dedicated model showed a slightly better performance,
along with fewer segmentation outliers. As a way of taking advantage of the
strength from both types of data set, it could be an option to use a large
clinical data set as a baseline model, and then finetune with smaller sized
cohorts with dedicated delineations.
更多查看译文
AI 理解论文
溯源树
样例
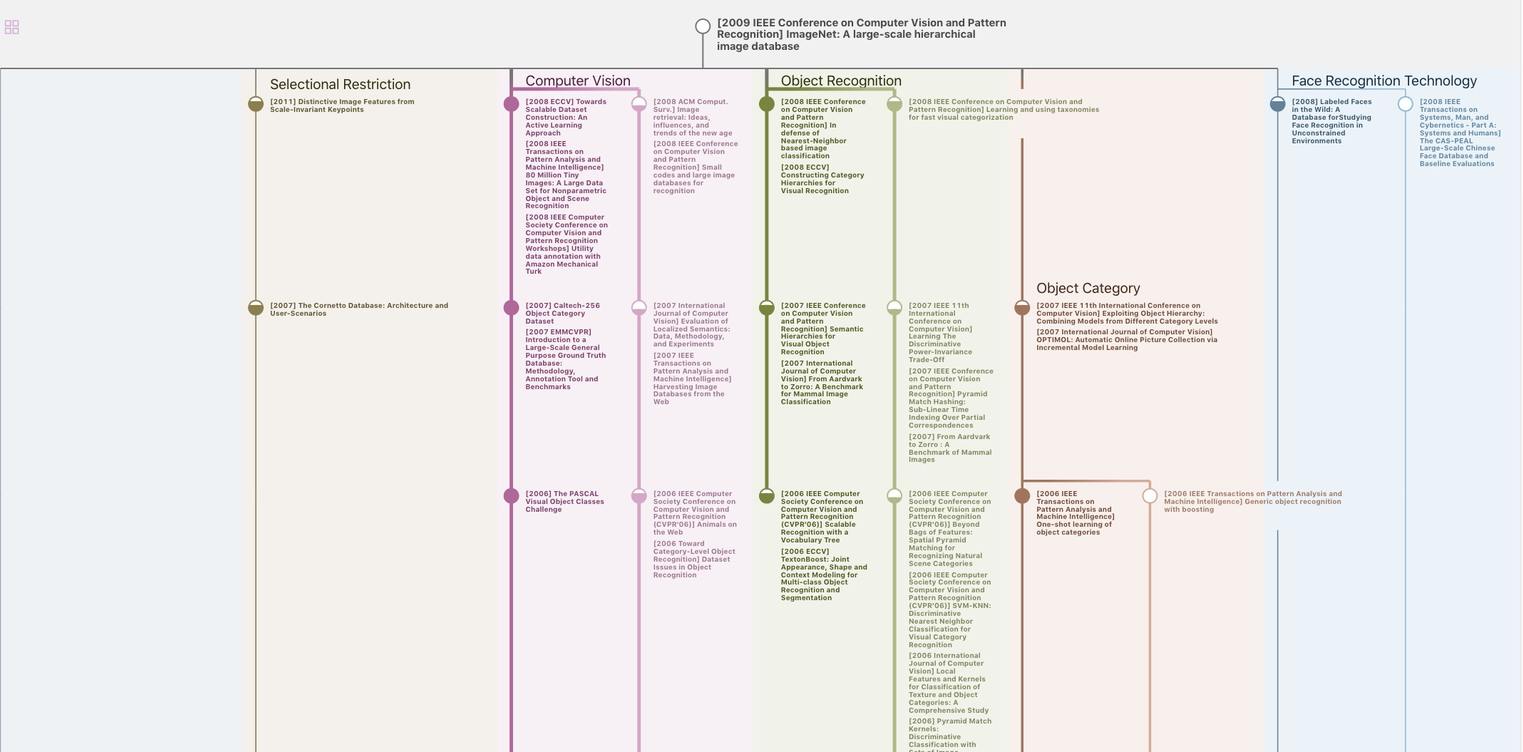
生成溯源树,研究论文发展脉络
Chat Paper
正在生成论文摘要