vPALs: Towards Verified Performance-aware Learning System For Resource Management
arxiv(2024)
摘要
Accurately predicting task performance at runtime in a cluster is
advantageous for a resource management system to determine whether a task
should be migrated due to performance degradation caused by interference. This
is beneficial for both cluster operators and service owners. However, deploying
performance prediction systems with learning methods requires sophisticated
safeguard mechanisms due to the inherent stochastic and black-box natures of
these models, such as Deep Neural Networks (DNNs). Vanilla Neural Networks
(NNs) can be vulnerable to out-of-distribution data samples that can lead to
sub-optimal decisions. To take a step towards a safe learning system in
performance prediction, We propose vPALs that leverage well-correlated system
metrics, and verification to produce safe performance prediction at runtime,
providing an extra layer of safety to integrate learning techniques to cluster
resource management systems. Our experiments show that vPALs can outperform
vanilla NNs across our benchmark workload.
更多查看译文
AI 理解论文
溯源树
样例
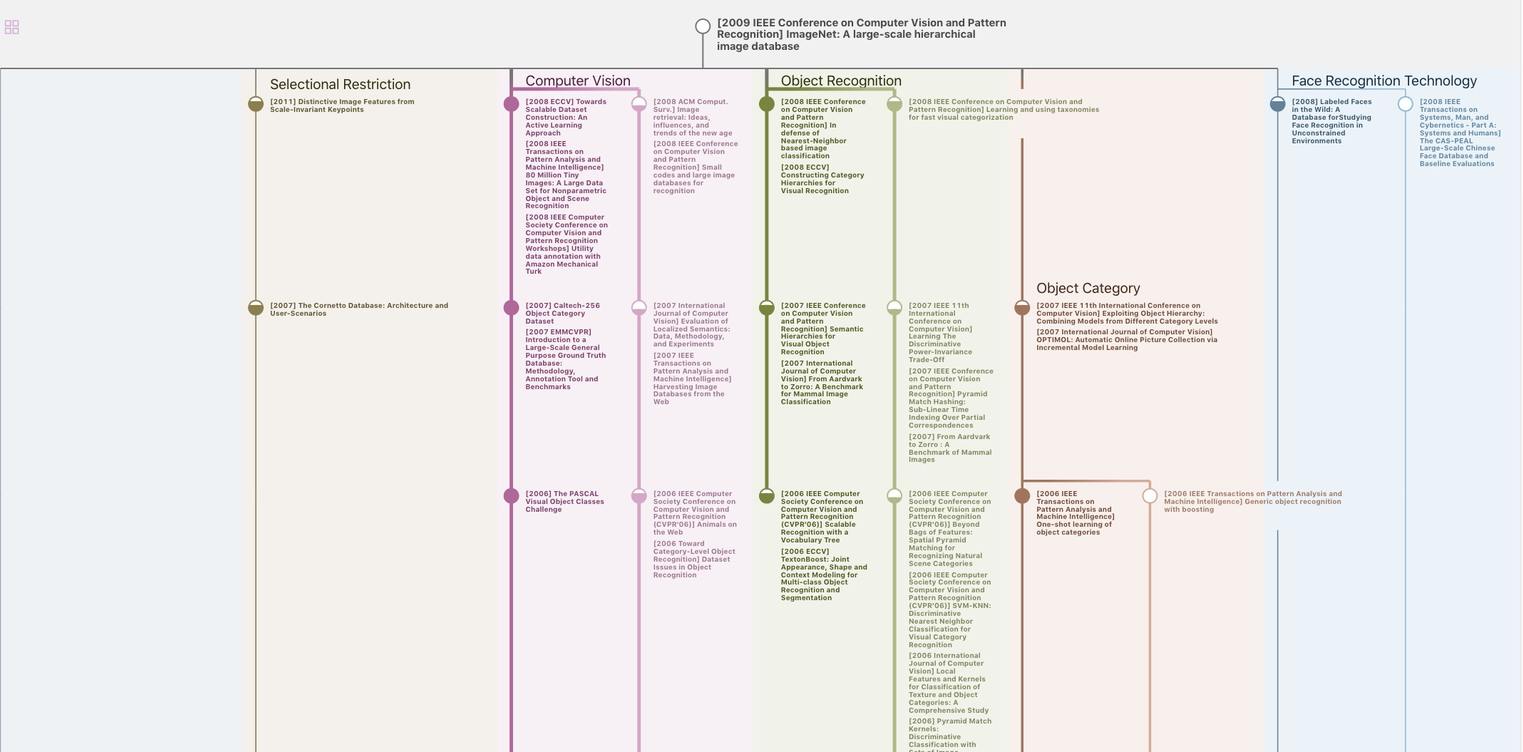
生成溯源树,研究论文发展脉络
Chat Paper
正在生成论文摘要