A Comparative Analysis of Word-Level Metric Differential Privacy: Benchmarking the Privacy-Utility Trade-off
arXiv (Cornell University)(2024)
Abstract
The application of Differential Privacy to Natural Language Processingtechniques has emerged in relevance in recent years, with an increasing numberof studies published in established NLP outlets. In particular, the adaptationof Differential Privacy for use in NLP tasks has first focused on theword-level, where calibrated noise is added to word embeddingvectors to achieve "noisy" representations. To this end, severalimplementations have appeared in the literature, each presenting an alternativemethod of achieving word-level Differential Privacy. Although each of theseincludes its own evaluation, no comparative analysis has been performed toinvestigate the performance of such methods relative to each other. In thiswork, we conduct such an analysis, comparing seven different algorithms on twoNLP tasks with varying hyperparameters, including the epsilon(ε) parameter, or privacy budget. In addition, we provide anin-depth analysis of the results with a focus on the privacy-utility trade-off,as well as open-source our implementation code for further reproduction. As aresult of our analysis, we give insight into the benefits and challenges ofword-level Differential Privacy, and accordingly, we suggest concrete stepsforward for the research field.
MoreTranslated text
AI Read Science
Must-Reading Tree
Example
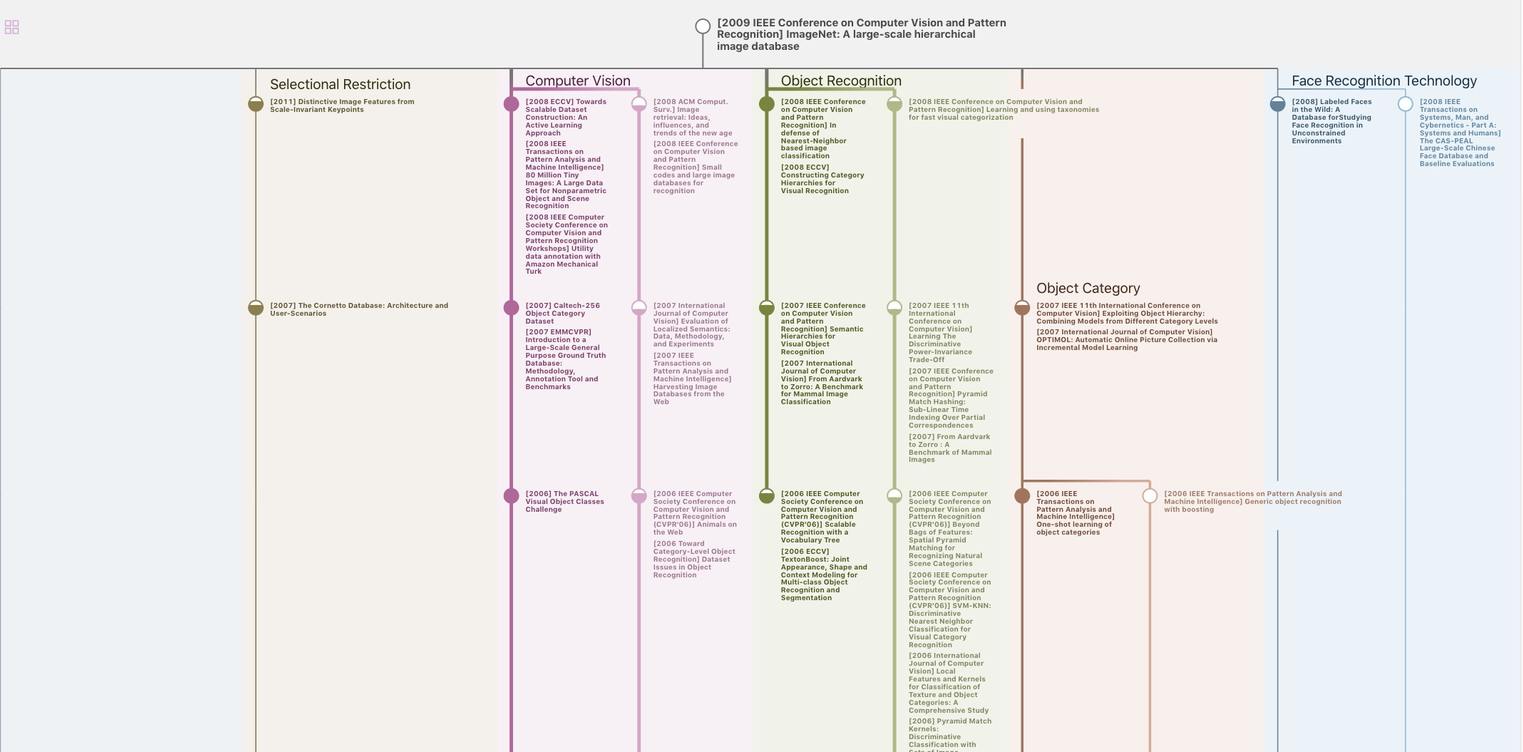
Generate MRT to find the research sequence of this paper
Chat Paper
Summary is being generated by the instructions you defined