DreamScene: 3D Gaussian-based Text-to-3D Scene Generation via Formation Pattern Sampling
arxiv(2024)
摘要
Text-to-3D scene generation holds immense potential for the gaming, film, and
architecture sectors. Despite significant progress, existing methods struggle
with maintaining high quality, consistency, and editing flexibility. In this
paper, we propose DreamScene, a 3D Gaussian-based novel text-to-3D scene
generation framework, to tackle the aforementioned three challenges mainly via
two strategies. First, DreamScene employs Formation Pattern Sampling (FPS), a
multi-timestep sampling strategy guided by the formation patterns of 3D
objects, to form fast, semantically rich, and high-quality representations. FPS
uses 3D Gaussian filtering for optimization stability, and leverages
reconstruction techniques to generate plausible textures. Second, DreamScene
employs a progressive three-stage camera sampling strategy, specifically
designed for both indoor and outdoor settings, to effectively ensure
object-environment integration and scene-wide 3D consistency. Last, DreamScene
enhances scene editing flexibility by integrating objects and environments,
enabling targeted adjustments. Extensive experiments validate DreamScene's
superiority over current state-of-the-art techniques, heralding its
wide-ranging potential for diverse applications. Code and demos will be
released at https://dreamscene-project.github.io .
更多查看译文
AI 理解论文
溯源树
样例
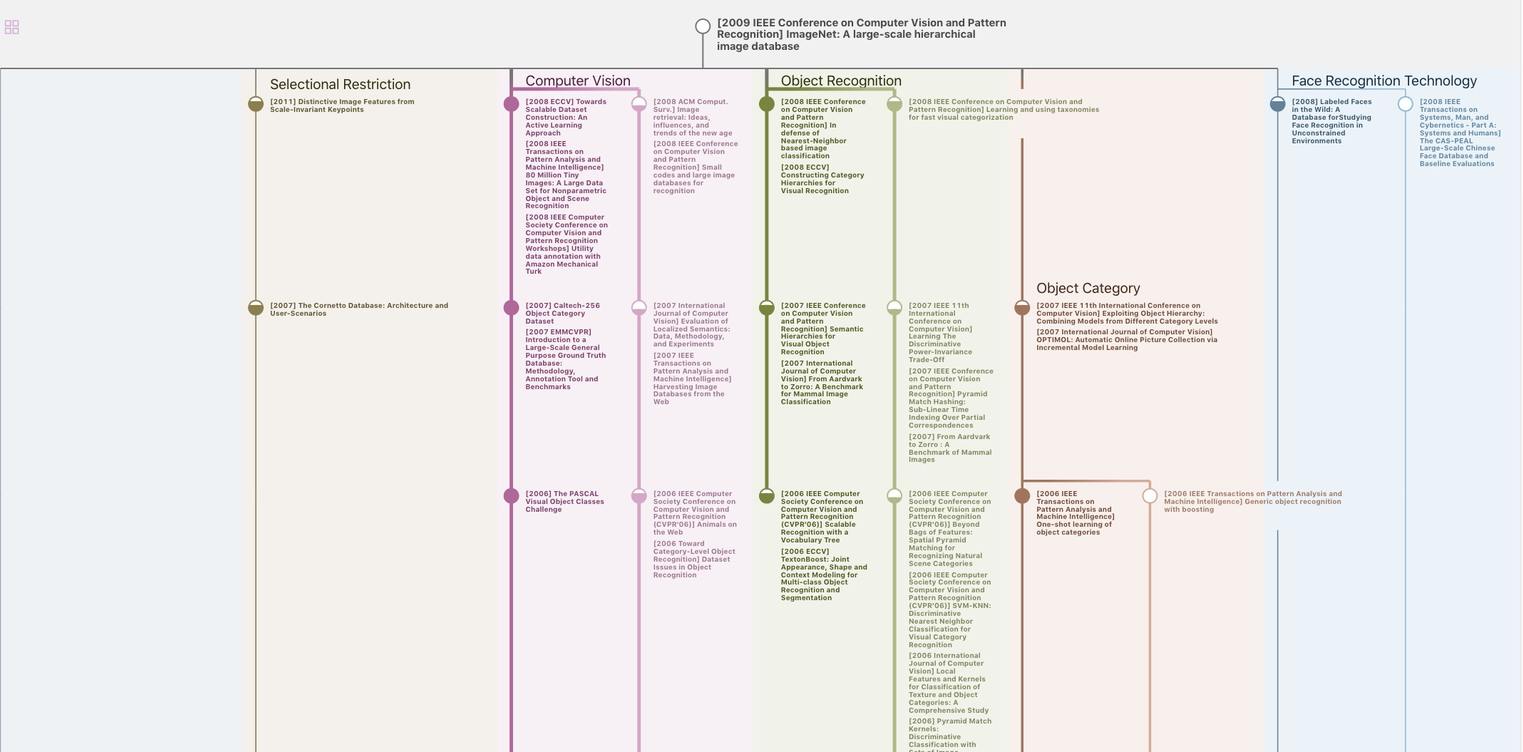
生成溯源树,研究论文发展脉络
Chat Paper
正在生成论文摘要