PAC-learning of free-fermionic states is NP-hard
arxiv(2024)
摘要
Free-fermionic states, also known as matchgates or Gaussian states, are a
fundamental class of quantum states due to their efficient classical
simulability and their crucial role across various domains of Physics. With the
advent of quantum devices, experiments now yield data from quantum states,
including estimates of expectation values. We establish that deciding whether a
given dataset, formed by a few Majorana correlation functions estimates, can be
consistent with a free-fermionic state is an NP-complete problem. Our result
also extends to datasets formed by estimates of Pauli expectation values. This
is in stark contrast to the case of stabilizer states, where the analogous
problem can be efficiently solved. Moreover, our results directly imply that
free-fermionic states are computationally hard to properly PAC-learn, where
PAC-learning of quantum states is a learning framework introduced by Aaronson.
Remarkably, this is the first class of classically simulable quantum states
shown to have this property.
更多查看译文
AI 理解论文
溯源树
样例
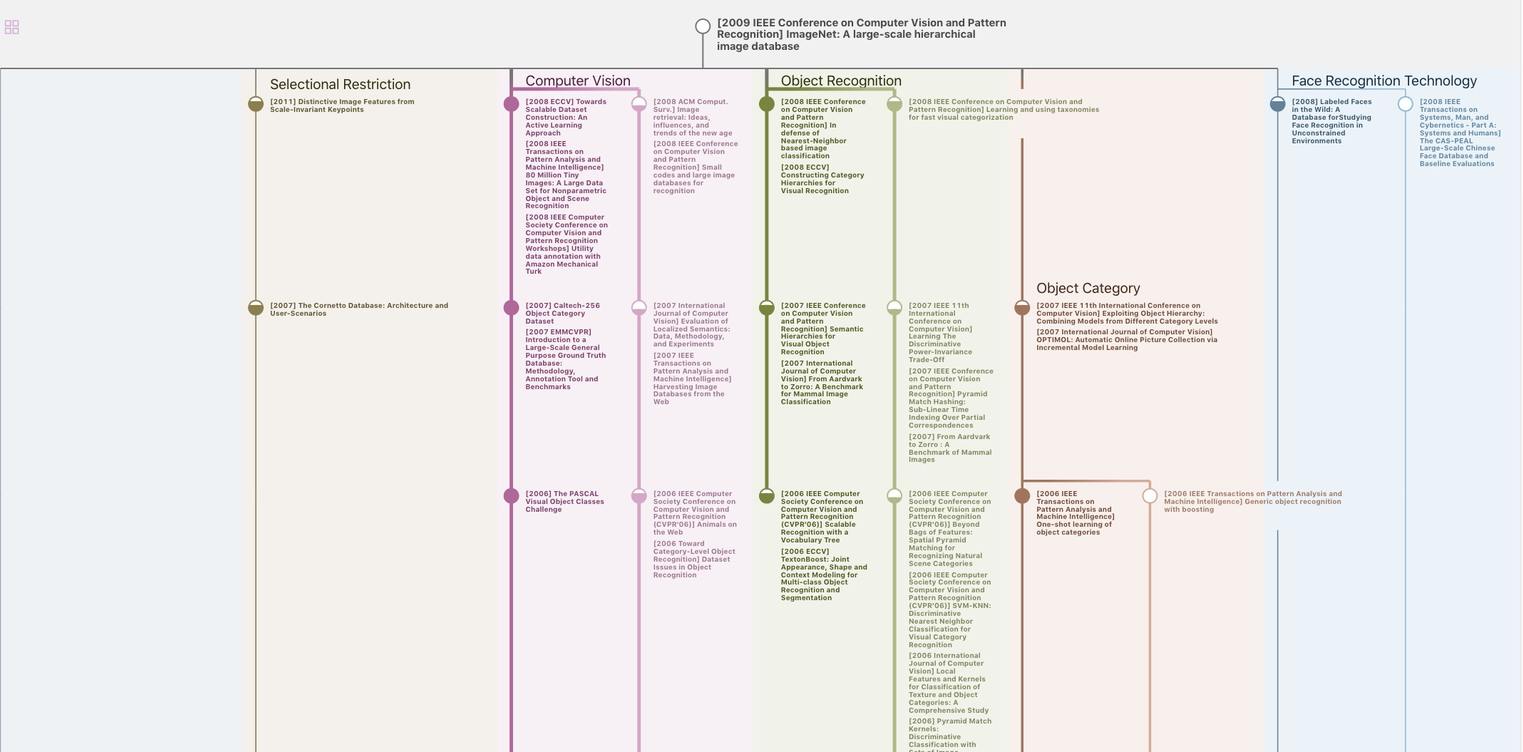
生成溯源树,研究论文发展脉络
Chat Paper
正在生成论文摘要