A tensile properties-related fatigue strength predicted machine learning framework for alloys used in aerospace
Engineering Fracture Mechanics(2024)
摘要
A tensile properties-related fatigue strength prediction framework based on machine learning (ML) methods was proposed. Firstly, 200 data containing six materials used in aerospace were collected. Secondly, tensile properties-related ML framework, including residual neural network (ResNet) model, gradient boosting decision tree (GBDT) model and LightGBM (LGB) model, was established to predict fatigue strength under symmetric cycles (R = -1) after features correlation analysis. Then, Gaussian noise was introduced into the features to prove the robustness and feature sensitivity of proposed ML framework. Finally, materials characteristics of fatigue resistance in the next generation were discussed after the analysis of the correlation between the tensile properties and fatigue strength. Compared with ± 50 % error band of classical prediction models, the prediction results of proposed ML framework located in the ± 10 % error band, especially for special crystal orientations and stress ratios. In practical applications, the tensile properties-related ML framework could be applied efficiently to materials fatigue strength prediction used in aerospace due to its robust prediction accuracy and generalization ability without additional parameters fitting.
更多查看译文
关键词
Fatigue strength,Machine learning framework,Tensile properties,Aerospace alloys
AI 理解论文
溯源树
样例
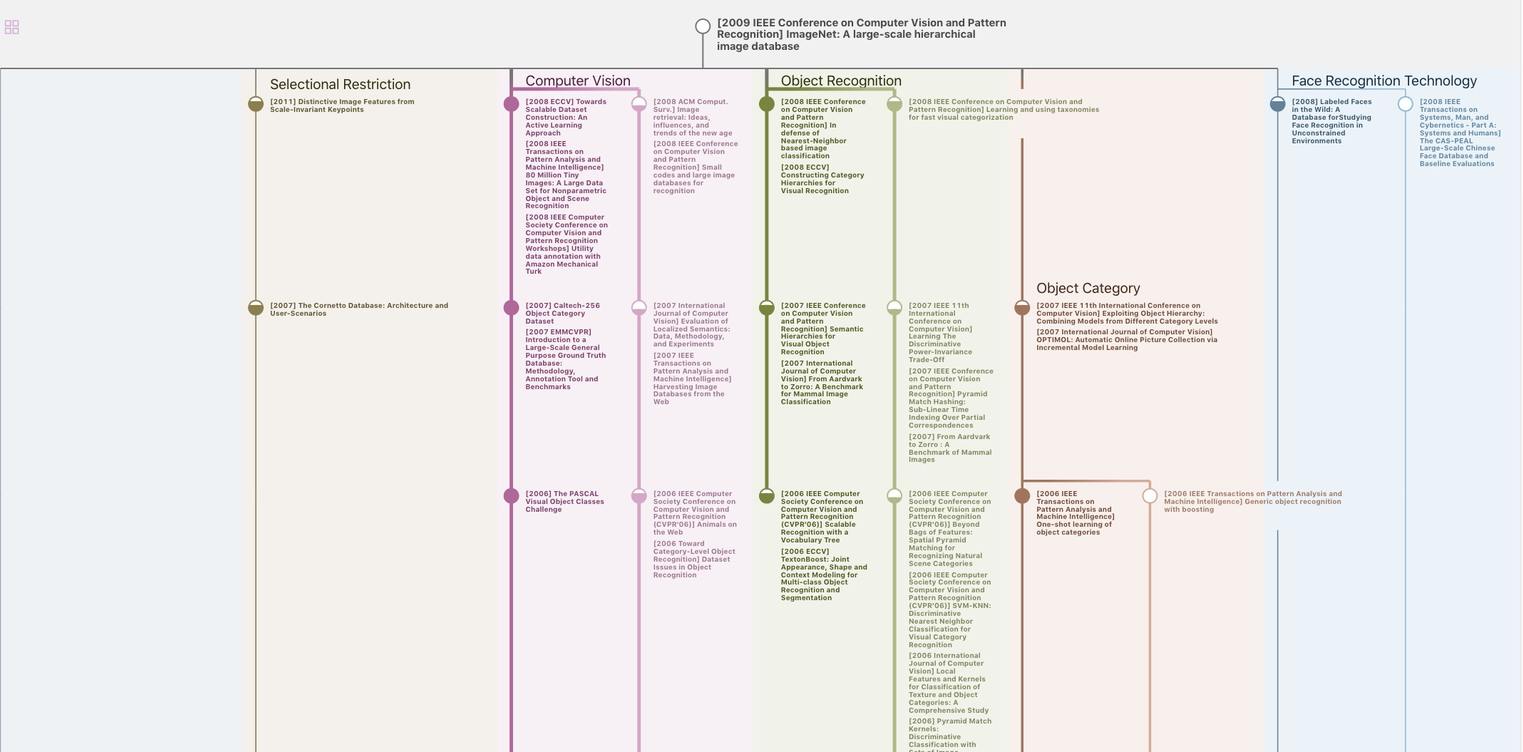
生成溯源树,研究论文发展脉络
Chat Paper
正在生成论文摘要