Layer-by-layer coating of Lacticaseibacillus rhamnosus GG (LGG) using chitosan and zein/tween-80/fucoidan nanoparticles to enhance LGG's survival under adverse conditions
Food Hydrocolloids(2024)
摘要
Lacticaseibacillus rhamnosus GG (LGG) is a widely recognized probiotic known for its health-promoting properties, such as supporting digestive and oral health, bolstering immune function, aiding in weight management, and potentially enhancing psychological well-being. However, unlocking its full potential necessitates addressing challenges like extending its shelf life and fortifying its resilience against harsh acidic conditions in the gastrointestinal tract. In this study, we employed a layer-by-layer (LbL) technique, using oppositely charged polymers — chitosan (CHI) and zein/tween-80/fucoidan nanoparticles (ZTFD), to encapsulate LGG, aiming to enhance LGG's functional attributes and viability. Our hypothesis centered on LBL encapsulation's potential to bolster LGG's survival and stability in adverse conditions by creating robust protective layers via electrostatic interactions between chitosan and ZTFD nanoparticles. Results demonstrated that (CHI/ZTFD)2-LGG, the two bi-layer coated variant, exhibited superior survival rates under extreme conditions such as freezing, heat, and storage, outperforming uncoated LGG. Moreover, in simulated gastrointestinal fluid over 120 min, plain LGG suffered a 3.92 log CFU/mL reduction, while (CHI/ZTFD)2-LGG experienced a milder 2.15 log CFU/mL reduction. In an in vivo mice study, (CHI/ZTFD)2-LGG displayed a remarkable 63.7-fold increase in survival compared to plain LGG. These findings highlight the potential of LbL encapsulation in enhancing LGG's robustness, enabling its use as a probiotic in food and pharmaceutical applications. The incorporation of encapsulated LGG into formulations holds promise for advancing probiotic utilization and enhancing consumer health.
更多查看译文
关键词
Probiotics,Survivability,Single cell encapsulation,Delivery system,Electrostatic interactions,In vivo study
AI 理解论文
溯源树
样例
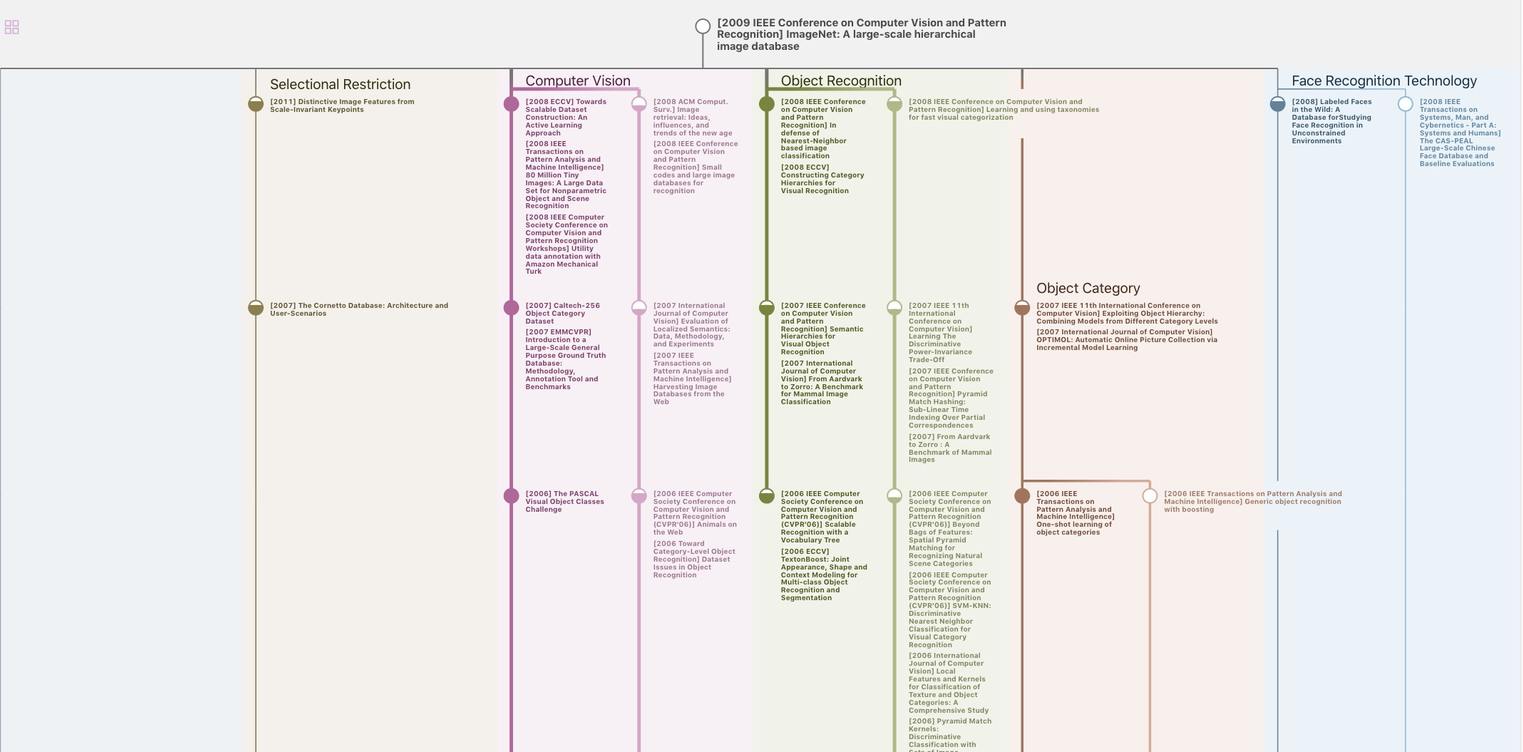
生成溯源树,研究论文发展脉络
Chat Paper
正在生成论文摘要