Depression symptoms modelling from social media text: an LLM driven semi-supervised learning approach
Language Resources and Evaluation(2024)
摘要
A fundamental component of user-level social media language based clinical depression modelling is depression symptoms detection (DSD). Unfortunately, there does not exist any DSD dataset that reflects both the clinical insights and the distribution of depression symptoms from the samples of self-disclosed depressed population. In our work, we describe a semi-supervised learning (SSL) framework which uses an initial supervised learning model that leverages (1) a state-of-the-art large mental health forum text pre-trained language model further fine-tuned on a clinician annotated DSD dataset, (2) a Zero-Shot learning model for DSD, and couples them together to harvest depression symptoms related samples from our large self-curated depressive tweets repository (DTR). Our clinician annotated dataset is the largest of its kind. Furthermore, DTR is created from the samples of tweets in self-disclosed depressed users Twitter timeline from two datasets, including one of the largest benchmark datasets for user-level depression detection from Twitter. This further helps preserve the depression symptoms distribution of self-disclosed tweets. Subsequently, we iteratively retrain our initial DSD model with the harvested data. We discuss the stopping criteria and limitations of this SSL process, and elaborate the underlying constructs which play a vital role in the overall SSL process. We show that we can produce a final dataset which is the largest of its kind. Furthermore, a DSD and a Depression Post Detection model trained on it achieves significantly better accuracy than their initial version.
更多查看译文
关键词
Semi-supervised learning,Zero-shot learning,Depression symptoms detection,Depression detection,Bidirectional Encoder Representations from Transformers (BERT),Mental-BERT
AI 理解论文
溯源树
样例
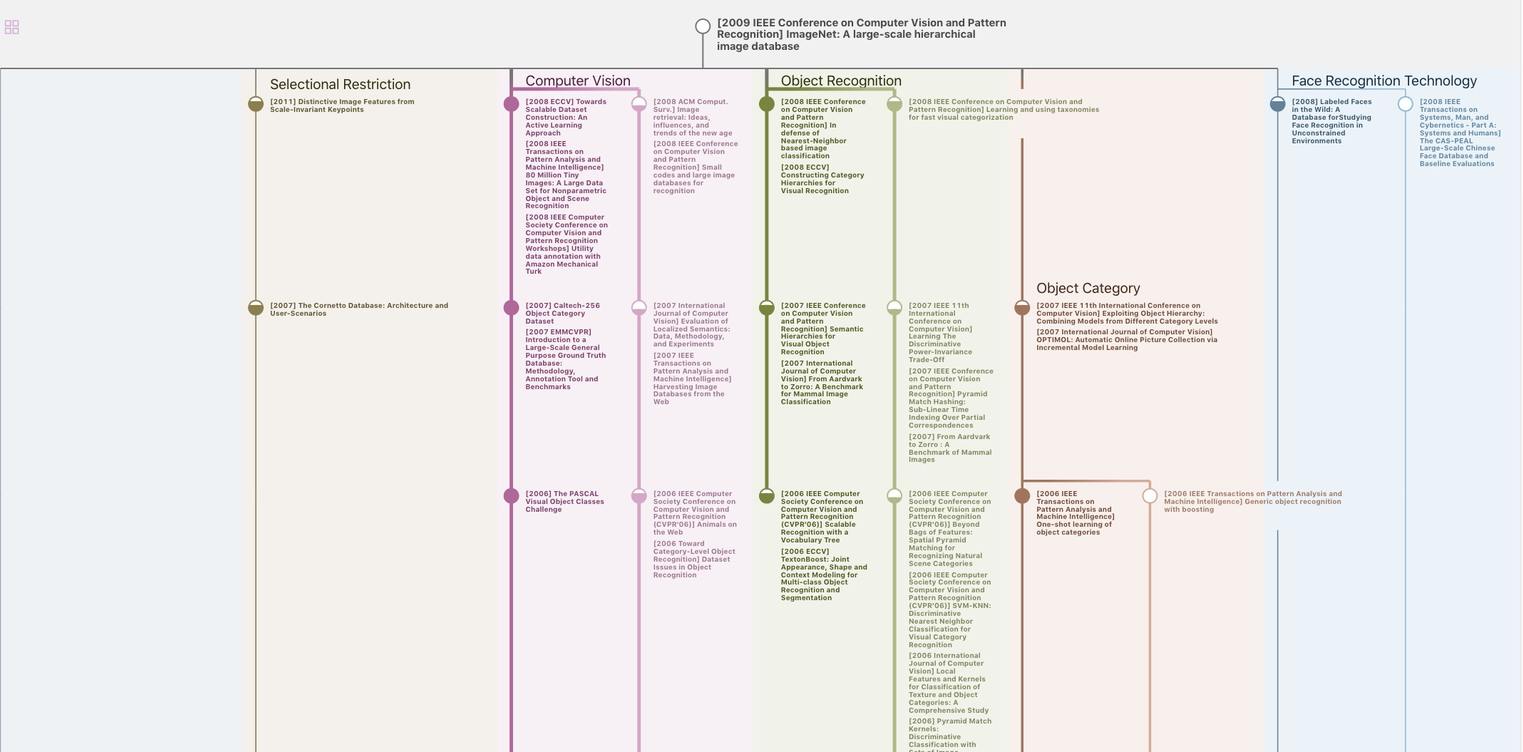
生成溯源树,研究论文发展脉络
Chat Paper
正在生成论文摘要