Evaluation and Comparison of 5G, WiFi, and Fusion With Incomplete Maps for Indoor Localization
IEEE ACCESS(2024)
摘要
Precise positioning will play a key role in future 5G/6G services. The upcoming location-based services drive the necessity of high-precision positioning to indoors. In fingerprinting, which is the most commonly used location algorithm indoors, comprehensive radio maps are essential for a precise localization service and highly influence on the result of the final position of the user. A Machine Learning (ML) algorithm that supports missing reference points information when maps are incomplete that are used during the training phase may improve the robustness and reliability of the localization service. In this work, we compare the performance of the classical fingerprinting technique and different Decision Tree Regressor (DTR)-based algorithms that are Decision Tree Adaboost (DTA), Linear Tree Adaboost (LTA) and Random Forest (RF). The experiments were carried out with real 5G and WiFi data in an indoor scenario to test the performance of the techniques. Additionally, we demonstrate the benefits of fusion of technologies when positioning with radio maps. Finally, an evaluation of the robustness from the different methods was carried out when missing information in radio maps during the training phase.
更多查看译文
关键词
Fingerprint recognition,Training,Wireless fidelity,5G mobile communication,Vectors,Location awareness,Estimation,Indoor communication,Fingerprinting,adaboost,random forest,5G,WiFi,fusion,indoor localization
AI 理解论文
溯源树
样例
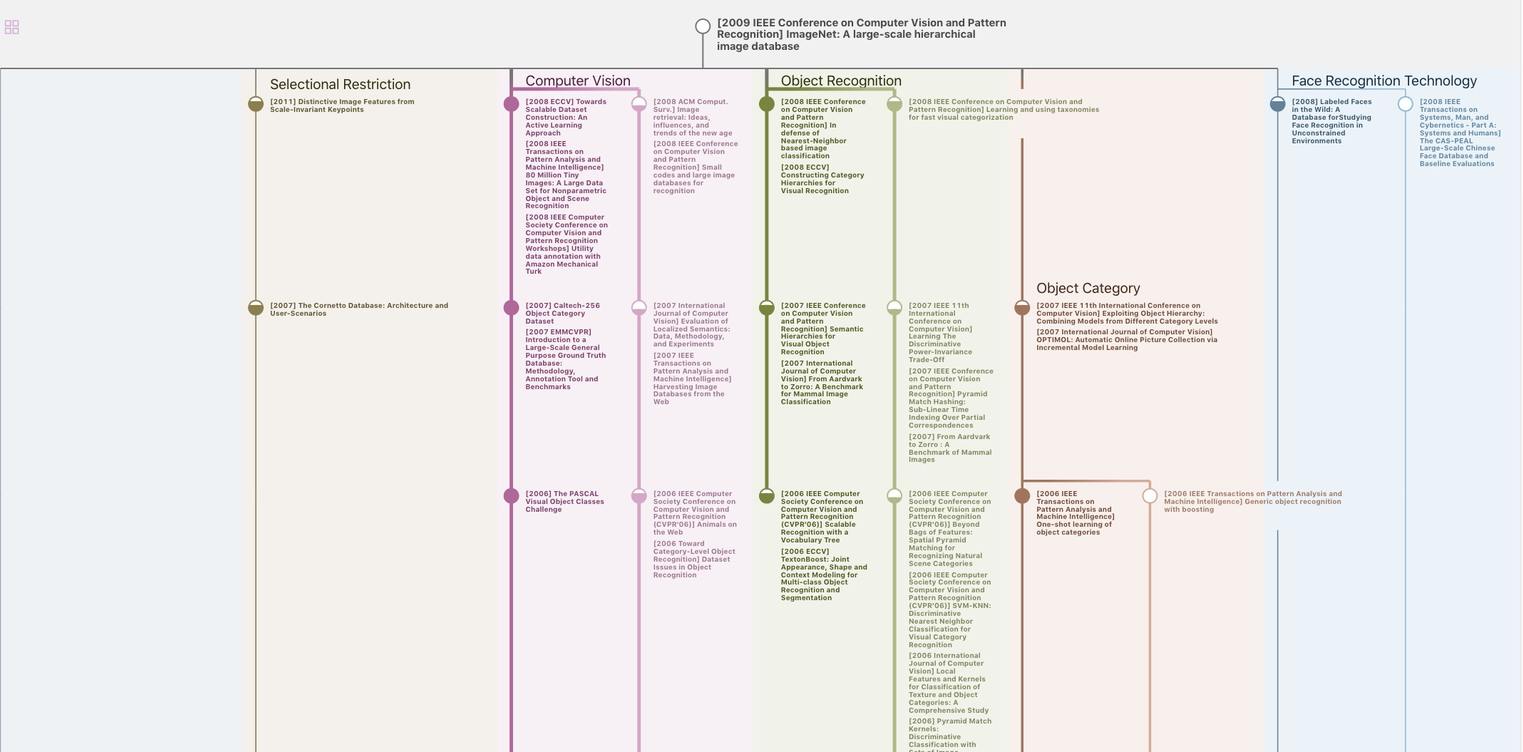
生成溯源树,研究论文发展脉络
Chat Paper
正在生成论文摘要