Investigating Biologically Plausible Neural Networks for Reservoir Computing Solutions
IEEE Access(2024)
摘要
While deep learning and backpropagation continue to dominate the field of machine learning in terms of benchmarks and versatility, recent neuroscientific advances shed light on more biologically plausible approaches. Spiking neural networks (SNNs), modelled after action potential dynamics, offer inherent time sensitivity and more efficiency in terms of performance to complexity. While investigating paradigms to support such alternatives, we attempt to answer whether reservoir computing can benefit from a spiking network based implementation with elements of biologically realistic models. This is done by varying both hyper-parameters and reservoir generation approaches and comparing implementations to spot potential improvements. We demonstrate how customized training of SNNs can result in competitive performance levels at lower operational complexity and be readily applied to other paradigms, such as the development of reservoir dynamics.
更多查看译文
关键词
reservoir computing,spiking neural networks,bio-plausible algorithms,leaky integrate-and-fire,LIF
AI 理解论文
溯源树
样例
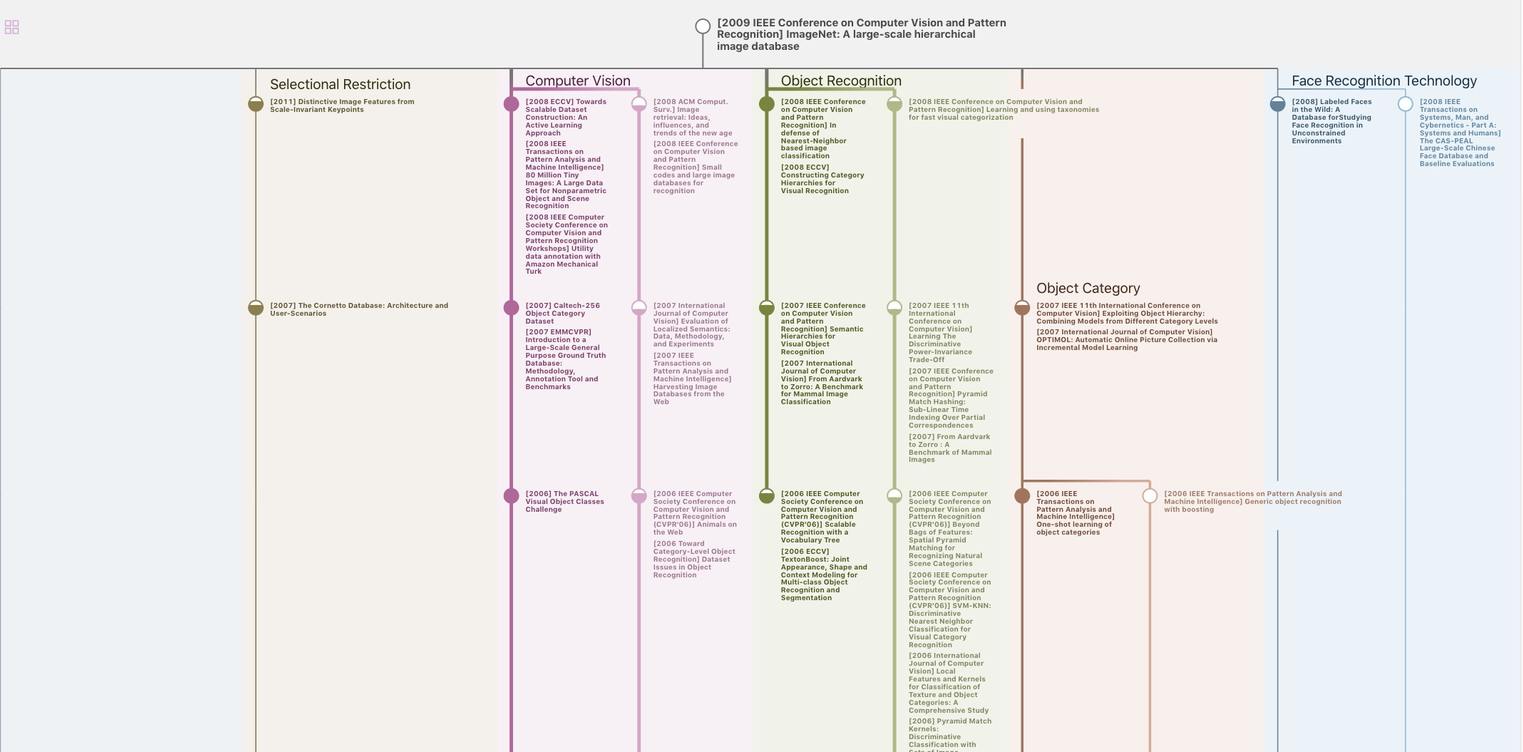
生成溯源树,研究论文发展脉络
Chat Paper
正在生成论文摘要