TrustBCFL: Mitigating Data Bias in IoT Through Blockchain-Enabled Federated Learning
IEEE Internet of Things Journal(2024)
摘要
The development of the Internet of Things (IoT), Big Data, and deep learning technologies has brought convenience to people’s lives. As personal privacy data protection laws and regulations tighten, the cost of acquiring high-quality annotated data from vast IoT datasets has significantly increased, resulting in prevalent issues such as data acquisition challenges and label noise in training data. In this work, we focus on the demand for privacy protection and trustworthy sharing of IoT data, and propose a method for addressing data bias in IoT through federated learning and blockchain by utilizing the theory of local intrinsic dimension (LID), incorporating committee consensus to achieve noise label identification and correction at the data level, reducing information loss in the training data. Additionally, it performs screening of low-quality local model updates at the model level, leveraging blockchain technology that addresses the single point of failure issues in traditional federated learning, ensuring the performance and security of the federated learning models. Analysis, proof of convergence, and experimentations on the proposed framework demonstrate good security and robustness in noisy environments, effectively addressing data bias in intelligent IoT settings. In scenarios with a noise level of 0.3, 0.6, and 0.9, the average model accuracy improved respectively by 7.75%, 7.30%, and 14.04% compared to FedAvg. Similarly, when compared to FedCorr, the average improvement in model accuracy is 5.19%, 3.63%, and 8.74% respectively. Moreover, the training time remains within an acceptable range for all cases.
更多查看译文
关键词
Blockchain,Data Bias Correction,Federated Learning,Intelligent IoT,Smart Contract
AI 理解论文
溯源树
样例
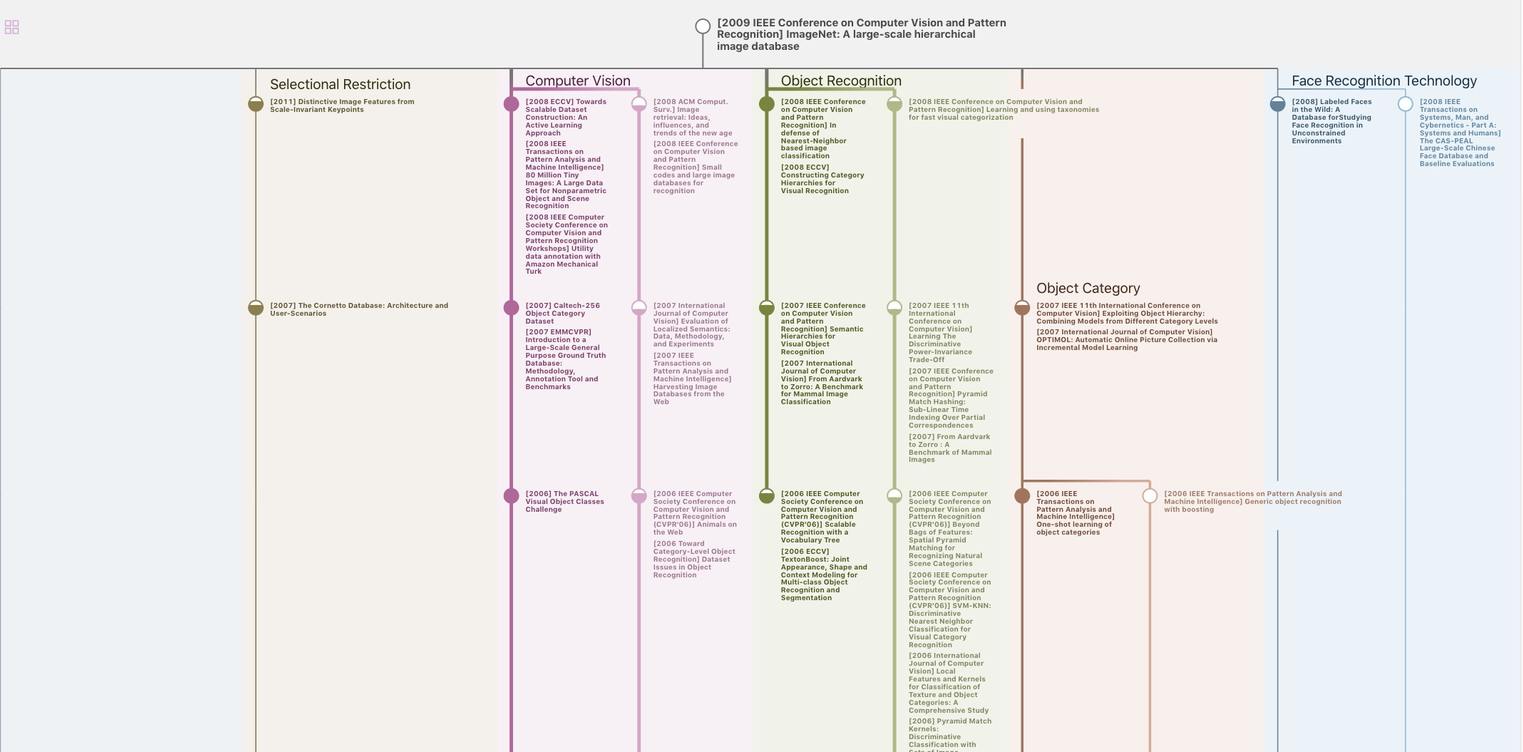
生成溯源树,研究论文发展脉络
Chat Paper
正在生成论文摘要