GNN-JFL: Graph Neural Network for Video SAR Shadow Tracking With Joint Motion-Appearance Feature Learning.
IEEE Trans. Geosci. Remote. Sens.(2024)
摘要
In this study, we address the challenges associated with Video Synthetic Aperture Radar (Video SAR) shadow tracking, a technique used for continuous monitoring of ground moving targets. Due to challenges such as changes in shadow appearance, low contrast between shadow and background, and scene occlusion in Video SAR, existing methods often encounter extensive matching errors in the data association process, resulting in unsatisfactory tracking performance. To overcome these issues, we propose a novel method, GNN-JFL, which is based on joint motion-appearance feature extraction and graph neural data association. This method uses the detector as a flexible plugin and introduces two key improvements in the tracker section to enhance tracking accuracy. Firstly, we introduce joint feature learning to extract the complementary appearance and motion features from shadow shapes and positions, obtaining more robust feature representations to improve tracking performance under intricate challenges. Secondly, by organically integrating Multi-object Tracking (MOT) problems and Graph Neural Networks (GNN), we propose a novel GNN-based shadow tracking architecture, which utilizes graph relationships to learn the associations between shadows for more accurate tracking predictions. Our method is validated using two measured datasets and demonstrate superior performance in terms of multi-object tracking accuracy (MOTA). It outperforms the suboptimal method by 4.2% and 3.6% in the two datasets, respectively. This research contributes to the advancement of continuous monitoring techniques employing Video SAR shadow tracking.
更多查看译文
关键词
Graph neural network,multi-shadow tracking,video synthetic aperture radar (Video SAR)
AI 理解论文
溯源树
样例
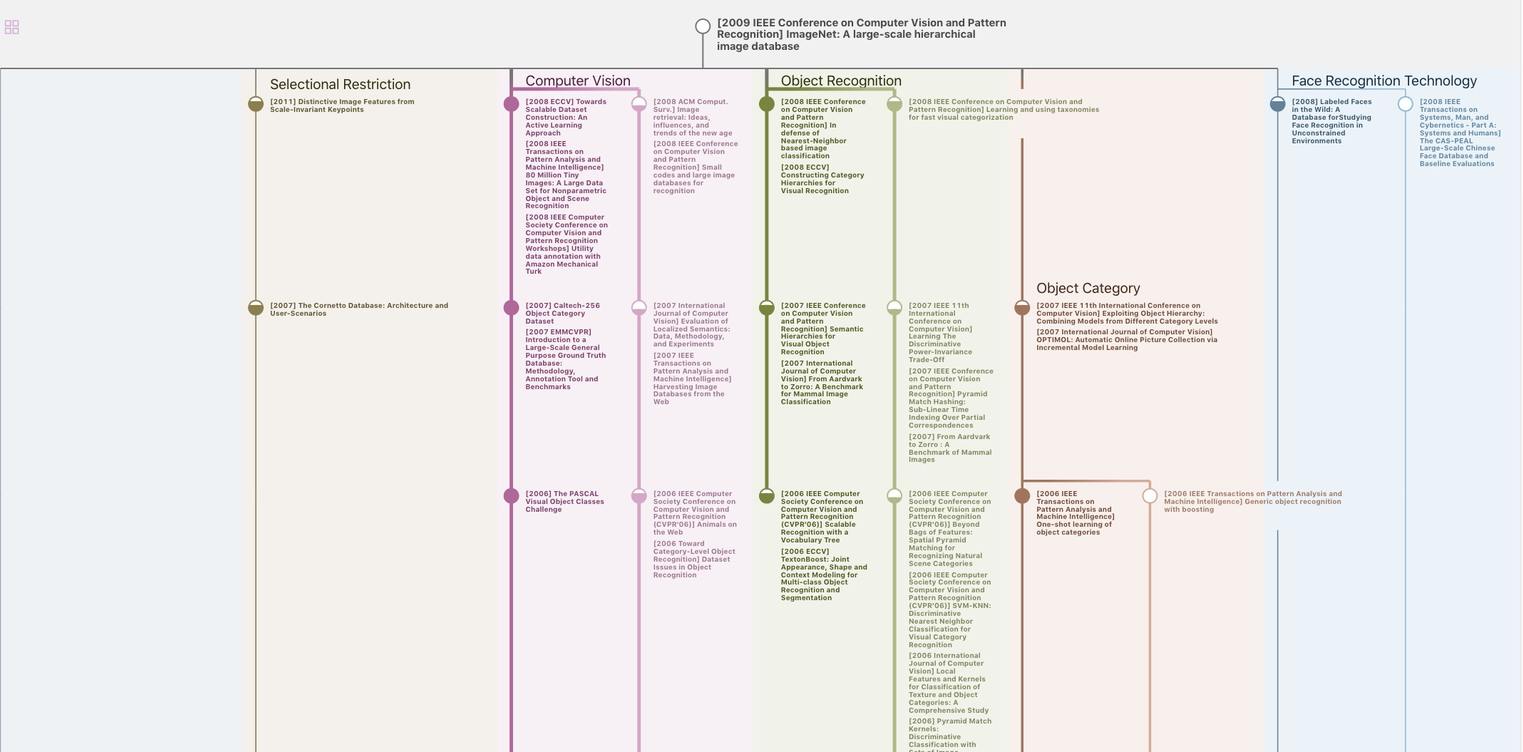
生成溯源树,研究论文发展脉络
Chat Paper
正在生成论文摘要