Exploring Generalizable Distillation for Efficient Medical Image Segmentation
IEEE Journal of Biomedical and Health Informatics(2024)
摘要
Efficient medical image segmentation aims to provide accurate pixel- wise predictions with a lightweight implementation framework. However, existing lightweight networks generally overlook the generalizability of the cross-domain medical segmentation tasks. In this paper, we propose Generalizable Knowledge Distillation (GKD), a novel framework for enhancing the performance of lightweight networks on cross-domain medical segmentation by generalizable knowledge distillation from powerful teacher networks. Considering the domain gaps between different medical datasets, we propose the Model-Specific Alignment Networks (MSAN) to obtain the domain-invariant representations. Meanwhile, a customized Alignment Consistency Training (ACT) strategy is designed to promote the MSAN training. Based on the domain-invariant vectors in MSAN, we propose two generalizable distillation schemes, Dual Contrastive Graph Distillation (DCGD) and Domain-Invariant Cross Distillation (DICD). In DCGD, two implicit contrastive graphs are designed to model the intra-coupling and inter-coupling semantic correlations. Then, in DICD, the domain-invariant semantic vectors are reconstructed from two networks (
i.e.
, teacher and student) with a crossover manner to achieve simultaneous generalization of lightweight networks, hierarchically. Moreover, a metric named Fréchet Semantic Distance (FSD) is tailored to verify the effectiveness of the regularized domain-invariant features. Extensive experiments conducted on the Liver, Retinal Vessel and Colonoscopy segmentation datasets demonstrate the superiority of our method, in terms of performance and generalization ability on lightweight networks. Our code will be available at GKD Framework.
更多查看译文
关键词
Medical Image Segmentation,Knowledge Distillation,Model Generalization,Contrastive Graph
AI 理解论文
溯源树
样例
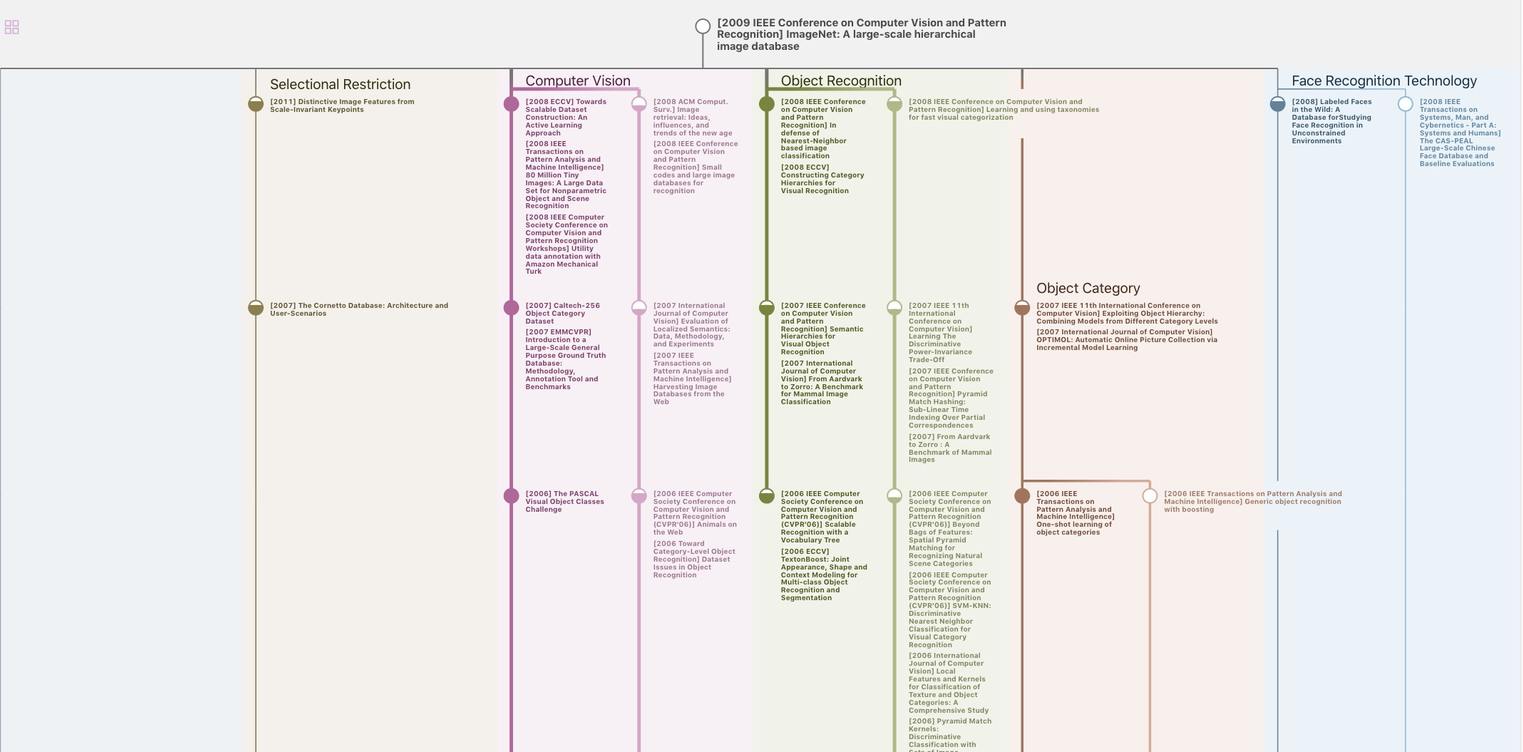
生成溯源树,研究论文发展脉络
Chat Paper
正在生成论文摘要