Smart Multimodal In-Bed Pose Estimation Framework Incorporating Generative Adversarial Neural Network
IEEE journal of biomedical and health informatics(2024)
摘要
Monitoring in-bed pose estimation based on the Internet of Medical Things (IoMT) and ambient technology has a significant impact on many applications such as sleep-related disorders including obstructive sleep apnea syndrome, assessment of sleep quality, and health risk of pressure ulcers. In this research, a new multimodal in-bed pose estimation has been proposed using a deep learning framework. The Simultaneously-collected multimodal Lying Pose (SLP) dataset has been used for performance evaluation of the proposed framework where two modalities including long wave infrared (LWIR) and depth images are used to train the proposed model. The main contribution of this research is the feature fusion network and the use of a generative model to generate RGB images having similar poses to other modalities (LWIR/depth). The inclusion of a generative model helps to improve the overall accuracy of the pose estimation algorithm. Moreover, the method can be generalized for situations to recover human pose both in home and hospital settings under various cover thickness levels. The proposed model is compared with other fusion-based models and shows an improved performance of 97.8% at PCKh@0.5. In addition, performance has been evaluated for different cover conditions, and under home and hospital environments which present improvements using our proposed model.
更多查看译文
关键词
Pose estimation,Sleep apnea,Monitoring,Bioinformatics,Feature extraction,Visualization,Hospitals,Internet of Medical Things,AI,SLP,Generative adversarial neural network,LWIR,depth
AI 理解论文
溯源树
样例
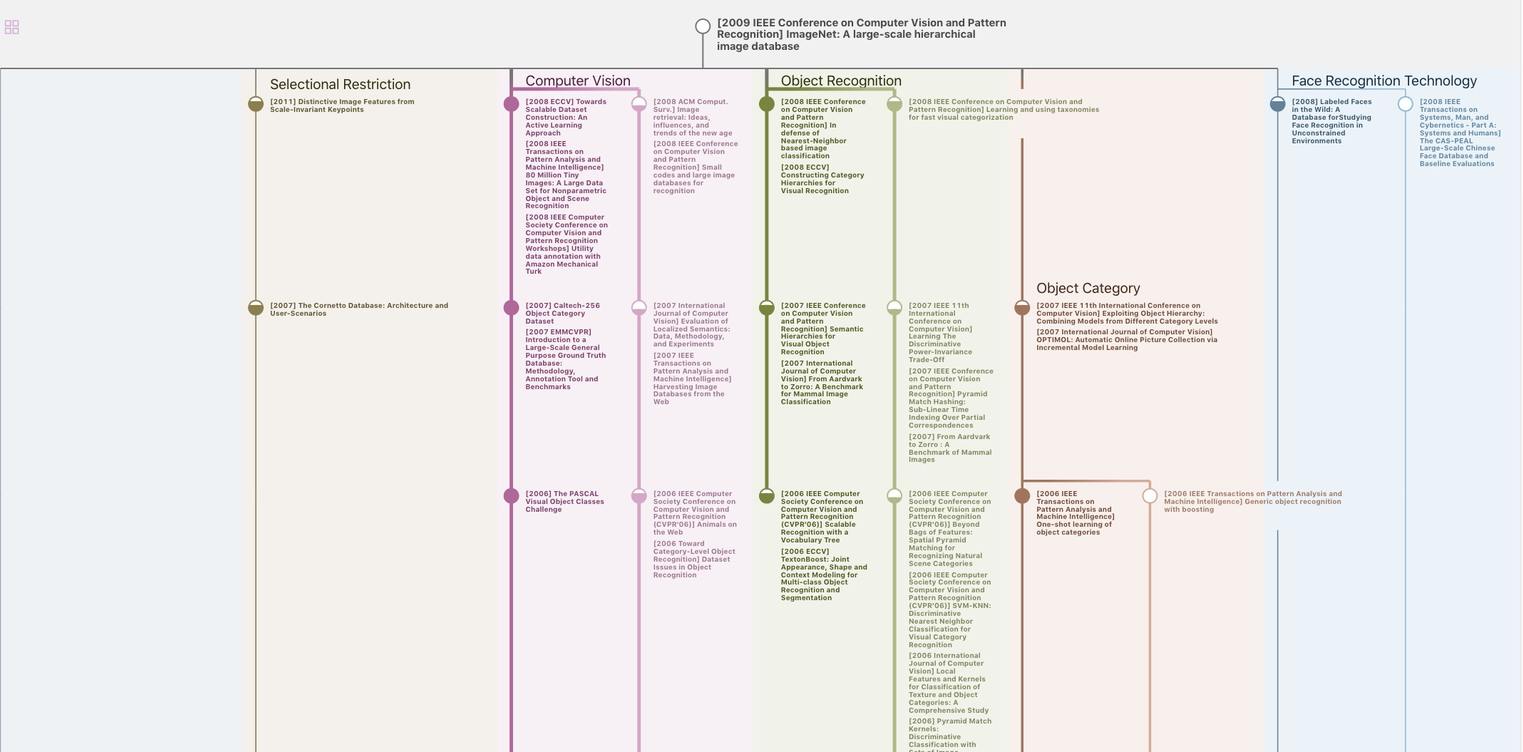
生成溯源树,研究论文发展脉络
Chat Paper
正在生成论文摘要