Pose-Guided Robust Action Recognition for Outdoor Internet of Things
IEEE Transactions on Consumer Electronics(2024)
摘要
Skeleton-based human recognition is a key technology for visual feedback, which can help the Internet of Things (IoT) interact with humans in a non-contact manner outdoors. Graph Convolutional Networks (GCNs), achieving an intuitive understanding of the human skeleton, have received increasing attention. Although current GCN-based works explore how to model unlinked body parts, they still show weak robustness in noisy solid data, such as joint/frame loss, which often happens in outdoor IoTs. Towards robust visual feedback, in the paper, we propose robust skeleton-based action recognition neural networks (Robust-SAR), a new cost-efficient approach for recognizing activities in noisy outdoor scenarios. Instead of estimating 2D or 3D skeleton coordinates, we first extract the heatmap of human poses from videos. We propose S-pose to learn heatmap at multiple levels, i.e., joint, joint-scale, and part-scale learning, boosting higher-order motion pattern learning. Additionally, we propose T-pose to adaptively employ the features of previous frames to enhance the current frame, further enhancing the robustness of spatiotemporal human representation. Experimentally, Robust-SAR achieves state-of-the-art recognition results on four benchmarks, including NTU-60, NTU-120, NUCLA, and Kinetics-400 (outdoor datasets). Furthermore, in noise-filled outdoor conditions, the performance of Robust-SAR only drops by about 0.5%, while other state-of-the-art methods drop by about 2%.
更多查看译文
关键词
outdoor action recognition,IoT-enabled sensing,robust motion analysis,human pose
AI 理解论文
溯源树
样例
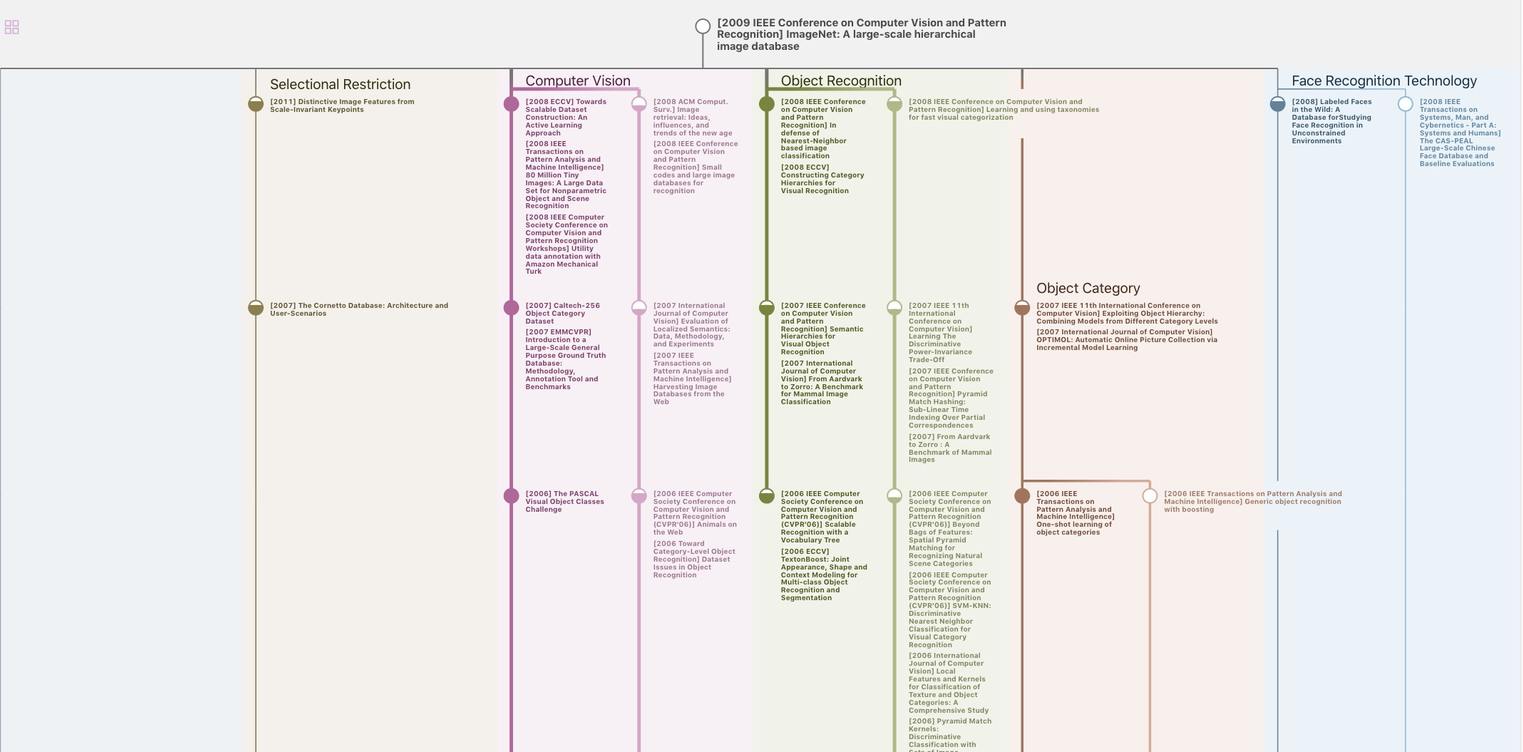
生成溯源树,研究论文发展脉络
Chat Paper
正在生成论文摘要