Deepfake Detection Fighting against Noisy Label Attack
IEEE Transactions on Multimedia(2024)
摘要
The face manipulation technique such as Deepfake has been widely used to create realistic faces, which raises growing concerns in the community. Based on the correct labeled data, the current Deepfake detectors are mostly trained on the clean dataset, usually resulting in the reliable high detection accuracy. However, in the real-world scenario, labelers possibly mislabel the data or malicious attackers always intend to poison the training data with incorrect label, namely noisy label attack, leading to poor detection results. To overcome the tough issue, we propose a Deepfake detection framework fighting against noisy label attack. Specifically, a Negative Sample Generator (NSG) utilizes the possibly-poisoned samples to generate labelreliable negative samples through simulating blending artifacts caused by Deepfake. Next, a Noise-immune Contrastive Learner (NiCL) takes both positive and negative samples as training data, exploring blending artifacts and intrinsic forgery clues to filtrate the noisy samples out. Moreover, relying on label purification, the filtrated noisy samples are further purified, which then are fed back to the feature extractor for the following model training. Extensive experiments on the benchmark datasets demonstrate the superiority of our proposed Deepfake detector. In particular, when fighting against noisy label attack, the high performance of the proposed detector is remarkably better than its competitors.
更多查看译文
关键词
Deepfake detection,noisy label attack,contrastive learning
AI 理解论文
溯源树
样例
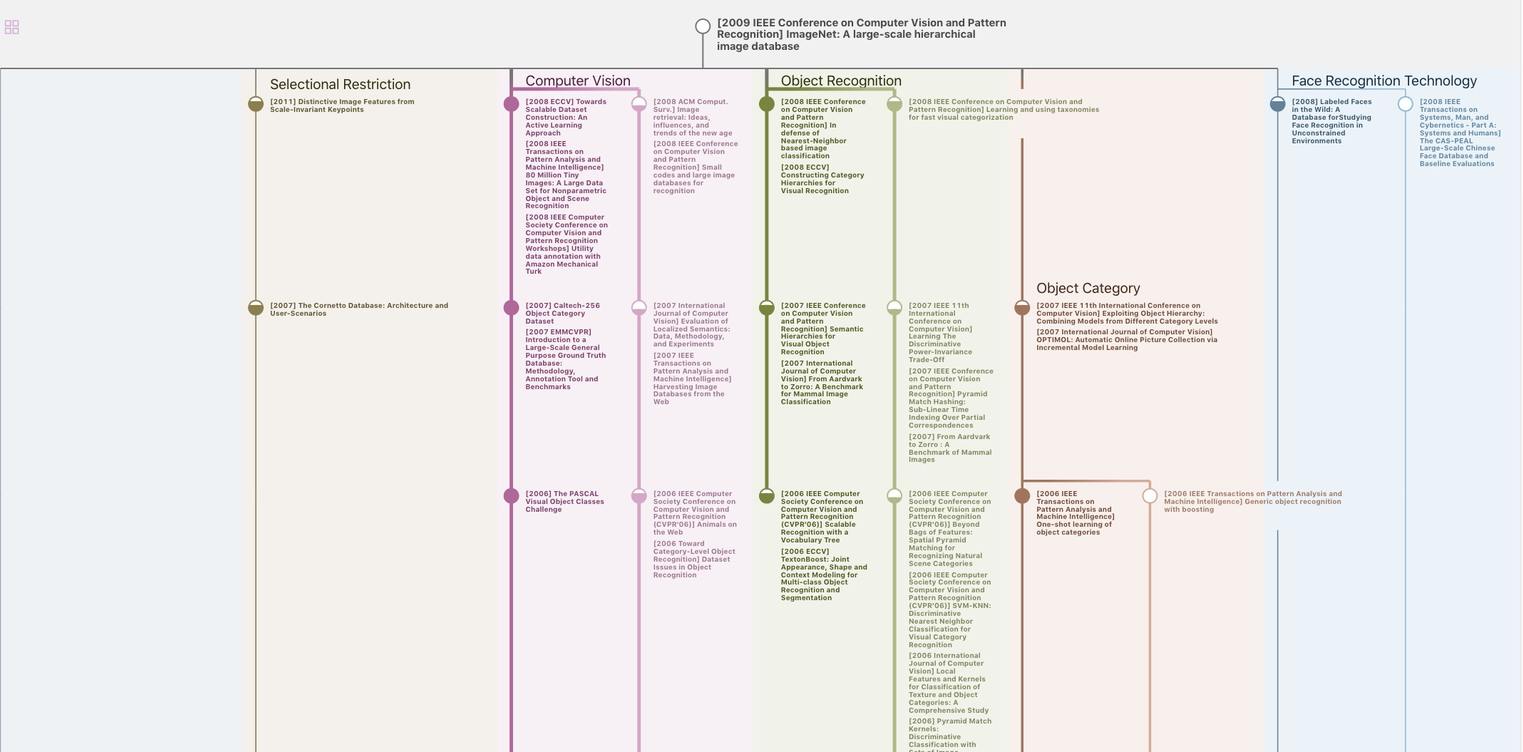
生成溯源树,研究论文发展脉络
Chat Paper
正在生成论文摘要