Automatic ECG Signal Quality Determination Using CNN with Optimal Hyperparameters for Quality-Aware Deep ECG Analysis Systems
IEEE Sensors Journal(2024)
摘要
Continuous monitoring of electrocardiogram (ECG) signal is made feasible with the progress in wearable technologies. The ECG signals are acquired and analyzed for health monitoring and diagnosis of cardiovascular diseases. However, ECG signals contaminated with various noises during acquisition must be screened to reduce false alarms during unsupervised health monitoring. Only clean signals should be analyzed for disease detection. We propose a robust and lightweight convolutional neural network (CNN) with an appropriate activation function, optimal number of convolution layers (CLs), and dense layers (DLs) for real-time and automatic ECG signal quality assessment (ECG-SQA) for an energy-constrained wearable health monitoring device with limited computing resources. The CNNs are trained and tested with standard ECG databases, which classify ECG signals as noisy and clean. The proposed optimal CNN for ECG-SQA has four CLs, five DLs, and an exponential linear unit (ELU) activation function. The optimal CNN for ECG-SQA has a sensitivity of 92.88%, 82.09%, and 99.64%, and specificity of 75%, 75.3%, and 73.97% for unseen databases of PhysioNet/Computing in Cardiology Challenge 2011, PhysioNet/Computing in Cardiology Challenge 2017, and St. Petersburg Institute of Cardiological Technics (INCART) 12-lead arrhythmia database, respectively. The proposed CNN has a model size of 5,633 kB, testing time of 121.00±39.77 ms, and energy consumption of 1851.3±608.48 mJ for quality assessment of 5 s ECG signal when implemented on Raspberry Pi as a real-time computing platform.
更多查看译文
关键词
Electrocardiogram,Convolutional Neural Network,Signal Quality Assessment,Wearable device
AI 理解论文
溯源树
样例
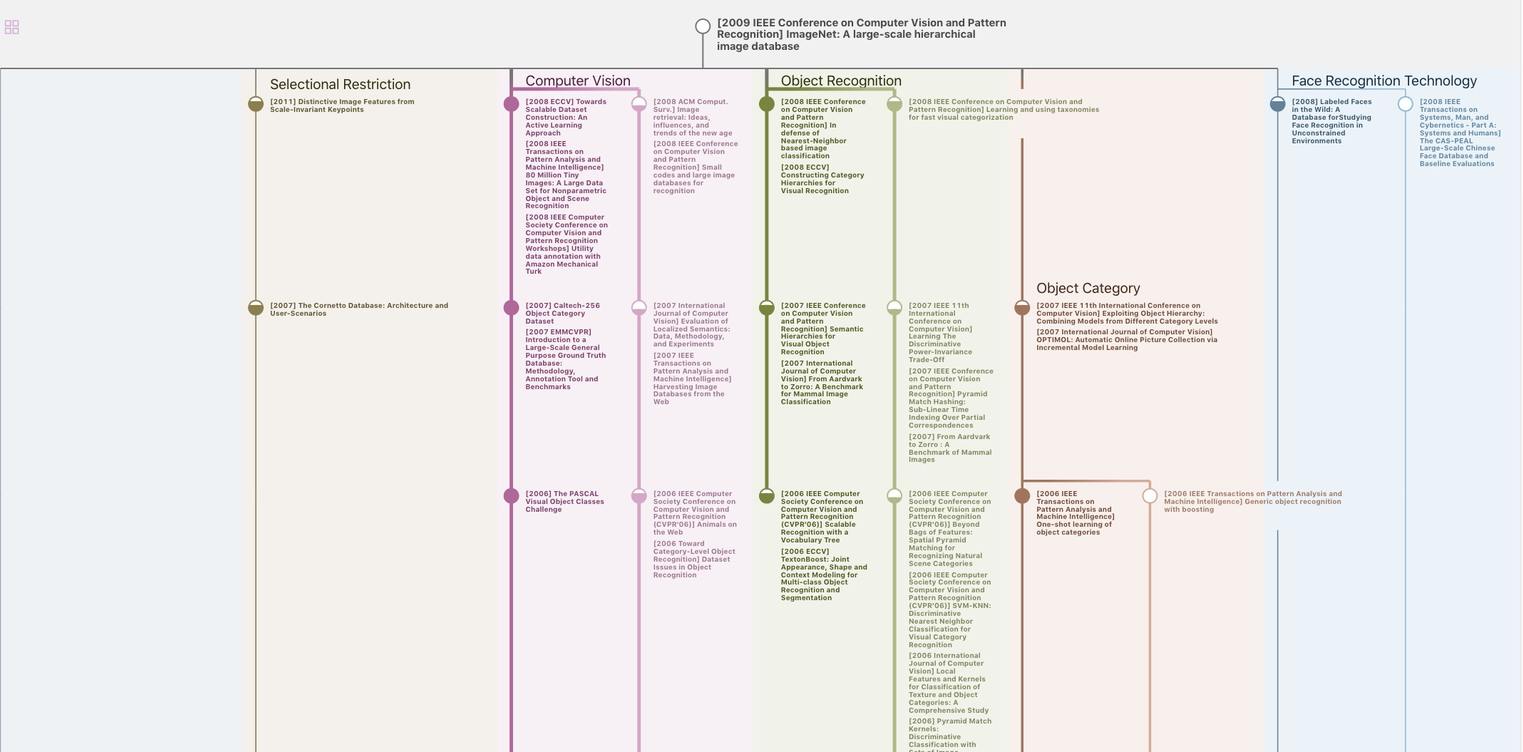
生成溯源树,研究论文发展脉络
Chat Paper
正在生成论文摘要