Combining Metamorphic Testing and Machine Learning to Enhance OpenStreetMap
IEEE TRANSACTIONS ON RELIABILITY(2024)
摘要
Metamorphic testing (MT) is a useful tool to test systems where an oracle is not available. MT relies on the definition of metamorphic relations (MR), that is, certain properties that relate a set of inputs and the set of outputs produced by the system under test (SUT) as response to these inputs. Usually, a violation of an MR implies that the SUT is faulty. However, some work on MT accepts, for certain SUTs, that the violation of an MR almost always is a symptom of an error but assumes the potential existence of false positives. This is the case, for instance, of our recent work where we applied MT to improve OpenStreetMap (OSM). Our MRs were able to uncover a large amount of errors in all the analyzed maps but we suffered the presence of a nonnegligible number of false positives. Therefore, an expert had to manually check the suspicious elements identified by our MRs. If we analyze large maps, then this manual task is unfeasible. In this article we solve the main limitation of our previous approach: we accurately and automatically discard false positives. Our new framework combines MT, along the same lines of our previous work, and machine learning. Specifically, we provide three models, one for each MR, based on the random forest model. The models were extensively trained with real data obtained from the application of MRs to maps of cities located in different continents. In order to evaluate the usefulness of our models, we tested them using different cities, in countries that were not considered in the training set. The results were very good: accuracy of the models is never lower than 0.90, it is usually much higher and in many situations reaches 1.0. The computation of the F1-scores yielded similar results.
更多查看译文
关键词
Urban areas,Testing,Manuals,Computational modeling,Training,Roads,Tagging,Machine learning (ML),metamorphic testing (MT),openstreetmap (OSM),quality of maps
AI 理解论文
溯源树
样例
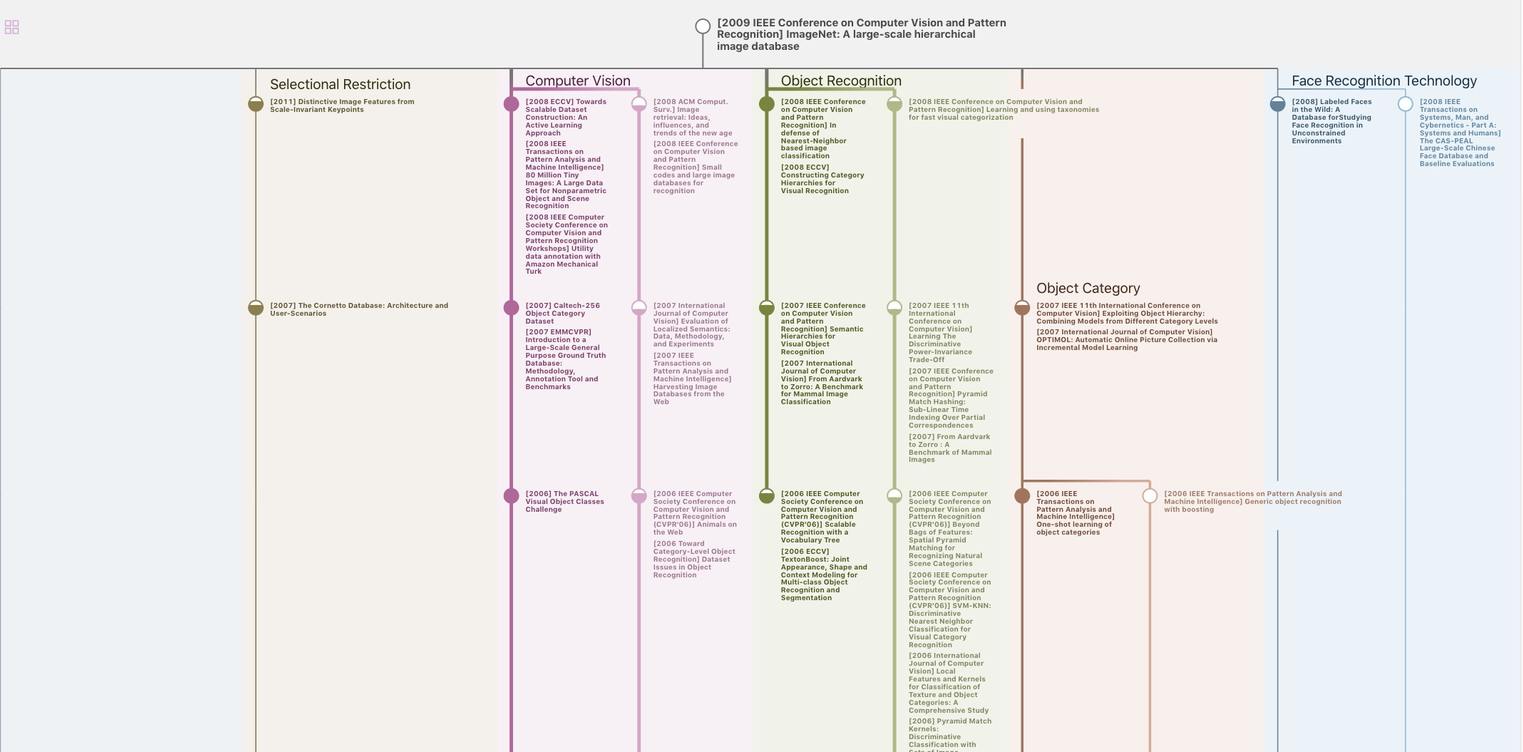
生成溯源树,研究论文发展脉络
Chat Paper
正在生成论文摘要