Analysis of dissolved oxygen influencing factors and concentration prediction using input variable selection technique: A hybrid machine learning approach
Journal of Environmental Management(2024)
摘要
Accurate quantification of dissolved oxygen (DO) is critically important for the protection and management of aquatic ecosystems. Successful applications have utilized mechanistic and data-driven models to simulate DO content in aquatic ecosystems. However, mechanistic models present challenges due to their complex and difficult-to-solve conditions, making them less portable. Additionally, data-driven model predictions are hindered by the challenge of numerous input variables, impacting both the running speed and prediction performance of the model. To address these challenges, water quality data and meteorological data of the Tanjiang River were obtained. The maximum information coefficient (MIC) input variable selection technique was employed to identify primary environmental factors influencing DO changes. Furthermore, coupled with support vector regression (SVR), two models (SVR and MIC-SVR) were employed to estimate the DO concentration of the Tanjiang River, and the optimal model was established. The results indicated a shift in the primary pollution factor from ammonia nitrogen to total phosphorus after recent treatment in the Tanjiang River. In comparison with the SVR model, the root mean square error (RMSE) of the MIC-SVR model was reduced by 4.46%, and the Nash-efficiency coefficient (NSE) was improved by 45.85%. In addition, study of kernel function selection revealed that considering as many kernel functions as possible is necessary for improving the performance of the SVR model. Conclusively, the proposed MIC-SVR model serves as an effective tool to analyze the relationship between DO and environmental factors, identifying the primary causes of low DO, and accurately predict the DO concentration in the Tanjiang River (especially in its middle and lower reaches), thus providing a reference for governmental decision-making on water environmental protection and water resource management.
更多查看译文
关键词
DO prediction,Support vector machine,Maximum information coefficient,Kernel function selection,Analysis of impact factors
AI 理解论文
溯源树
样例
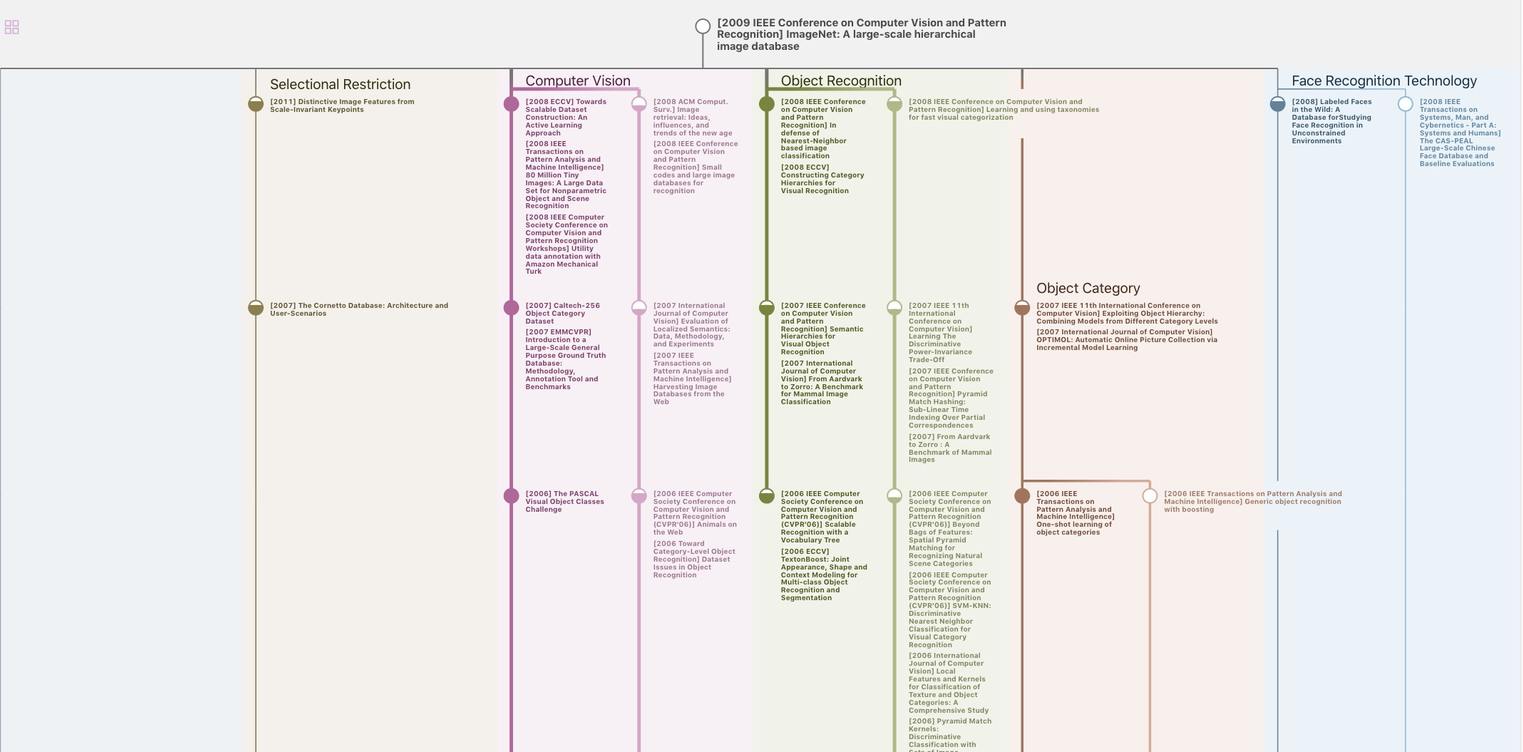
生成溯源树,研究论文发展脉络
Chat Paper
正在生成论文摘要