Domain Knowledge Transfer for Feature Exploration
2023 IEEE Asia-Pacific Conference on Computer Science and Data Engineering (CSDE)(2023)
摘要
Transferring domain knowledge is crucial for effective feature exploration in data analysis and machine learning. Given the extensive amount of data available today, no dataset remains entirely novel within its domain. However, our approach to handling datasets by segregating them from domain knowledge may lead us to overlook insights that could significantly enhance the process of comprehending the data. When delving into familiar knowledge using novel datasets, there's a risk of duplicating efforts and wasting resources and time. To address this, we introduce an implementation strategy aimed at incorporating domain knowledge into a fresh dataset. This strategy involves categorizing the attributes within the dataset into classes. By analyzing the class counts for each attribute, we propose generating associative rules. These rules can then be integrated into the new dataset as additional attributes, effectively infusing it with domain knowledge.
更多查看译文
关键词
nominal data,feature selection,feature extraction,domain knowledge,pivot,classification
AI 理解论文
溯源树
样例
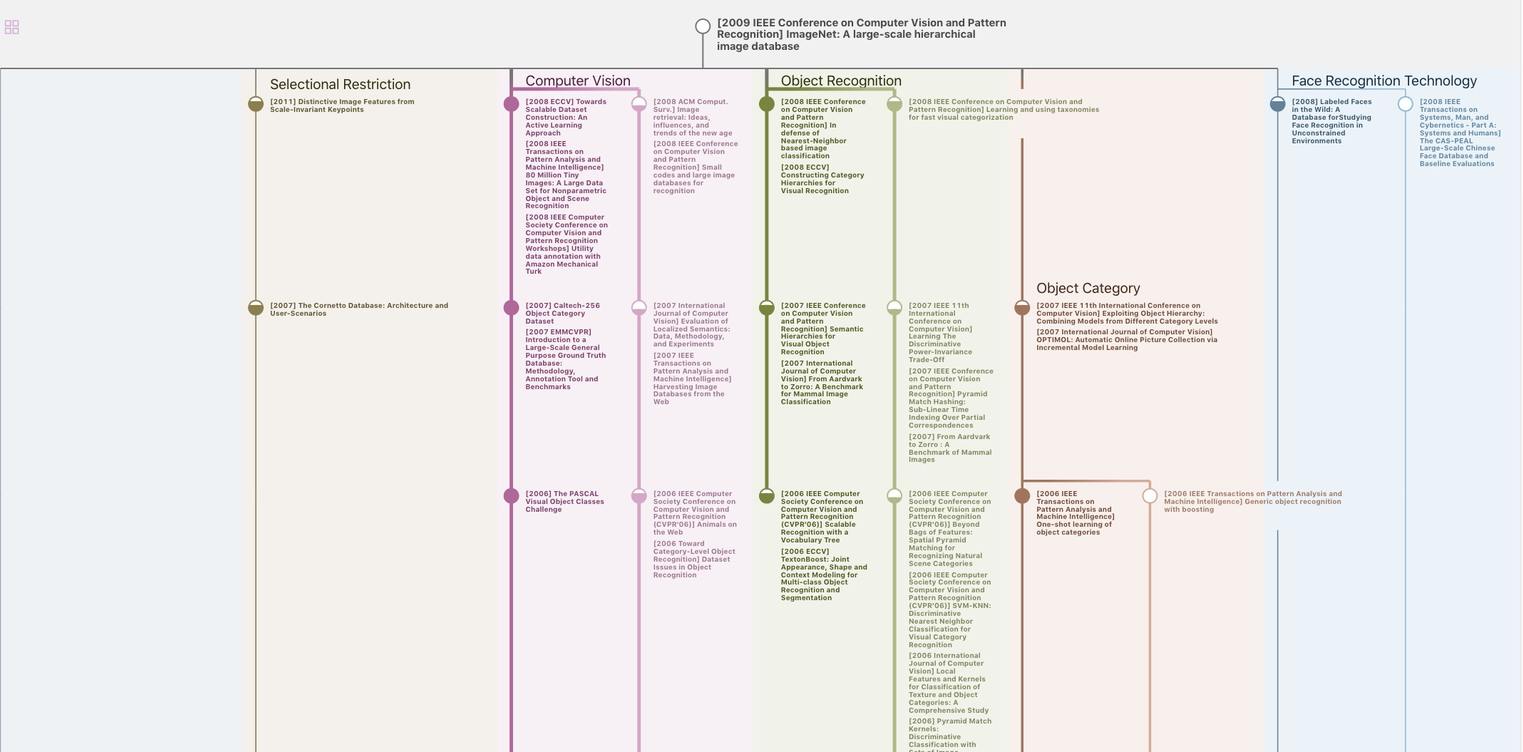
生成溯源树,研究论文发展脉络
Chat Paper
正在生成论文摘要