Real Estate Price Prediction on GenerativeLanguage Models
2023 IEEE Asia-Pacific Conference on Computer Science and Data Engineering (CSDE)(2023)
摘要
Real estate prediction is an important field of study that can assist home sellers and property investors in making informed decisions to maximize their profits. However, predicting real estate prices presents a significant challenge in the temporal dimension because house prices fluctuate over time in response to market dynamics and are influenced by a complex array of factors, such as location, quality of the house and so on. This paper focuses on leveraging the power of transformer-based language models and self-attentions for real estate house price prediction. The Transformer architecture is well-known for its ability to understand the relationships between words or tokens in a sequence of human language and process the entire sequence in parallel, enabling more efficient and scalable computations. Our study explores the fine-tuning of attention mechanisms and output hidden states from Transformer-based models, comparing their performance against baseline models. Through our experimentation and analysis, the results demonstrate that the transformer-based attention models outperform the baseline models for real estate price prediction. We also discovered that utilizing self-attentions from unsupervised text learning can enhance the accuracy of real estate price prediction.
更多查看译文
关键词
real estate,house price prediction,transformer attention,generative AI
AI 理解论文
溯源树
样例
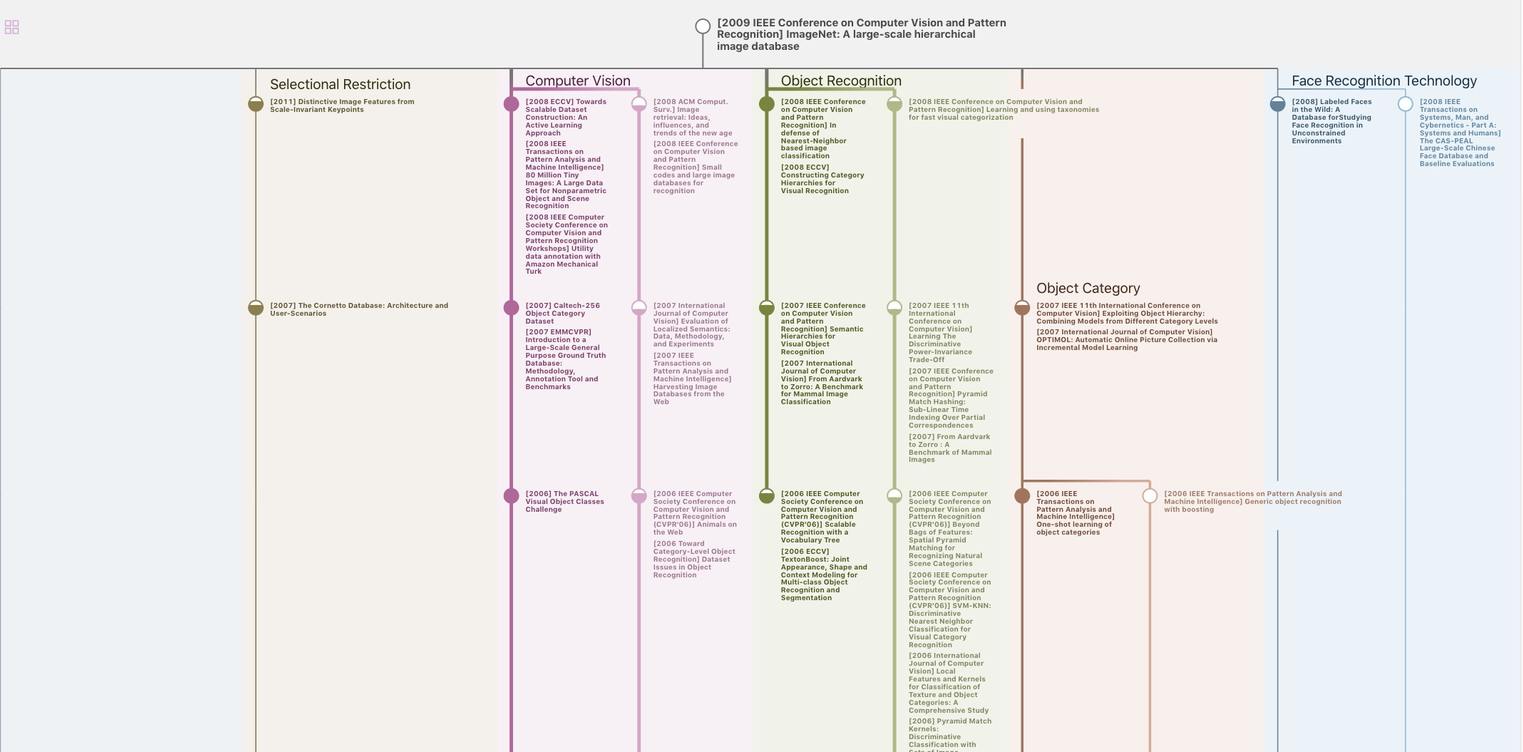
生成溯源树,研究论文发展脉络
Chat Paper
正在生成论文摘要