Machine Learning in Quasi-Newton Methods
AXIOMS(2024)
摘要
In this article, we consider the correction of metric matrices in quasi-Newton methods (QNM) from the perspective of machine learning theory. Based on training information for estimating the matrix of the second derivatives of a function, we formulate a quality functional and minimize it by using gradient machine learning algorithms. We demonstrate that this approach leads us to the well-known ways of updating metric matrices used in QNM. The learning algorithm for finding metric matrices performs minimization along a system of directions, the orthogonality of which determines the convergence rate of the learning process. The degree of learning vectors' orthogonality can be increased both by choosing a QNM and by using additional orthogonalization methods. It has been shown theoretically that the orthogonality degree of learning vectors in the Broyden-Fletcher-Goldfarb-Shanno (BFGS) method is higher than in the Davidon-Fletcher-Powell (DFP) method, which determines the advantage of the BFGS method. In our paper, we discuss some orthogonalization techniques. One of them is to include iterations with orthogonalization or an exact one-dimensional descent. As a result, it is theoretically possible to detect the cumulative effect of reducing the optimization space on quadratic functions. Another way to increase the orthogonality degree of learning vectors at the initial stages of the QNM is a special choice of initial metric matrices. Our computational experiments on problems with a high degree of conditionality have confirmed the stated theoretical assumptions.
更多查看译文
关键词
minimization algorithm,quasi-Newton method,convergence rate,machine learning
AI 理解论文
溯源树
样例
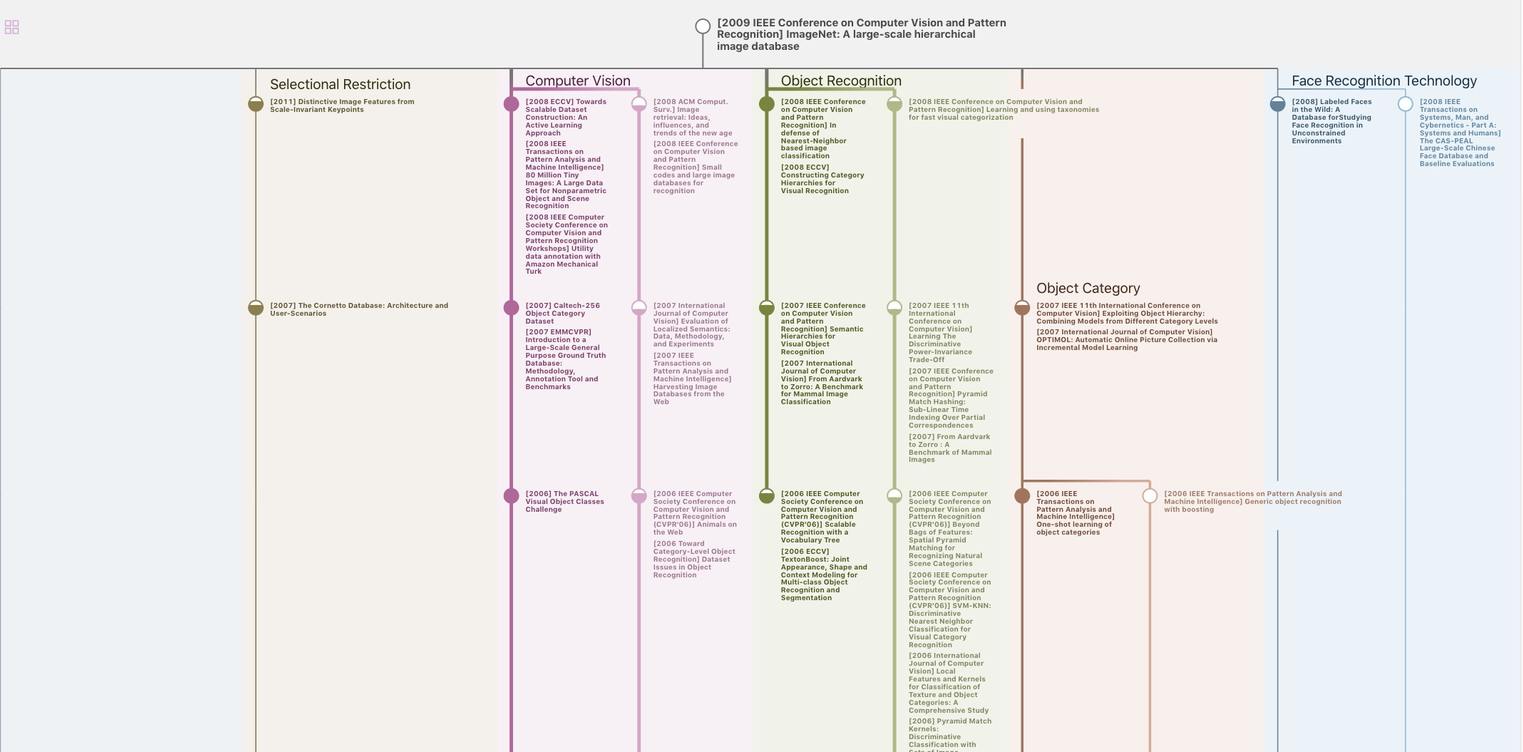
生成溯源树,研究论文发展脉络
Chat Paper
正在生成论文摘要