Understanding Patterns of Deep Learning Model Evolution in Network Architecture Search.
International Conference on High Performance Computing, Data, and Analytics(2023)
摘要
Network Architecture Search and specifically Regularized Evolution is a common way to refine the structure of a deep learning model. However, little is known about how models empirically evolve over time which has design implications for designing caching policies, refining the search algorithm for particular applications, and other important use cases. In this work, we algorithmically analyze and quantitatively characterize the patterns of model evolution for a set of models from the Candle project and the Nasbench-201 search space. We show how the evolution of the model structure is influenced by the regularized evolution algorithm. We describe how evolutionary patterns appear in distributed settings and opportunities for caching and improved scheduling. Lastly, we describe the conditions that affect when particular model architectures rise and fall in popularity based on their frequency of acting as a donor in a sliding window.
更多查看译文
关键词
Transfer Learning,AI,Network Architecture Search,Regularized Evolution,Characterization Study
AI 理解论文
溯源树
样例
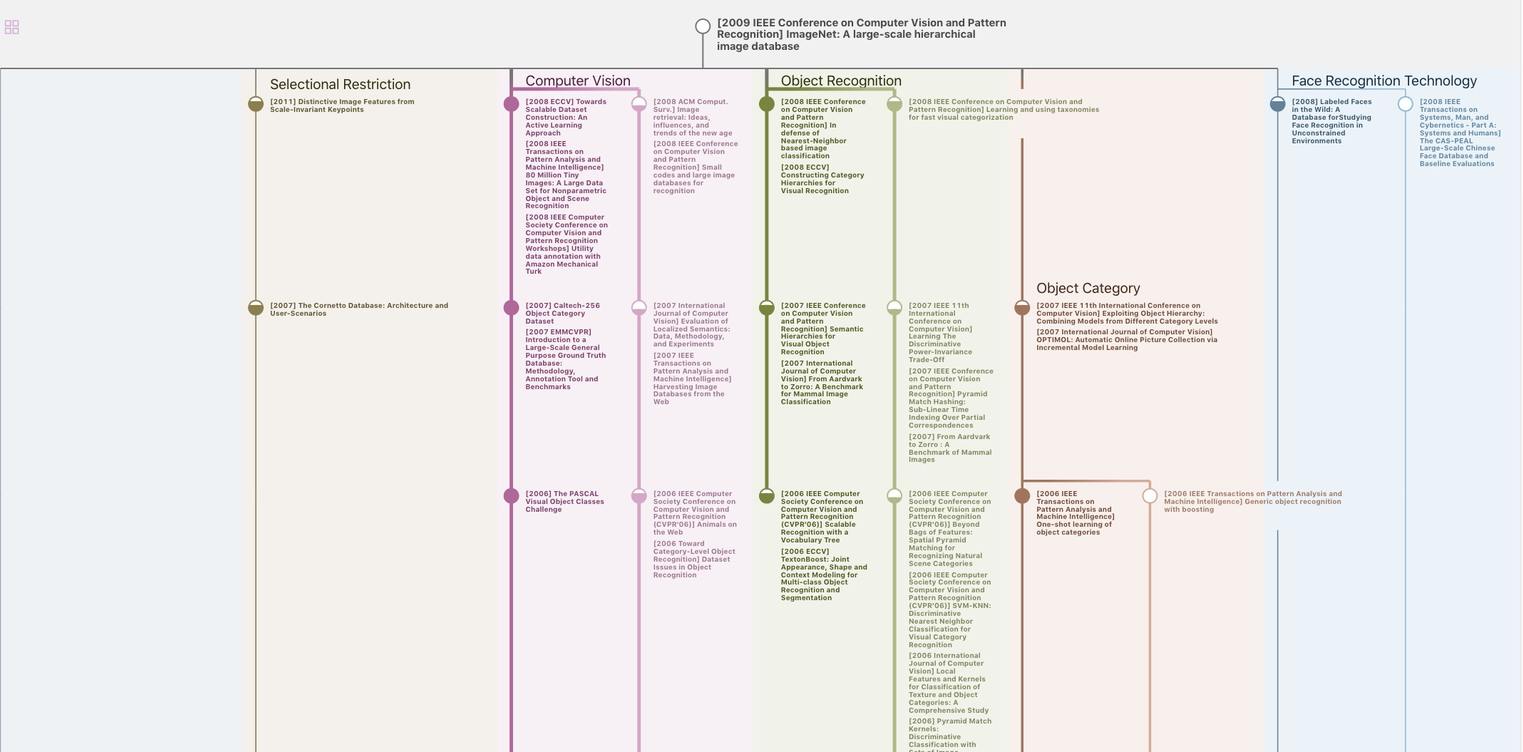
生成溯源树,研究论文发展脉络
Chat Paper
正在生成论文摘要