MADM: A Model-agnostic Denoising Module for Graph-based Social Recommendation
PROCEEDINGS OF THE 17TH ACM INTERNATIONAL CONFERENCE ON WEB SEARCH AND DATA MINING, WSDM 2024(2024)
摘要
Graph-based social recommendation improves the prediction accuracy of recommendation by leveraging high-order neighboring information contained in social relations. However, most of them ignore the problem that social relations can be noisy for recommendation. Several studies attempt to tackle this problem by performing social graph denoising, but they suffer from 1) adaptability issues for other graph-based social recommendation models and 2) insufficiency issues for user social representation learning. To address the limitations, we propose a model-agnostic graph denoising module (denoted as MADM) which works as a plug-and-play module to provide refined social structure for base models. Meanwhile, to propel user social representations to be minimal and sufficient for recommendation, MADM further employs mutual information maximization (MIM) between user social representations and the interaction graph and realizes two ways of MIM: contrastive learning and forward predictive learning. We provide theoretical insights and guarantees from the perspectives of Information Theory and Multi-view Learning to explain its rationality. Extensive experiments on three real-world datasets demonstrate the effectiveness of MADM. The codes are available here.
更多查看译文
关键词
Social Recommendation,Graph Denoising
AI 理解论文
溯源树
样例
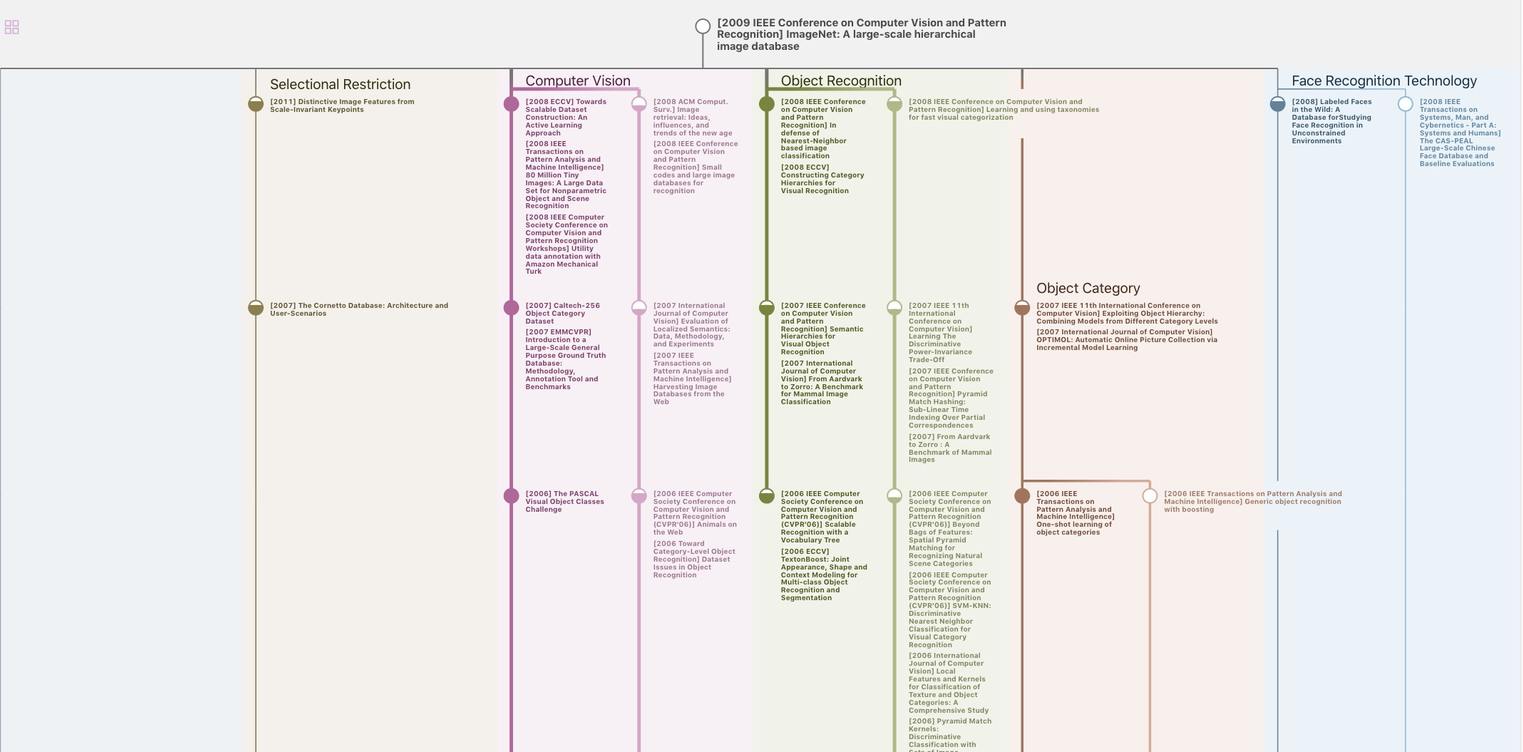
生成溯源树,研究论文发展脉络
Chat Paper
正在生成论文摘要