A Linguistic Grounding-Infused Contrastive Learning Approach for Health Mention Classification on Social Media
PROCEEDINGS OF THE 17TH ACM INTERNATIONAL CONFERENCE ON WEB SEARCH AND DATA MINING, WSDM 2024(2024)
摘要
Social media users use disease and symptoms words in different ways, including describing their personal health experiences figuratively or in other general discussions. The health mention classification (HMC) task aims to separate how people use terms, which is important in public health applications. Existing HMC studies address this problem using pretrained language models (PLMs). However, the remaining gaps in the area include the need for linguistic grounding, the requirement for large volumes of labelled data, and that solutions are often only tested on Twitter or Reddit, which provides limited evidence of the transportability of models. To address these gaps, we propose a novel method that uses a transformer-based PLM to obtain a contextual representation of target (disease or symptom) terms coupled with a contrastive loss to establish a larger gap between target terms' literal and figurative uses using linguistic theories. We introduce the use of a simple and effective approach for harvesting candidate instances from the broad corpus and generalising the proposed method using selftraining to address the label scarcity challenge. Our experiments on publicly available health-mention datasets from Twitter (HMC2019) and Reddit (RHMD) demonstrate that our method outperforms the state-of-the-art HMC methods on both datasets for the HMC task. We further analyse the transferability and generalisability of our method and conclude with a discussion on the empirical and ethical considerations of our study.
更多查看译文
关键词
Health Mention Classification,Public Health Surveillance,Contrastive Learning,Social Media
AI 理解论文
溯源树
样例
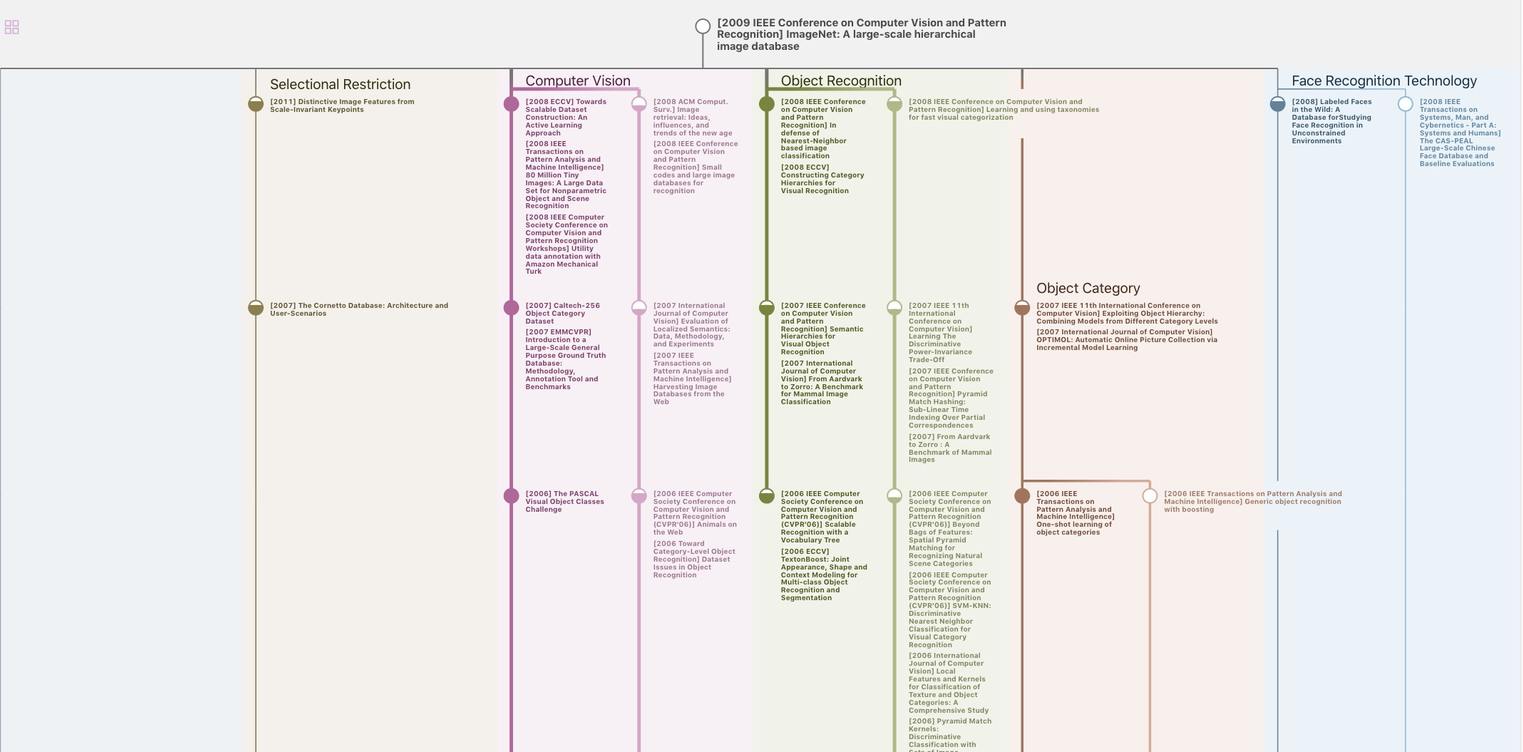
生成溯源树,研究论文发展脉络
Chat Paper
正在生成论文摘要