Semiparametric bivariate hierarchical state space model with application to hormone circadian relationship
ANNALS OF APPLIED STATISTICS(2024)
摘要
The adrenocorticotropic hormone and cortisol play critical roles in stress regulation and the sleep-wake cycle. Most research has been focused on how the two hormones regulate each other in terms of short-term pulses. Few studies have been conducted on the circadian relationship between the two hormones and how it differs between normal and abnormal groups. The circadian patterns are difficult to model as parametric functions. Directly extending univariate functional mixed effects models would result in a large dimensional problem and a challenging nonparametric inference. In this article we propose a semiparametric bivariate hierarchical state space model in which each hormone profile is modeled by a hierarchical state space model with nonparametric population-average and subject-specific components. The bivariate relationship is constructed by concatenating two latent independent subject-specific random functions specified by a design matrix, leading to a parametric inference on the correlation. We propose a computationally efficient state-space EM algorithm for estimation and inference. We apply the proposed method to a study of chronic fatigue syndrome and fibromyalgia and discover an erratic regulation pattern in the patient group in contrast to a circadian regulation pattern conforming to the day-night cycle in the control group.
更多查看译文
关键词
Circadian rhythm,functional mixed effects model,state space EM algorithm,time- varying correlation
AI 理解论文
溯源树
样例
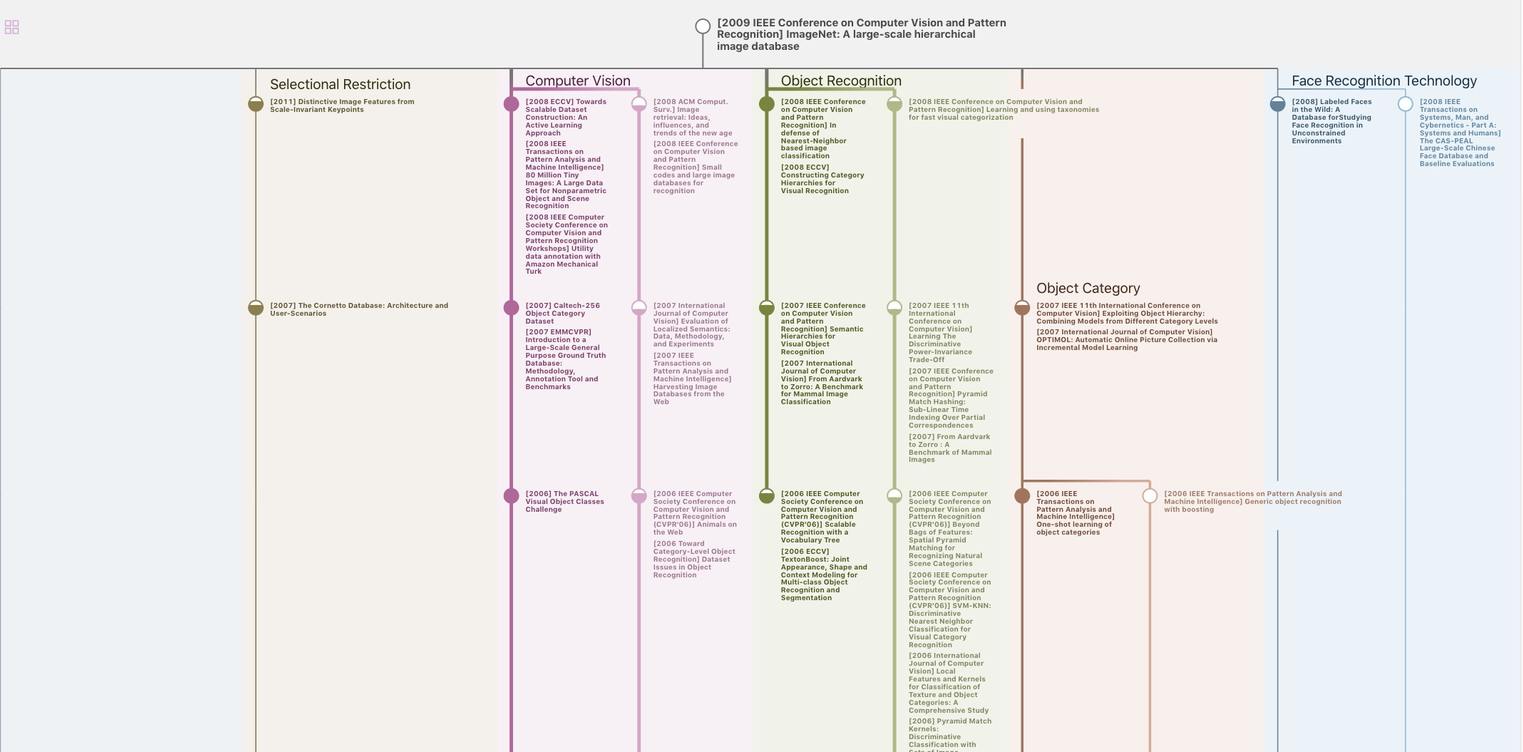
生成溯源树,研究论文发展脉络
Chat Paper
正在生成论文摘要