A Modulation Classification Algorithm Based on Feature-Embedding Graph Convolutional Network
IEEE Access(2024)
摘要
Deep-learning is widely used in modulation classification to reduce labor and improve the efficiency. Graph convolutional network (GCN) is a type of feature extraction network for graph data. Considering the signals as graph nodes and the similarity of each signal as an edge, the GCN propagates node information to similar nodes along the edges. GCN extracts more features and achieves better classification results, particularly for characterless examples. In this paper, we propose a modulation classification algorithm based on a feature-embedding GCN (FE-GCN). It comprises three parts: feature-embedding network (FEN), similarity adjacent matrix calculation network (SAMCN), and graph convolutional classification network (GCCN). The FEN embeds the signal data into a one-dimensional feature vector. The SAMCN calculates the similarity of all signal feature vectors to a matrix using a single convolutional neural network (CNN). The GCCN is used to extract the final features and classify the signals in a graph. Simulation results on the public dataset RML2016.10A show that the FE-GCN performs effectively and outperforms a series of advanced deep-learning methods.
更多查看译文
关键词
Feature Embedding,Modulation Recognition,GCN
AI 理解论文
溯源树
样例
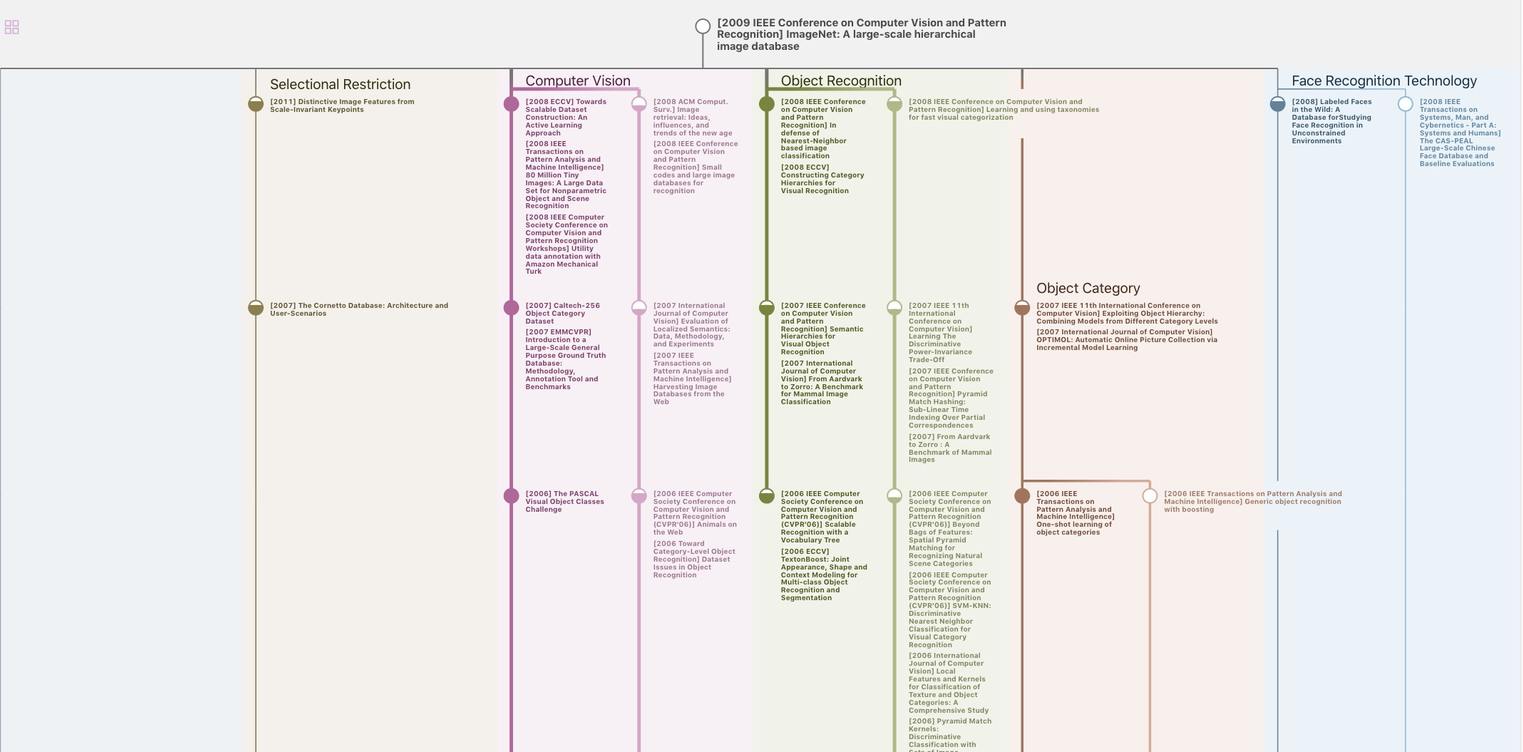
生成溯源树,研究论文发展脉络
Chat Paper
正在生成论文摘要