Investigating the potential of Machine Learning for fault location on Inverter-Based Resource interconnection lines: Insights and recommendations
Electric Power Systems Research(2024)
摘要
Given the increasing penetration of Inverter-Based Resources (IBR) and the impacts of these generators on existing fault location functions, this paper explores the potential of Machine Learning (ML) for designing one-terminal fault locators applied to systems with IBRs. For the studies, a system with a widely used topology for IBR interconnection to a transmission grid is modeled in PSCAD software, considering fault scenarios on the interconnection line with varying fault parameters, in addition to different IBR controls/topologies, grid short-circuit levels and signal noise levels. Besides the investigations on correctly choosing the attributes extracted from the signals for training ML methods, which introduces the concept of learning based on the existing method’s response, the studies cover performance assessments of 35 ML methods for the fault location task, indicating the most suitable ones, and studies about the influence of the training set size on the ML methods performance. Moreover, comparative performance analyses are conducted between the ML-based methodology and state-of-the-art fault locators, highlighting the benefits of the ML-based approach in reducing errors obtained in the fault location task. Finally, the paper recommends stages for applying ML-based methods to real systems, besides providing insights and recommendations on such practical applications.
更多查看译文
关键词
Fault diagnosis,Fault location,Inverter-Interfaced Resources,Machine-learning,Renewable energy
AI 理解论文
溯源树
样例
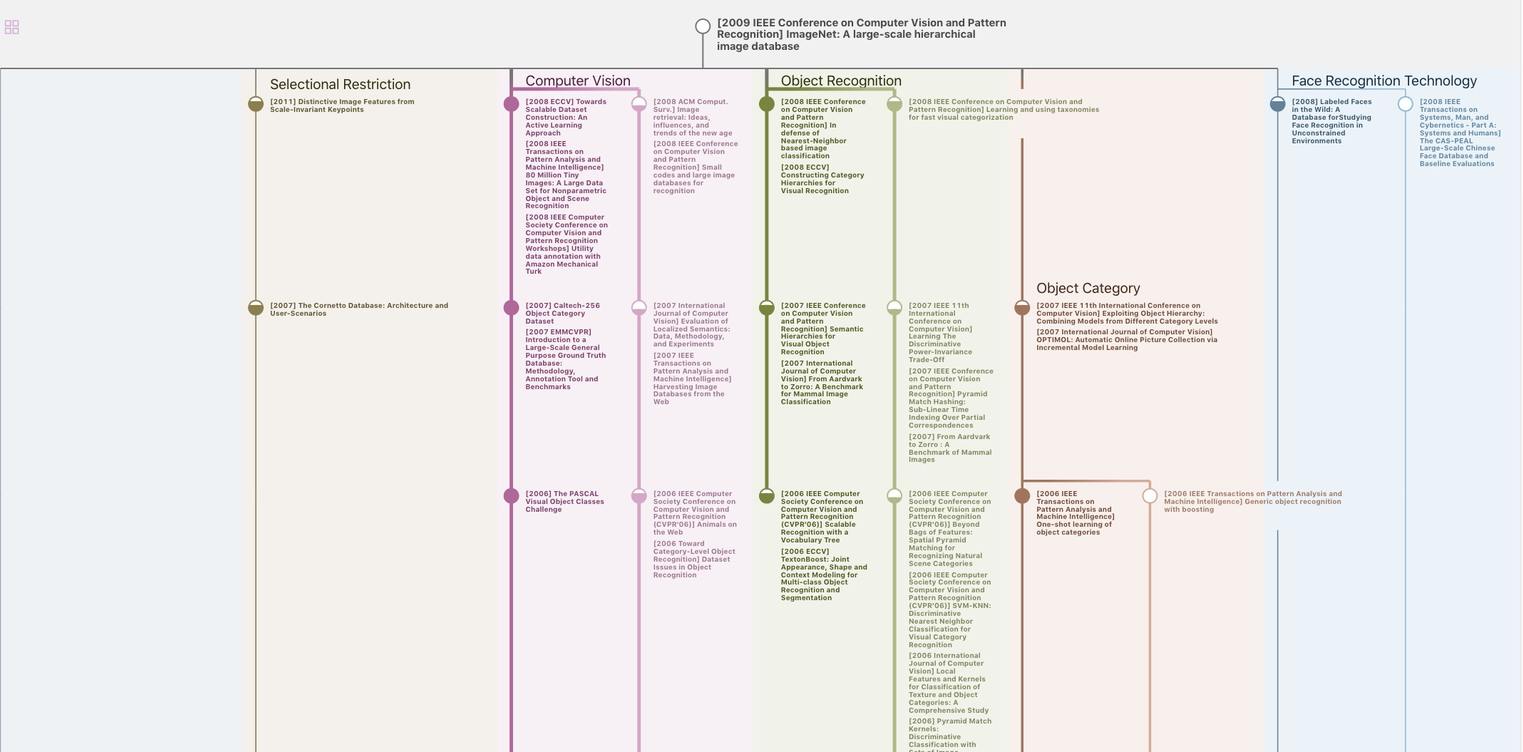
生成溯源树,研究论文发展脉络
Chat Paper
正在生成论文摘要