Pixel-associated autoencoder for hyperspectral anomaly detection
International Journal of Applied Earth Observation and Geoinformation(2024)
摘要
Autoencoders (AEs) are central to hyperspectral anomaly detection, given their impressive efficacy. However, the current methodologies often neglect the global pixel similarity of the hyperspectral image (HsI), thereby limiting reconstruction accuracy. This study introduces an innovative pixel-associated AE approach that leverages pixel associations to augment hyperspectral anomaly detection. First, a dictionary construction methodology was introduced based on superpixel distance estimation to construct distinct dictionaries for background and local anomalies. Second, to recognize pixel similarities, the similarity metric of each pixel from the original HsI to the background dictionary and to the local anomaly dictionary was employed as the AE network input in lieu of the original HsI. Third, a dual hidden-layer feature similarity constraint network was proposed to enhance the reconstruction error of background and anomaly targets. Finally, the reconstruction error was utilized to score the anomaly target. The proposed method was benchmarked against other state-of-the-art techniques using synthetic and real HsI datasets to assess its effectiveness. The experimental results demonstrated the superior performance of the proposed method, outperforming the alternatives.
更多查看译文
关键词
Anomaly detection,Autoencoder (AE),Hyperspectral image (HsI),Pixel similarity,Similarity metric
AI 理解论文
溯源树
样例
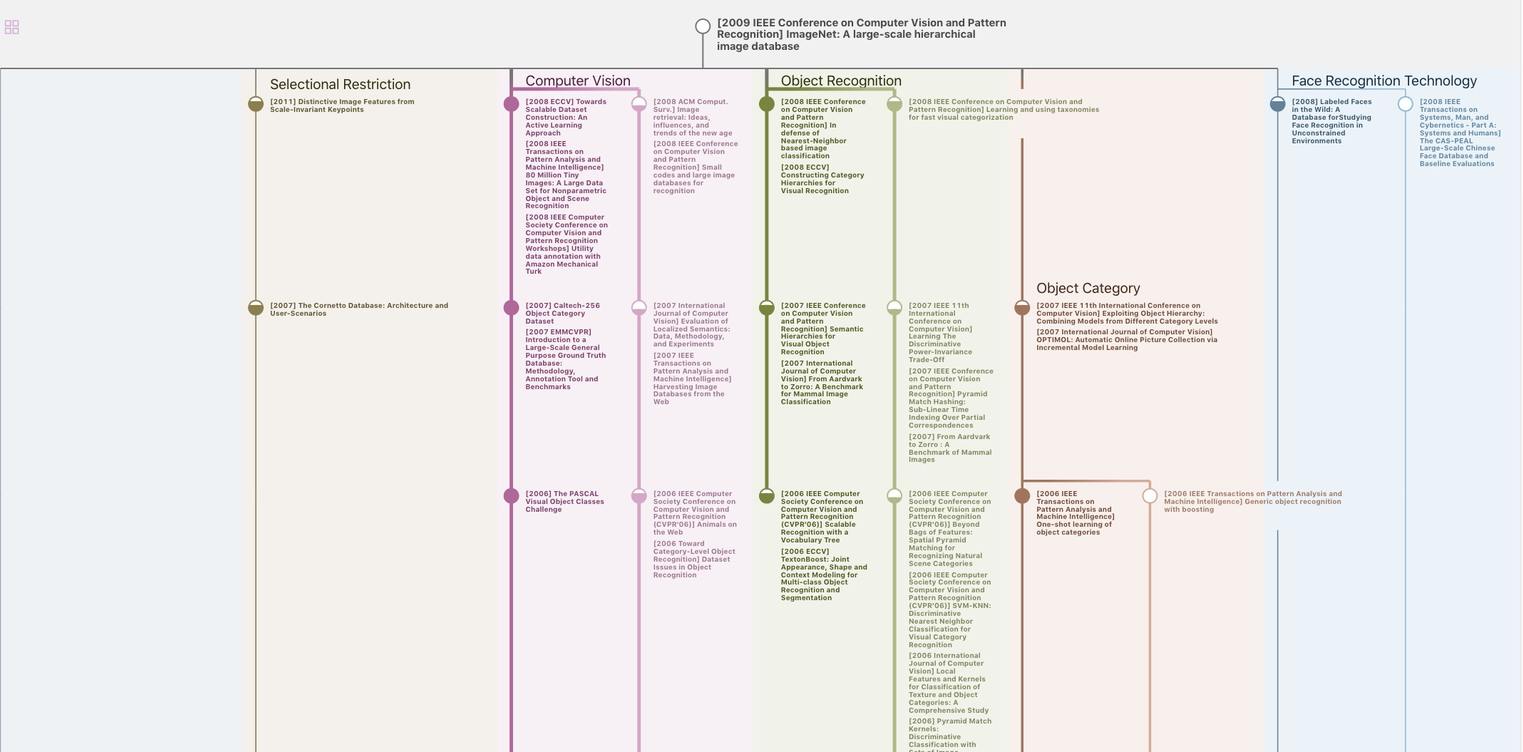
生成溯源树,研究论文发展脉络
Chat Paper
正在生成论文摘要