Performance prediction of VO2-based smart radiation devices through semi-self-supervised learning with phase transition adaptation
Next Energy(2024)
摘要
Accurately forecasting the infrared radiation properties of multilayer systems exhibiting phase transition behavior presents a formidable challenge. In this study, we propose a physically-inspired Phase Transition Adaptation Model (PTAM) that leverages a deep neural network with a branching architecture, coupled with an analytical optical solver. Given the inherent difficulty in accurately measuring film thickness and the inability to test optical constants in situ, we employ a semi-self-supervised learning strategy and train the model exclusively using experimental twin spectral data generated by VO2-based smart radiation devices (SRDs) during the thermal phase transition process. Our proposed model exhibits remarkable proficiency in capturing spatial distribution information pertaining to material characteristics in multilayer systems possessing thermochromic phenomena. Additionally, it demonstrates exceptional accuracy in predicting the radiation regulation performance of such systems. These advances have significant implications for the cost-effective and efficient development of SRDs. In line with the pressing need to combat climate change and promote sustainable energy practices, this research makes a vital contribution to the quest for a more sustainable future.
更多查看译文
关键词
Neural network,Deep learning,Radiation regulation,Vanadium dioxide
AI 理解论文
溯源树
样例
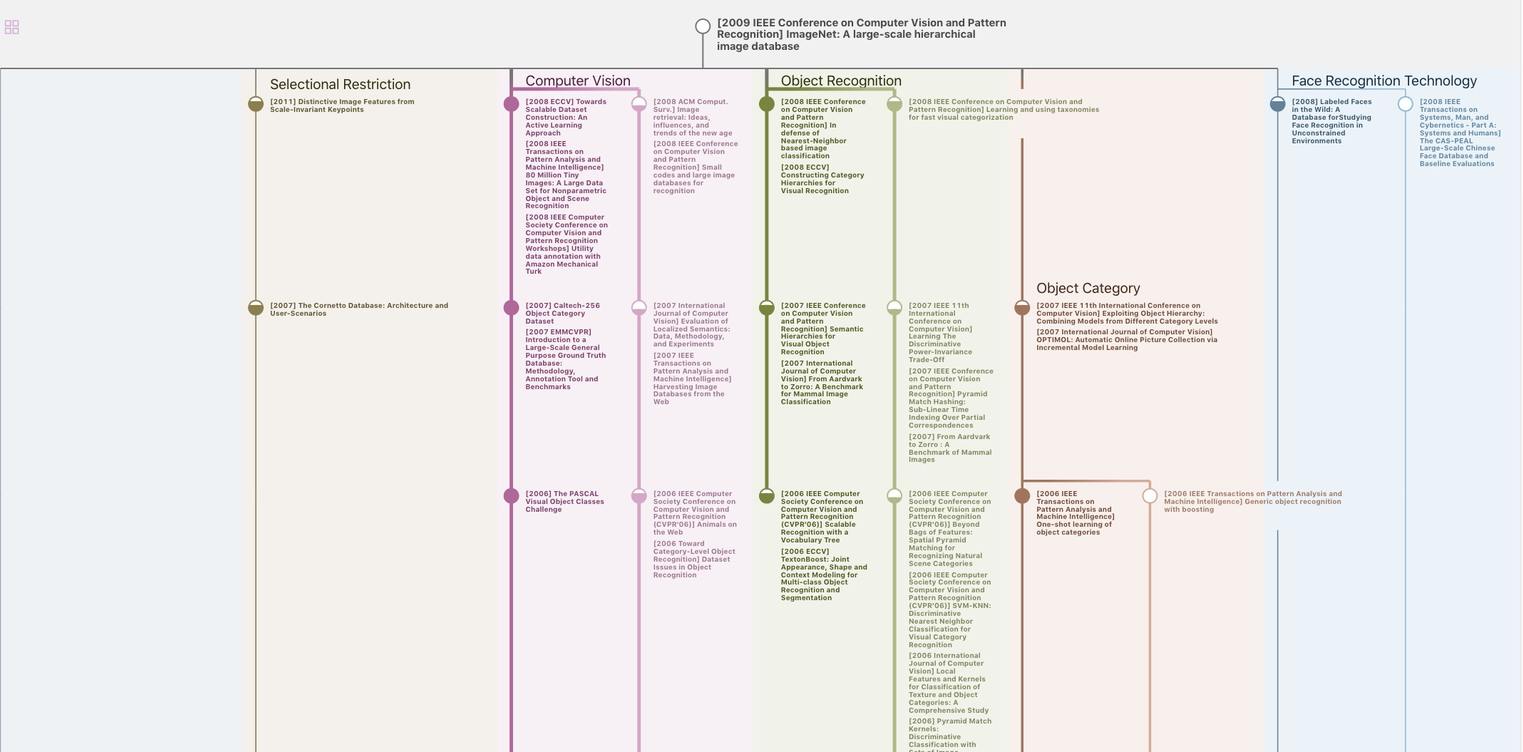
生成溯源树,研究论文发展脉络
Chat Paper
正在生成论文摘要