Advanced machine learning-powered tunable optical signal processor for precise chromatic dispersion compensation in analog B5G/6G mobile fronthaul networks
Machine Learning in Photonics(2024)
AI 理解论文
溯源树
样例
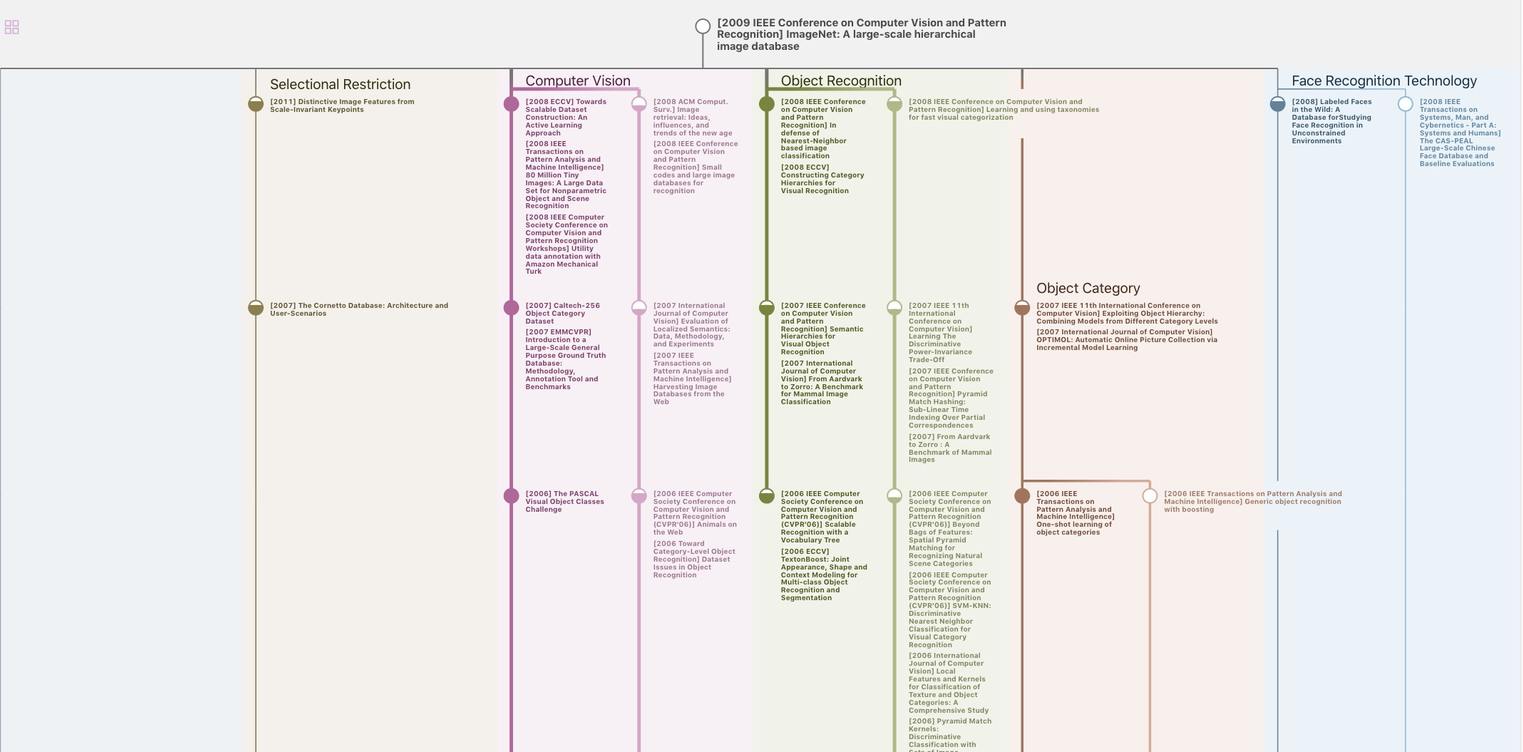
生成溯源树,研究论文发展脉络
Chat Paper
正在生成论文摘要
Machine Learning in Photonics(2024)