Randomized multiarm bandits: An improved adaptive data collection method
STATISTICAL ANALYSIS AND DATA MINING(2024)
摘要
In many scientific experiments, multiarmed bandits are used as an adaptive data collection method. However, this adaptive process can lead to a dependence that renders many commonly used statistical inference methods invalid. An example of this is the sample mean, which is a natural estimator of the mean parameter but can be biased. This can cause test statistics based on this estimator to have an inflated type I error rate, and the resulting confidence intervals may have significantly lower coverage probabilities than their nominal values. To address this issue, we propose an alternative approach called randomized multiarm bandits (rMAB). This combines a randomization step with a chosen MAB algorithm, and by selecting the randomization probability appropriately, optimal regret can be achieved asymptotically. Numerical evidence shows that the bias of the sample mean based on the rMAB is much smaller than that of other methods. The test statistic and confidence interval produced by this method also perform much better than its competitors.
更多查看译文
关键词
biased estimator,multiarmed bandits,optimal regret,statistical inference,type I error
AI 理解论文
溯源树
样例
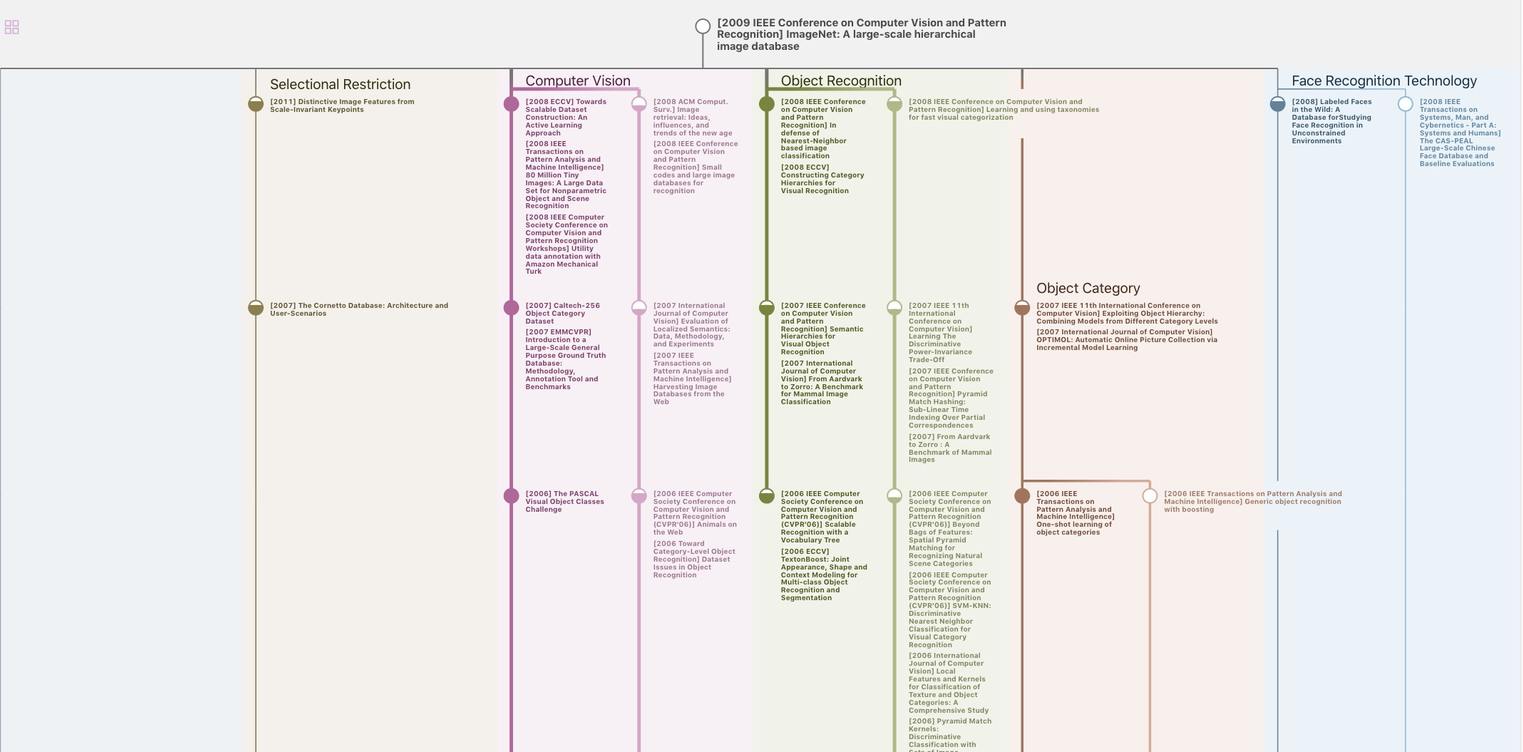
生成溯源树,研究论文发展脉络
Chat Paper
正在生成论文摘要