Flexible-dimensional L-statistic for Mean Estimation of Symmetric Distributions
Statistical papers(2024)
摘要
Estimating the mean of a population is a recurrent topic in statistics because of its multiple applications. If previous data is available, or the distribution of the deviation between the measurements and the mean is known, it is possible to perform such estimation by using L-statistics, whose optimal linear coefficients, typically referred to as weights, are derived from a minimization of the mean squared error. However, such optimal weights can only manage sample sizes equal to the one used to derive them, while in real-world scenarios this size might slightly change. Therefore, this paper proposes a method to overcome such a limitation and derive approximations of flexible-dimensional optimal weights. To do so, a parametric family of functions based on extreme value reductions and amplifications is proposed to be adjusted to the cumulative optimal weights of a given sample from a symmetric distribution. Then, the application of Yager’s method to derive weights for ordered weighted average (OWA) operators allows computing the approximate optimal weights for sample sizes close to the original one. This method is justified from the theoretical point of view by proving a convergence result regarding the cumulative weights obtained for different sample sizes. Finally, the practical performance of the theoretical results is shown for several classical symmetric distributions.
更多查看译文
关键词
L-statistics,Flexible sample size,Extreme values reductions,Extreme values amplifications,Mean estimation,62F35
AI 理解论文
溯源树
样例
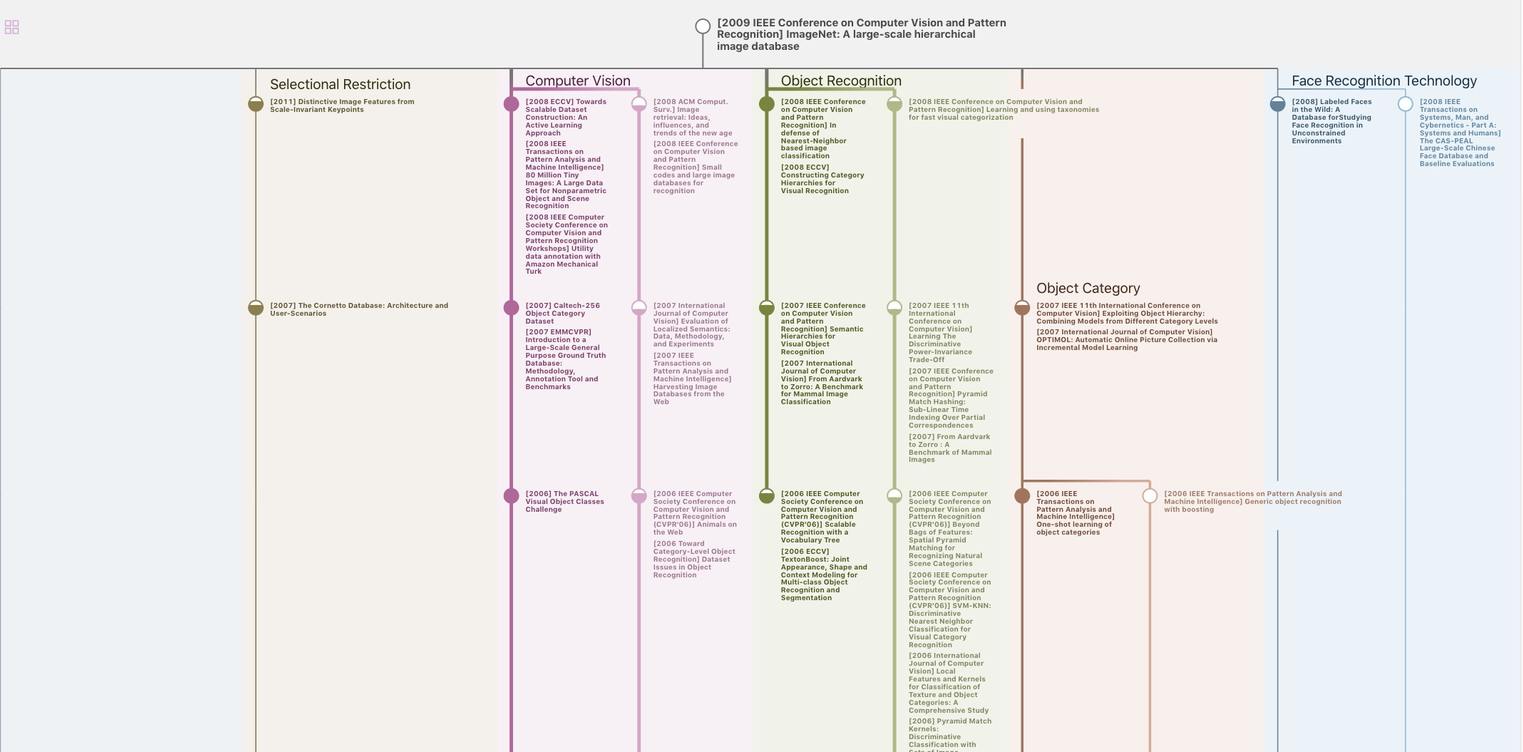
生成溯源树,研究论文发展脉络
Chat Paper
正在生成论文摘要