Mapping Global Lithospheric Mantle Pressure-Temperature Conditions by Machine-Learning Thermobarometry
GEOPHYSICAL RESEARCH LETTERS(2024)
摘要
Comprehending the temperature distribution within Earth's lithospheric mantle is of paramount importance for understanding the dynamics of Earth's interior. Traditional mineral-based thermobarometers effectively constrain temperature and pressure for particular compositions, but their application is limited at the global scale. Here, we trained machine-learning (ML) algorithms on 985 published high-temperature and high-pressure experiments for use as thermometers and barometers to overcome the limitations of classic methods. We compared our ML models to classic thermobarometers to assess the accuracies of predicted pressures and temperatures. The comparison shows that the ML models outperform classic methods and better fit various mineral pairs. Global application of the ML models unveils mantle conditions beneath cratons. Furthermore, depths to the lithosphere-asthenosphere boundary (LAB) calibrated based on the ML thermobarometry results are generally deeper by similar to 40 km than those derived geophysically, implying the existence of melt-bearing or hydrated mineral zones at the LAB. The temperature of the lithospheric mantle is pivotal to processes such as the production of magmas and the structural stability of cratons. Researchers have traditionally employed mineral-based thermobarometers to lithospheric mantle temperatures, but those conventional techniques have limited applications and are susceptible to inaccuracies. Here, we explored a new approach using machine learning, a powerful tool for identifying complex patterns in high-dimensional data space. We trained machine-learning models using data from high-pressure and high-temperature experiments, then compared our machine-learning models to traditional methods. Our results show that the machine-learning models better predict pressure and temperature for specific mineral combinations. We also used our optimized model to predict mantle temperatures and pressures based on a global data set of xenolith analyses, revealing insights about the temperature of the lithospheric mantle and the depth to the lithosphere-asthenosphere boundary beneath continents. This research shows that machine learning can greatly improve our understanding of Earth's deep processes, providing more accurate insights into its dynamics and evolution. Thermometers and barometers calibrated and validated for xenolithic mantle minerals by machine learning Global lithospheric mantle temperatures predicted based on xenolithic mineral compositions calculated by machine-learning thermobarometry Thermal continental lithosphere-asthenosphere boundarys refined by machine-learning thermobarometry are consistently similar to 40 km deeper than those determined geophysically
更多查看译文
关键词
machine learning,thermobarometry,lithospheric mantle,lithosphere-asthenosphere boundary,craton destruction
AI 理解论文
溯源树
样例
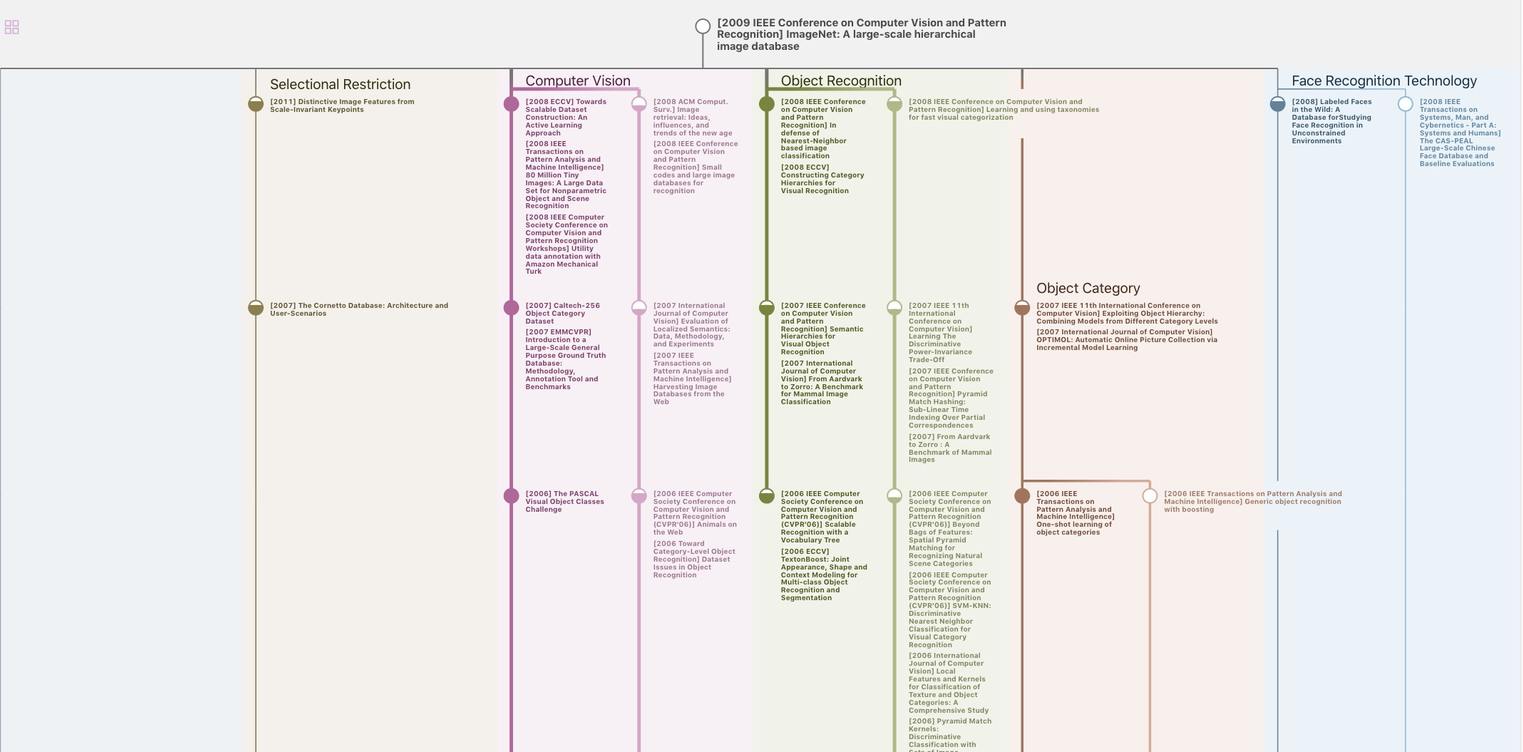
生成溯源树,研究论文发展脉络
Chat Paper
正在生成论文摘要