Multi-source precipitation estimation using machine learning: Clarification and benchmarking
Journal of Hydrology(2024)
摘要
Multi-source precipitation estimation techniques, including interpolation, bias correction, and merging, are essential for acquiring high-precision precipitation datasets. Machine learning (ML) methods have achieved inspiring success in this field, but current studies utilizing ML often blur the boundaries of interpolation, bias correction, and merging because of the utilization of uniform input–output frameworks. The ambiguity in classification (interpolation, bias correction and merging) results in inconsistent and inappropriate comparative benchmarking among studies. A widespread example is the tendency to select easy-to-beat benchmarks, which can magnify the relative performance of ML methods. We propose to reclarify ML-based precipitation estimation by conducting a series of experiments with designed feature combinations to unveil the essence of ML-based methods. Our findings suggest that with different feature combinations, there are no essential distinctions between models for merging, bias correction and interpolation. Ground measurements, as the reference in supervised learning, play a more vital role than different features in precipitation estimation. This means that the ML-based multi-source merging or bias correction should be treated as gauge data-based interpolation with various auxiliary predictors. Moreover, the improvements of ML estimates are more significant compared to low-standard benchmarks like individual precipitation datasets and simple merging methods, but less preeminent compared to high-standard benchmarks such as a sophisticated interpolation method (e.g., Geographically Weighted Regression). Therefore, we call for positioning such ML applications as interpolation and adhering to high-standard benchmarks whenever possible, which can illustrate the true potential and promote further advancements of ML in geospatial estimation.
更多查看译文
AI 理解论文
溯源树
样例
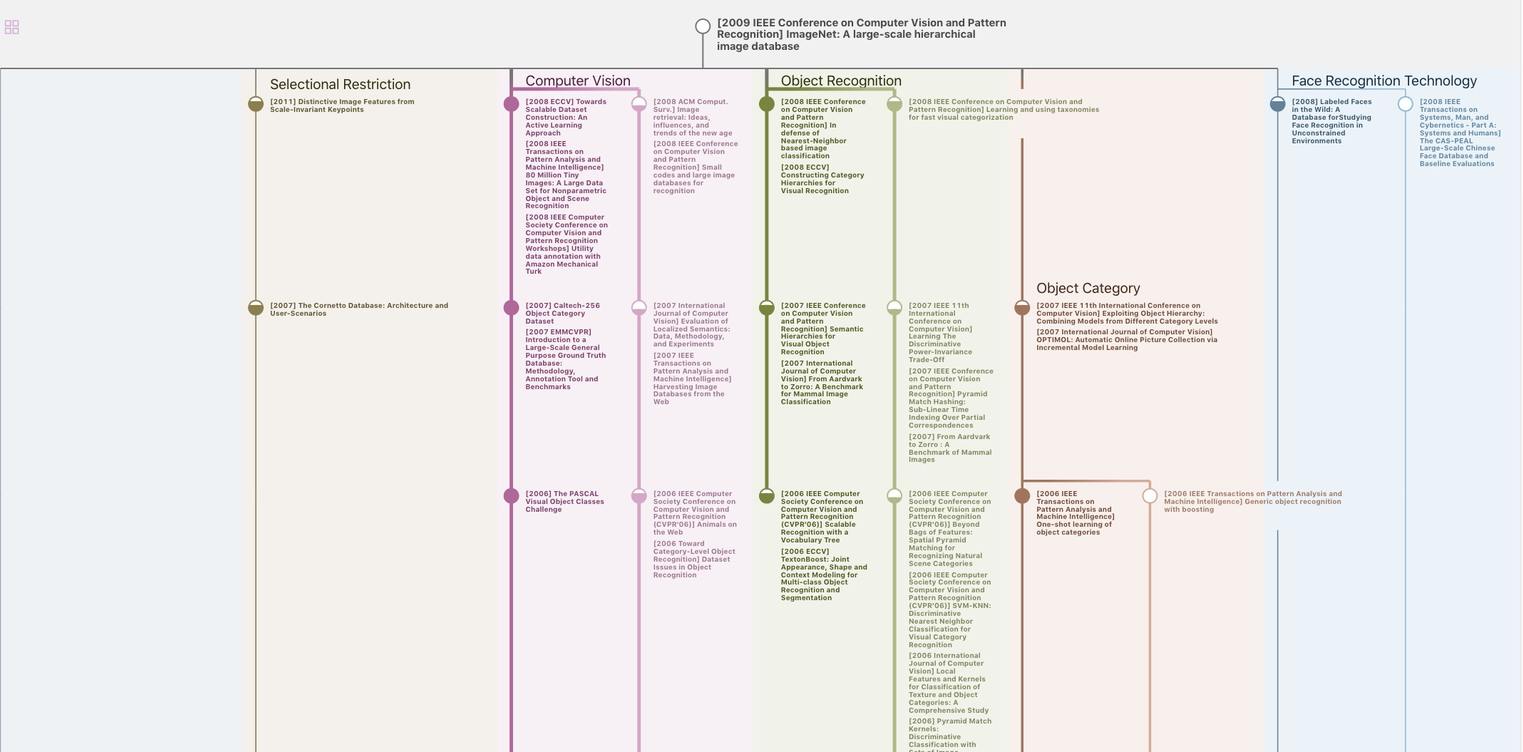
生成溯源树,研究论文发展脉络
Chat Paper
正在生成论文摘要