A lightweight algorithm for small traffic sign detection based on improved YOLOv5s
Signal, Image and Video Processing(2024)
摘要
With the rise of deep learning technology, significant progress has been made in object detection. Traffic sign detection is a research hotspot for object detection tasks. However, due to small size of traffic signs, there is room for further improvement in the comprehensive performance of the existing technology. In this paper, we propose a lightweight network based on yolov5s to achieve real-time localization and classification of small traffic signs. First, we improve the bottleneck transformers with 3 convolution (Bot3) module to enhance the backbone network’s ability to extract features from small targets, improving the accuracy while reducing the number of parameters and giga floating-point operations per second (GFLOPs). Second, we introduce ghost convolution (GhostConv) to obtain redundant feature maps with cheap operations to further improve the model’s efficiency. Finally, we use soft non-maximum suppression (Soft-NMS) in the detection phase to improve the model accuracy again without additional computational overhead for training. According to the tests on the Tsinghua-Tencent 100 K (TT100K) dataset, the proposed method outperforms the original YOLOv5s in small traffic sign detection, with an increase of 8.7 mAP_50 , a reduction of 22.5
更多查看译文
关键词
Traffic sign detection,Small object,Lightweight network,YOLOv5s
AI 理解论文
溯源树
样例
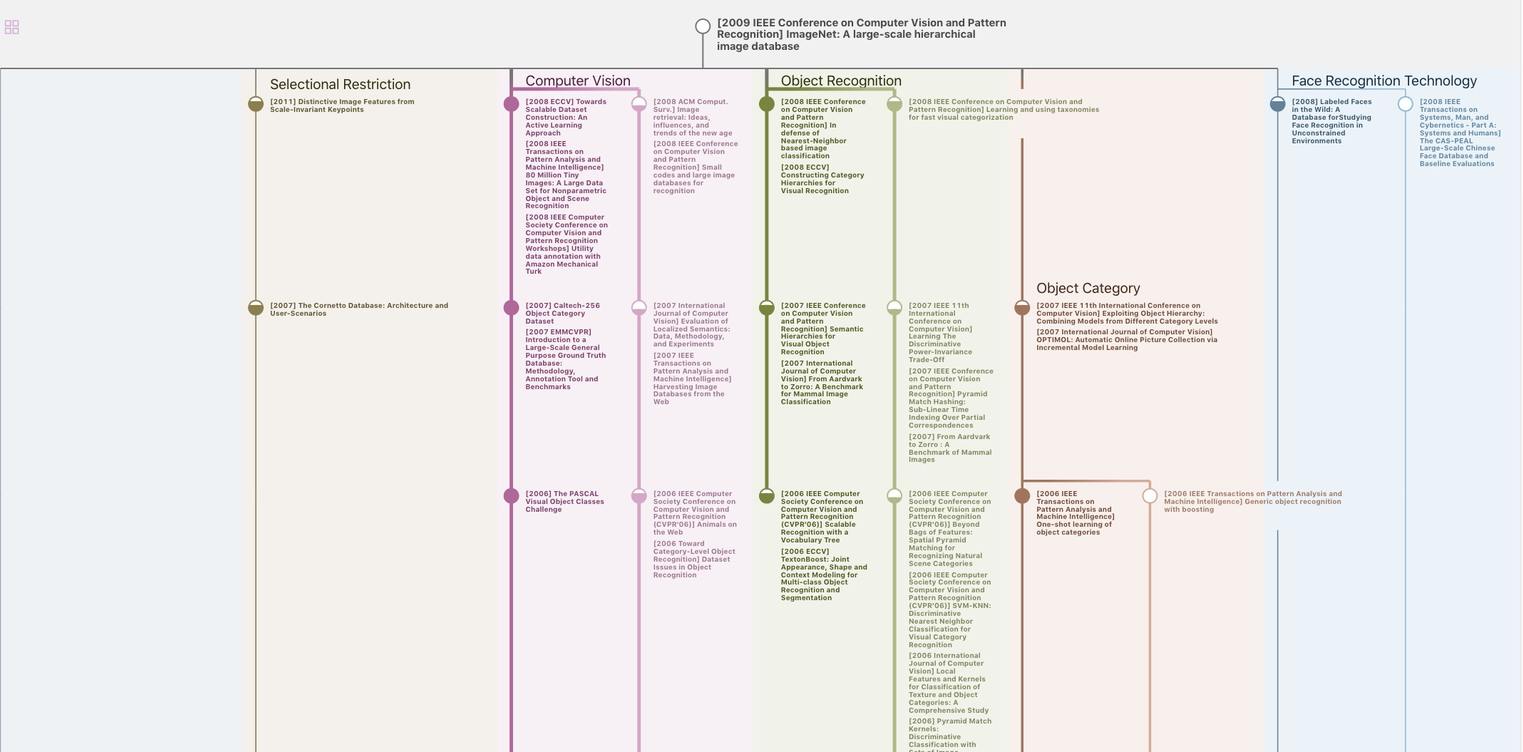
生成溯源树,研究论文发展脉络
Chat Paper
正在生成论文摘要