Hybrid Black-Box Classification for Customer Churn Prediction with Segmented Interpretability Analysis
DECISION SUPPORT SYSTEMS(2024)
摘要
Customer retention management relies on advanced analytics for decision making. Decision makers in this area require methods that are capable of accurately predicting which customers are likely to churn and that allow to discover drivers of customer churn. As a result, customer churn prediction models are frequently evaluated based on both their predictive performance and their capacity to extract meaningful insights from the models. In this paper, we extend hybrid segmented models for customer churn prediction by incorporating powerful models that can capture non-linearities. To ensure the interpretability of such segmented hybrid models, we introduce a novel model-agnostic approach that extends SHAP. We extensively benchmark the proposed methods on 14 customer churn datasets on their predictive performance. The interpretability aspect of the new model-agnostic approach for interpreting hybrid segmented models is illustrated using a case study. Our contributions to decision making literature are threefold. First, we introduce new hybrid segmented models as powerful tools for decision makers to boost predictive performance. Second, we provide insights in the relative predictive performance by an extensive benchmarking study that compares the new hybrid segmented methods with their base models and existing hybrid models. Third, we propose a model-agnostic tool for segmented hybrid models that provide decision makers with a tool to gain insights for any hybrid segmented model and illustrate it on a case study. Although we focus on customer retention management in this study, this paper is also relevant for decision makers that rely on predictive modeling for other tasks.
更多查看译文
关键词
XAI,Customer retention,Hybrid algorithm,Explainable analytics
AI 理解论文
溯源树
样例
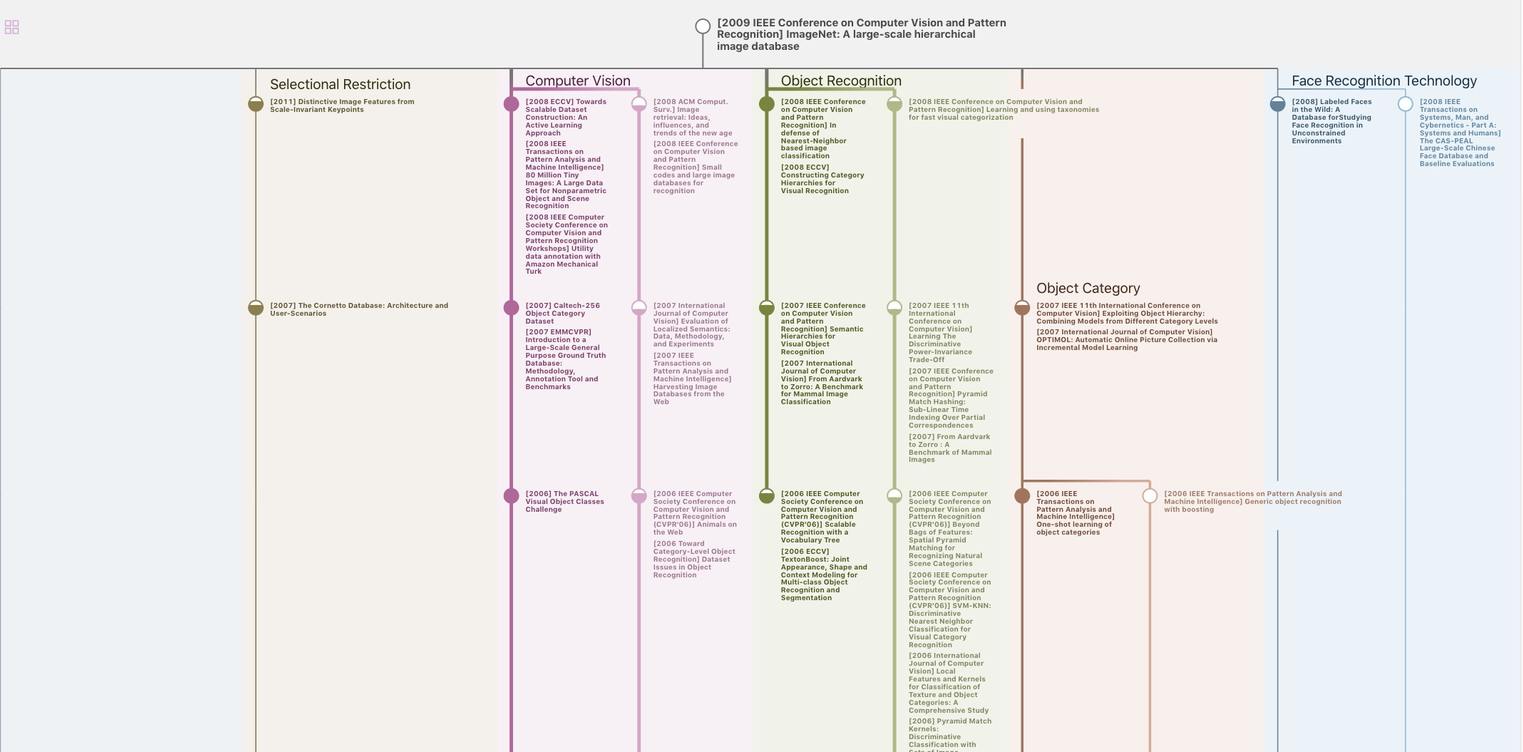
生成溯源树,研究论文发展脉络
Chat Paper
正在生成论文摘要