AugLPN-NILM: Augmented Lightweight Parallel Network for NILM Embedding Attention Module over Sequence to Point
SUSTAINABLE ENERGY GRIDS & NETWORKS(2024)
摘要
Non-Intrusive Load Monitoring (NILM), also called energy disaggregation, is a feasible strategy for identifying appliance-by-appliance power consumption given the aggregated power demand of electrical loads. A non-intrusive sequence to point load disaggregation parallel model (AugLPN-NILM) is proposed in this paper. Spatial-temporal feature fusion is introduced in our AugLPN to offer more differentiated features, which alleviates the weakness of time domain feature loss, therefore, can boost the performance of energy disaggregation. Most current methods have large performance variations of predicting power consumption for different appliances, the parallel architecture in the AugLPN not only greatly reduces the variations with a relatively small number of parameters to tune, but also accurately predicts the on/off state of appliances across unseen houses or buildings. Furthermore, we present an enhanced attention module, which is applied to activate or restrain features adaptively and especially improve decomposition accuracy of type II appliances. In addition, an effective postprocessing algorithm is proposed to further optimize the disaggregation results. The experiments executed on two publicly accessible datasets, UK-DALE and REDD, demonstrate our proposed AugLPN model’s superiority compared with some existing algorithms in all the identical experimental conditions. The exact code for reproducing the results in this paper is available at https://github.com/linfengYang/AugLPN_NILM.
更多查看译文
关键词
Non -intrusive load monitoring (NILM),Load disaggregation,Sequence to point,Lightweight network,Enhanced attention module
AI 理解论文
溯源树
样例
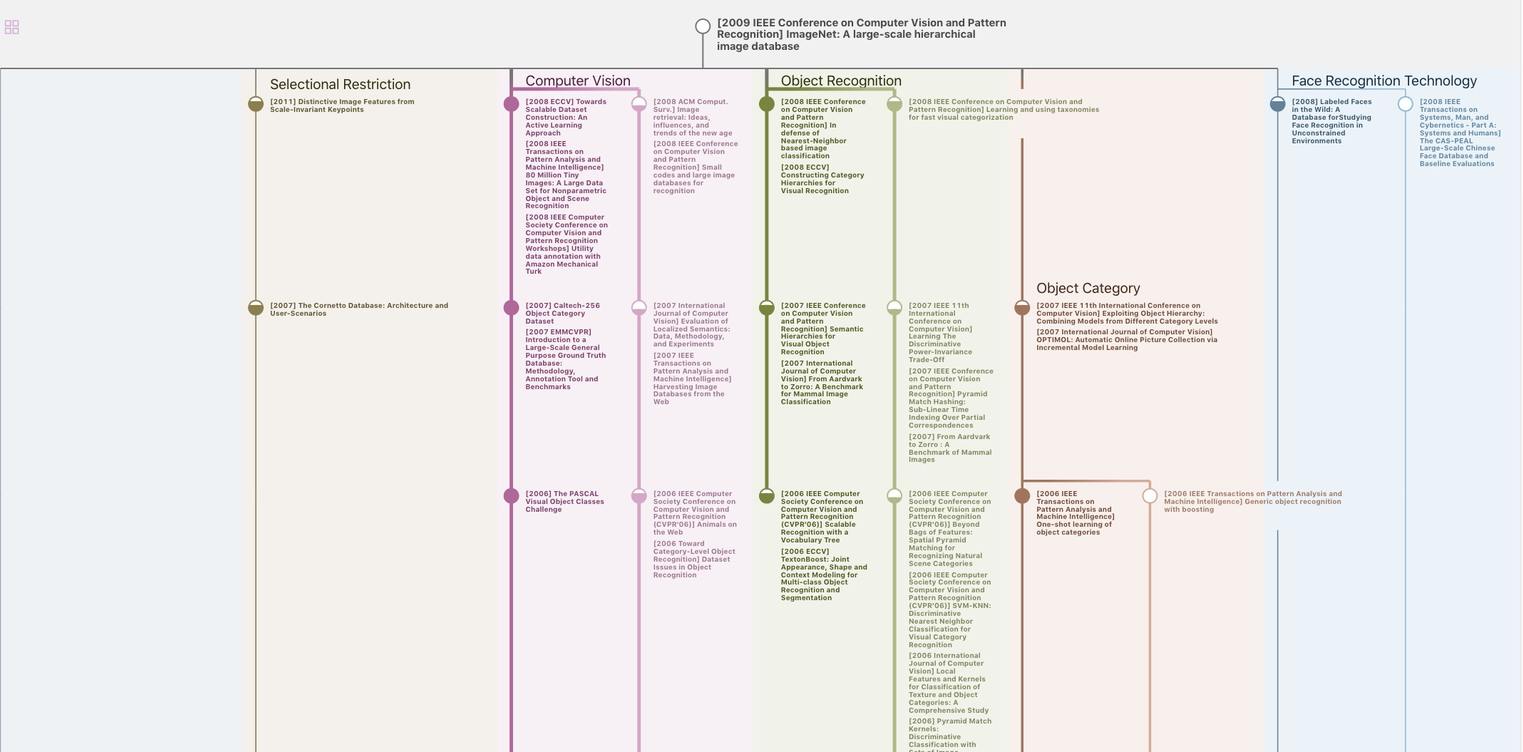
生成溯源树,研究论文发展脉络
Chat Paper
正在生成论文摘要