Device parameter prediction for GAA junctionless nanowire FET using ANN approach
Microelectronics Journal(2024)
摘要
The primary objective of this study is to investigate the potential of artificial neural network (ANN) for predicting the short-channel effect parameters and current-voltage curve in gate-all-around junctionless nanowire FET (GAA-JL-NWFET). The evaluation of the effectiveness of an ANN is typically done by analyzing metrics like root mean square error (RMSE) and coefficient of determination (R2-score). This study comprises various visualizations such as plots depicting the training loss per epoch against the epoch, scatter plots that compare actual values with predicted values and density plots illustrating the relationship between the density of data points and errors. The ability of the ANN model to accurately predict the important device parameters indicates its effectiveness. The parameters include drain current (IDS), drain induced barrier lowering (DIBL), threshold voltage (Vt), subthreshold swing (SS), on-current (ION) and off-current (IOFF). The root mean square error (RMSE) values corresponding to IDS, DIBL, Vt, SS, ION and IOFF were found to be 5.92 %, 0.79 %, 1.50 %, 1.31 %, 4.35 % and 9.81 % respectively. Furthermore, it is worth to mention that the R2-scores associated with these characteristics exhibit a very high level of accuracy as can be seen by the values of 0.9835, 0.9156, 0.9919, 0.9644, 0.9833 and 0.9097 for IDS, DIBL, Vt, SS, ION and IOFF respectively. The approach of using ANN model matches the accuracy of physics-based technology computer aided design (TCAD) solvers and its precision makes it an excellent alternative. In summary, this work demonstrates the potential of ANN as a robust tool for accurate prediction of device performance specifically in the field of semiconductor devices.
更多查看译文
关键词
Junctionless,Nanowire,GAA,ANN,Short channel effects
AI 理解论文
溯源树
样例
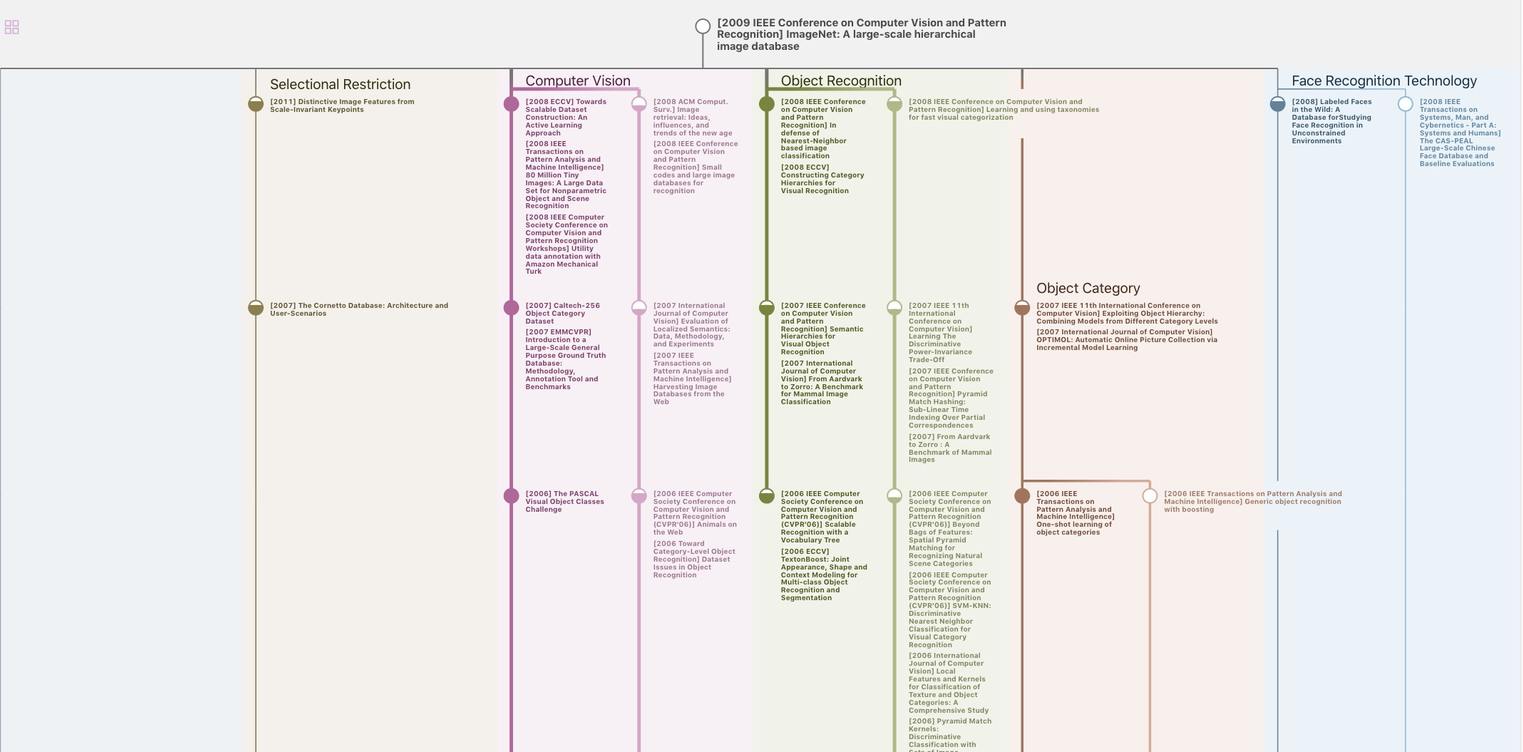
生成溯源树,研究论文发展脉络
Chat Paper
正在生成论文摘要